Prof. Dr. Cyrill Stachniss
Head Contact:Email: cyrill.stachniss@nulligg.uni-bonn.de
Tel: +49 – 228 – 73 – 27 13 (secretary)
Tel: +49 – 228 – 73 – 27 14 (direct)
Fax: +49 – 228 – 73 – 27 12
Office: Nussallee 15, 1. OG, room 1.009
Address:
University of Bonn
Photogrammetry, IGG
Nussallee 15
53115 Bonn
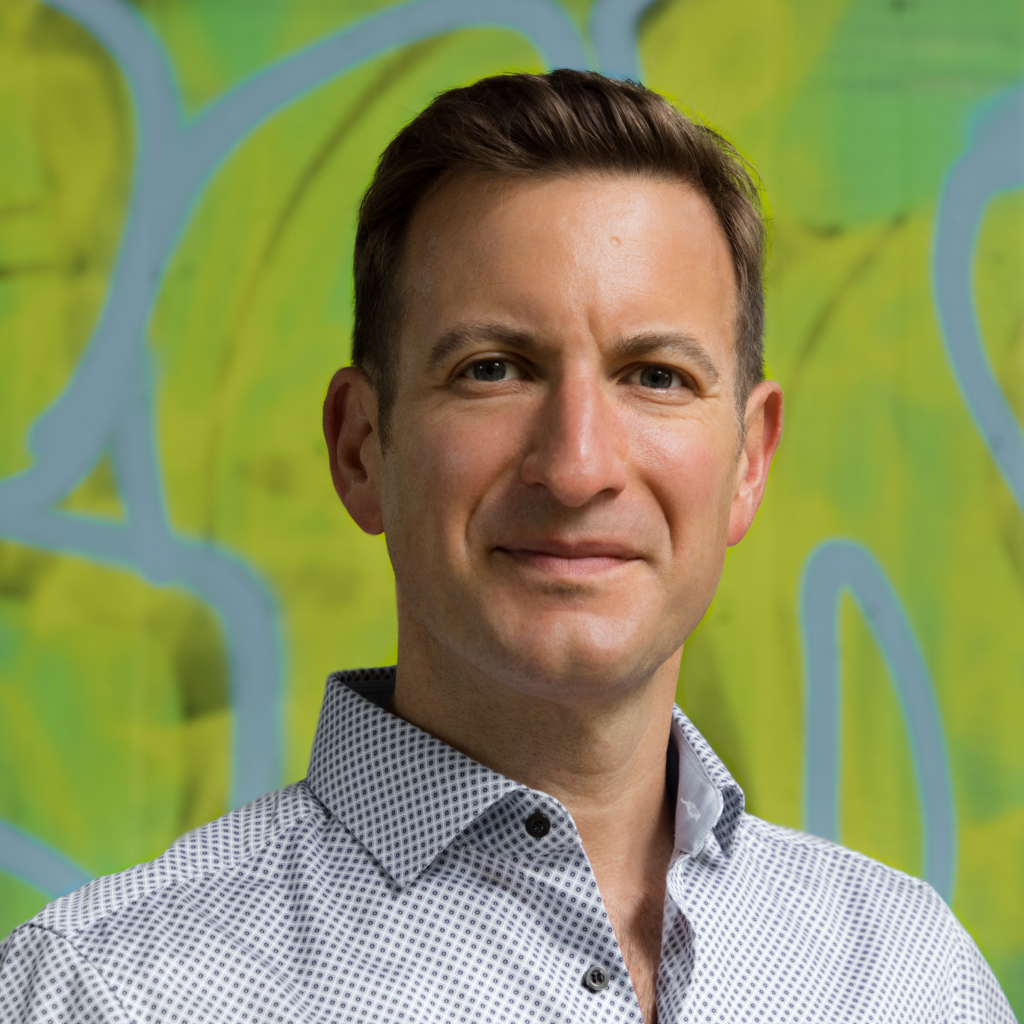
5 Minutes with Cyrill
Check out my video series 5 Minutes with Cyrill where I try to explain relevant concepts from robotics and computer vision within 5 minutes.
Research Interests in Robotics and Photogrammetry
- Localization, Mapping, SLAM, Bundle Adjustment
- Autonomous Navigation
- Visual and Laser Perception
- Scene Analysis and Classification
- Autonomous Cars
- Robotics for Agriculture
- Unmanned Aerial Vehicles
Short CV
Cyrill Stachniss is a Full Professor at the University of Bonn and heads the Lab for Photogrammetry and Robotics. He is additionally a Visiting Professor in Engineering at the University of Oxford and is with the Lamarr Institute for Machine Learning and Artificial Intelligence. Before working in Bonn, he was a lecturer at the University of Freiburg’s AIS Lab, a guest lecturer at the University of Zaragoza in Spain, and a senior researcher at the Swiss Federal Institute of Technology in the ALS Lab of Roland Siegwart. Cyrill Stachniss finished his habilitation in 2009 and received his Ph.D. thesis entitled “Exploration and Mapping with Mobile Robots” supervised by Wolfram Burgard at the University of Freiburg in 2006. From 2008-2013, he was an associate editor of the IEEE Transactions on Robotics, since 2010 a Microsoft Research Faculty Fellow, and received the IEEE RAS Early Career Award in 2013. Since 2015, he is a senior editor for the IEEE Robotics and Automation Letters. He is the spokesperson of the DFG Cluster of Excellence EXC 2070 ”PhenoRob – Robotics and Phenotyping for Sustainable Crop Production” and of the DFG Research Unit FOR 1505 ”Mapping on Demand”. He was furthermore involved in the coordination of several EC-funded FP7 and H2020 projects. In his research, he focuses on probabilistic techniques in the context of mobile robotics, navigation, and perception. Central areas of his research are solutions to the simultaneous localization and mapping problem, visual perception, robot learning, self-driving cars, agricultural robotics, and unmanned aerial vehicles. He has coauthored more than 280 peer-reviewed publications. Extended CVAwards
- ICRA 2023 — Best Automation Paper for: Target‑Aware Implicit Mapping for Agricultural Crop Inspection
- IROS 2022 — Finalists Best Agri‐Robotics Paper for: Contrastive 3D Shape Completion and Reconstruction for Agricultural Robots using RGB‐D Frames
- RAM 2022 — IEEE Robotics & Automation Magazine Best Paper Award for 2021 for: Building an Aerial-Ground Robotics System for Precision Farming: An Adaptable Solution
- ICRA 2022 — ICRA Outstanding Automation Paper Award (2022) for: Precise 3D Reconstruction of Plants from UAV Imagery Combining Bundle Adjustment and Template Matching
- ICCV 2021 — Best Result on the Segmenting and Tracking Every Point and Pixel Workshop at ICCV 2021 for: Contrastive Instance Association for 4D Panoptic Segmentation
- Faculty Award 2021 for Geodesy for N. Chebrolu’s work: Adaptive Robust Kernels for Non-Linear Least Squares Problems
- IROS 2020 — Best Agri-Robotics Paper Award (2020) for: Unsupervised Domain Adaptation for Transferring Plant Classification Systems to New Field Environments, Crops, and Robots
- RSS 2020 — Best Systems Paper Finalist (2020) for: OverlapNet – Loop Closing for LiDAR-based SLAM
- Faculty Award 2019 for Geodesy for I. Bogoslavskyi’s work: General Framework for Flexible Multi-cue Photometric Point Cloud Registration
- ICRA 2018 Workshop — Best Demo Award at the ICRA 2018 Workshop on Multimodal Robot Perception: Perception, Inference, and Learning for Joint Semantic, Geometric, and Physical Understanding (2018)
- ICRA 2018 — Best Service Paper Finalist (2018) for: Real-Time Semantic Segmentation of Crop and Weed for Precision Agriculture Robots Leveraging Background Knowledge in CNN
- IROS 2017 — Best Application Paper Finalist (2017) for: Semi-Supervised Online Visual Crop and Weed Classification in Precision Farming Exploiting Plant Arrangement
- ICRA 2017 — Best Automation Paper Award by the IEEE Robotics and Automation Society (2017) for: UAV-Based Crop and Weed Classification for Smart Farming
- ICRA 2015 — Finalist Best Service Robotics paper (2015) for: Robot, Organize my Shelves! Tidying up Objects by Predicting User Preferences
- Faculty Teaching Award of the Faculty of Engineering, Freiburg Univ. (Fakultätslehrpreis) (2012/2013)
- IEEE Robotics and Automation Society Early Career Award (2013) for my contributions to mobile robot exploration and SLAM
- ICRA 2013 — Best Associate Editor Award (2013)
- ICRA 2013 — Finalist best student paper (2013) for: Robust Map Optimization Using Dynamic Covariance Scaling
- Robotics: Science and Systems Early Career Spotlight (2012)
- Microsoft Research Faculty Fellow (2010)
- 7th EURON Georges Giralt Award for the best robotics thesis in Europe in 2006 (received in 2008)
- Wolfgang-Gentner PhD Award (2006) for my PhD thesis Exploration and Mapping with Mobile Robots
- ICRA 2005 — Finalist best student paper (2005) for the paper Supervised Learning of Places from Range Data using AdaBoost
- ICASE-IROS 2004 — Best paper award on applications (2005) for the paper Grid-based FastSLAM and Exploration with Active Loop Closing
- Award of the German Engineering Society, VDI (2003) for my master’s thesis Zielgerichtete Kollisionsvermeidung fuer mobile Roboter in dynamischen Umgebungen
Publications
2024
- F. Magistri, T. Läbe, E. Marks, S. Nagulavancha, Y. Pan, C. Smitt, L. Klingbeil, M. Halstead, H. Kuhlmann, C. McCool, J. Behley, and C. Stachniss, “A Dataset and Benchmark for Shape Completion of Fruits for Agricultural Robotics,” arXiv Preprint, 2024.
[BibTeX] [PDF]@article{magistri2024arxiv, title={{A Dataset and Benchmark for Shape Completion of Fruits for Agricultural Robotics}}, author={F. Magistri and T. L\"abe and E. Marks and S. Nagulavancha and Y. Pan and C. Smitt and L. Klingbeil and M. Halstead and H. Kuhlmann and C. McCool and J. Behley and C. Stachniss}, journal = arxiv, year=2024, eprint={2407.13304}, }
- Y. Pan, X. Zhong, L. Wiesmann, T. Posewsky, J. Behley, and C. Stachniss, “PIN-SLAM: LiDAR SLAM Using a Point-Based Implicit Neural Representation for Achieving Global Map Consistency,” IEEE Transactions on Robotics (TRO), 2024. doi:10.1109/TRO.2024.3422055
[BibTeX] [PDF] [Code]@article{pan2024tro, author = {Y. Pan and X. Zhong and L. Wiesmann and T. Posewsky and J. Behley and C. Stachniss}, title = {{PIN-SLAM: LiDAR SLAM Using a Point-Based Implicit Neural Representation for Achieving Global Map Consistency}}, journal = tro, year = {2024}, volume = {}, number = {}, pages = {}, doi = {10.1109/TRO.2024.3422055}, codeurl = {https://github.com/PRBonn/PIN_SLAM}, note = {Accepted} }
- J. Weyler, F. Magistri, E. Marks, Y. L. Chong, M. Sodano, G. Roggiolani, N. Chebrolu, C. Stachniss, and J. Behley, “PhenoBench: A Large Dataset and Benchmarks for Semantic Image Interpretation in the Agricultural Domain,” IEEE Trans. on Pattern Analysis and Machine Intelligence (TPAMI), 2024. doi:10.1109/TPAMI.2024.3419548
[BibTeX] [PDF] [Code]@article{weyler2024tpami, author = {J. Weyler and F. Magistri and E. Marks and Y.L. Chong and M. Sodano and G. Roggiolani and N. Chebrolu and C. Stachniss and J. Behley}, title = {{PhenoBench: A Large Dataset and Benchmarks for Semantic Image Interpretation in the Agricultural Domain}}, journal = tpami, year = {2024}, volume = {}, number = {}, pages = {}, doi = {10.1109/TPAMI.2024.3419548}, codeurl = {https://github.com/PRBonn/phenobench}, note = {Accepted} }
- D. Casado Herraez, L. Chang, M. Zeller, L. Wiesmann, J. Behley, M. Heidingsfeld, and C. Stachniss, “SPR: Single-Scan Radar Place Recognition,” IEEE Robotics and Automation Letters (RA-L), 2024.
[BibTeX]@article{casado-herraez2024ral, author = {Casado Herraez, D. and L. Chang and M. Zeller and L. Wiesmann and J. Behley and M. Heidingsfeld and C. Stachniss}, title = {{SPR: Single-Scan Radar Place Recognition}}, journal = ral, year = {2024}, volume = {}, number = {}, pages = {}, doi = {}, note = {Accepted} }
- F. Magistri, Y. Pan, J. Bartels, J. Behley, C. Stachniss, and C. Lehnert, “Improving Robotic Fruit Harvesting Within Cluttered Environments Through 3D Shape Completion,” IEEE Robotics and Automation Letters (RA-L), vol. 9, iss. 8, p. 7357–7364, 2024. doi:10.1109/LRA.2024.3421788
[BibTeX] [PDF]@article{magistri2024ral, author = {F. Magistri and Y. Pan and J. Bartels and J. Behley and C. Stachniss and C. Lehnert}, title = {{Improving Robotic Fruit Harvesting Within Cluttered Environments Through 3D Shape Completion}}, journal = ral, volume = {9}, number = {8}, pages = {7357--7364}, year = 2024, doi = {10.1109/LRA.2024.3421788}, }
- I. B. Opra, B. Le Dem, J. Walls, D. Lukarski, and C. Stachniss, “Leveraging GNSS and Onboard Visual Data from Consumer Vehicles for Robust Road Network Estimation,” in Proc. of the IEEE/RSJ Intl. Conf. on Intelligent Robots and Systems (IROS), 2024.
[BibTeX]@inproceedings{opra2024iros, author = {I.B. Opra and Le Dem, B. and J. Walls and D. Lukarski and C. Stachniss}, title = {{Leveraging GNSS and Onboard Visual Data from Consumer Vehicles for Robust Road Network Estimation}}, booktitle = iros, year = 2024, note = {Accepted} }
- L. Lobefaro, M. V. R. Malladi, T. Guadagnino, and C. Stachniss, “Spatio-Temporal Consistent Mapping of Growing Plants for Agricultural Robots in the Wild,” in Proc. of the IEEE/RSJ Intl. Conf. on Intelligent Robots and Systems (IROS), 2024.
[BibTeX] [PDF] [Code]@inproceedings{lobefaro2024iros, author = {L. Lobefaro and M.V.R. Malladi and T. Guadagnino and C. Stachniss}, title = {{Spatio-Temporal Consistent Mapping of Growing Plants for Agricultural Robots in the Wild}}, booktitle = iros, year = 2024, note = {Accepted}, codeurl = {https://github.com/PRBonn/spatio-temporal-mapping.git}, }
- E. A. Marks, J. Bömer, F. Magistri, A. Sah, J. Behley, and C. Stachniss, “BonnBeetClouds3D: A Dataset Towards Point Cloud-Based Organ-Level Phenotyping of Sugar Beet Plants Under Real Field Conditions,” in Proc. of the IEEE/RSJ Intl. Conf. on Intelligent Robots and Systems (IROS), 2024.
[BibTeX]@inproceedings{marks2024iros, author = {E.A. Marks and J. B\"omer and F. Magistri and A. Sah and J. Behley and C. Stachniss}, title = {{BonnBeetClouds3D: A Dataset Towards Point Cloud-Based Organ-Level Phenotyping of Sugar Beet Plants Under Real Field Conditions}}, booktitle = iros, year = 2024, note = {Accepted} }
- H. Lim, S. Jang, B. Mersch, J. Behley, H. Myung, and C. Stachniss, “HeLiMOS: A Dataset for Moving Object Segmentation in 3D Point Clouds From Heterogeneous LiDAR Sensors,” in Proc. of the IEEE/RSJ Intl. Conf. on Intelligent Robots and Systems (IROS), 2024.
[BibTeX]@inproceedings{lim2024iros, author = {H. Lim and S. Jang and B. Mersch and J. Behley and H. Myung and C. Stachniss}, title = {{HeLiMOS: A Dataset for Moving Object Segmentation in 3D Point Clouds From Heterogeneous LiDAR Sensors}}, booktitle = iros, year = 2024, note = {Accepted} }
- R. Schirmer, N. Vaskevicius, P. Biber, and C. Stachniss, “Fast Global Point Cloud Registration using Semantic NDT,” in Proc. of the IEEE/RSJ Intl. Conf. on Intelligent Robots and Systems (IROS), 2024.
[BibTeX]@inproceedings{schirmer2024iros, author = {R. Schirmer and N. Vaskevicius and P. Biber and C. Stachniss}, title = {{Fast Global Point Cloud Registration using Semantic NDT}}, booktitle = iros, year = 2024, note = {Accepted} }
- L. Jin, H. Kuang, Y. Pan, C. Stachniss, and M. Popović, “STAIR: Semantic-Targeted Active Implicit Reconstruction,” in Proc. of the IEEE/RSJ Intl. Conf. on Intelligent Robots and Systems (IROS), 2024.
[BibTeX]@inproceedings{jin2024iros, author = {L. Jin and H. Kuang and Y. Pan and C. Stachniss and M. Popovi\'c}, title = {{STAIR: Semantic-Targeted Active Implicit Reconstruction}}, booktitle = iros, year = 2024, note = {Accepted} }
- S. Pan, L. Jin, X. Huang, C. Stachniss, M. Popović, and M. Bennewitz, “Exploiting Priors from 3D Diffusion Models for RGB-Based One-Shot View Planning,” in Proc. of the IEEE/RSJ Intl. Conf. on Intelligent Robots and Systems (IROS), 2024.
[BibTeX]@inproceedings{pan2024iros, author = {S. Pan and L. Jin and X. Huang and C. Stachniss and M. Popovi\'c and M. Bennewitz}, title = {{Exploiting Priors from 3D Diffusion Models for RGB-Based One-Shot View Planning}}, booktitle = iros, year = 2024, note = {Accepted} }
- J. Bömer, F. Esser, E. A. Marks, R. A. Rosu, S. Behnke, L. Klingbeil, H. Kuhlmann, C. Stachniss, A. -K. Mahlein, and S. Paulus, “A 3D Printed Plant Model for Accurate and Reliable 3D Plant Phenotyping,” GigaScience, vol. 13, p. giae035, 2024. doi:10.1093/gigascience/giae035
[BibTeX] [PDF]@article{boemer2024giga, author = {J. B\"omer and F. Esser and E.A. Marks and R.A. Rosu and S. Behnke and L. Klingbeil and H. Kuhlmann and C. Stachniss and A.-K. Mahlein and S. Paulus}, title = {{A 3D Printed Plant Model for Accurate and Reliable 3D Plant Phenotyping}}, journal = giga, volume = {13}, number = {}, pages = {giae035}, issn = {2047-217X}, year = 2024, doi = {10.1093/gigascience/giae035}, url = {https://academic.oup.com/gigascience/article-pdf/doi/10.1093/gigascience/giae035/58270533/giae035.pdf}, }
- H. Storm, S. J. Seidel, L. Klingbeil, F. Ewert, H. Vereecken, W. Amelung, S. Behnke, M. Bennewitz, J. Börner, T. Döring, J. Gall, A. -K. Mahlein, C. McCool, U. Rascher, S. Wrobel, A. Schnepf, C. Stachniss, and H. Kuhlmann, “Research Priorities to Leverage Smart Digital Technologies for Sustainable Crop Production,” European Journal of Agronomy, vol. 156, p. 127178, 2024. doi:https://doi.org/10.1016/j.eja.2024.127178
[BibTeX] [PDF]@article{storm2024eja, author = {H. Storm and S.J. Seidel and L. Klingbeil and F. Ewert and H. Vereecken and W. Amelung and S. Behnke and M. Bennewitz and J. B\"orner and T. D\"oring and J. Gall and A.-K. Mahlein and C. McCool and U. Rascher and S. Wrobel and A. Schnepf and C. Stachniss and H. Kuhlmann}, title = {{Research Priorities to Leverage Smart Digital Technologies for Sustainable Crop Production}}, journal = {European Journal of Agronomy}, volume = {156}, pages = {127178}, year = {2024}, issn = {1161-0301}, doi = {https://doi.org/10.1016/j.eja.2024.127178}, url = {https://www.sciencedirect.com/science/article/pii/S1161030124000996}, }
- J. Hertzberg, B. Kisliuk, J. C. Krause, and C. Stachniss, “Interview: Cyrill Stachniss’ View on AI in Agriculture,” German Journal of Artificial Intelligence (KI), 2024. doi:10.1007/s13218-023-00831-8
[BibTeX] [PDF]@article{hertzberg2024ki, author = {J. Hertzberg and B. Kisliuk and J.C. Krause and C. Stachniss}, title = {{Interview: Cyrill Stachniss’ View on AI in Agriculture}}, journal = {German Journal of Artificial Intelligence (KI)}, year = {2024}, doi = {10.1007/s13218-023-00831-8}, url = {https://link.springer.com/article/10.1007/s13218-023-00831-8}, }
- M. Sodano, F. Magistri, L. Nunes, J. Behley, and C. Stachniss, “Open-World Semantic Segmentation Including Class Similarity,” in Proc. of the IEEE/CVF Conf. on Computer Vision and Pattern Recognition (CVPR), 2024.
[BibTeX] [PDF] [Code] [Video]@inproceedings{sodano2024cvpr, author = {M. Sodano and F. Magistri and L. Nunes and J. Behley and C. Stachniss}, title = {{Open-World Semantic Segmentation Including Class Similarity}}, booktitle = cvpr, year = 2024, codeurl = {https://github.com/PRBonn/ContMAV}, videourl = {https://youtu.be/ei2cbyPQgag?si=_KabYyfjzzJZi1Zy}, }
- L. Nunes, R. Marcuzzi, B. Mersch, J. Behley, and C. Stachniss, “Scaling Diffusion Models to Real-World 3D LiDAR Scene Completion,” in Proc. of the IEEE/CVF Conf. on Computer Vision and Pattern Recognition (CVPR), 2024.
[BibTeX] [PDF] [Code] [Video]@inproceedings{nunes2024cvpr, author = {L. Nunes and R. Marcuzzi and B. Mersch and J. Behley and C. Stachniss}, title = {{Scaling Diffusion Models to Real-World 3D LiDAR Scene Completion}}, booktitle = cvpr, year = 2024, codeurl = {https://github.com/PRBonn/LiDiff}, videourl = {https://youtu.be/XWu8svlMKUo}, }
- X. Zhong, Y. Pan, C. Stachniss, and J. Behley, “3D LiDAR Mapping in Dynamic Environments using a 4D Implicit Neural Representation,” in Proc. of the IEEE/CVF Conf. on Computer Vision and Pattern Recognition (CVPR), 2024.
[BibTeX] [PDF] [Code] [Video]@inproceedings{zhong2024cvpr, author = {X. Zhong and Y. Pan and C. Stachniss and J. Behley}, title = {{3D LiDAR Mapping in Dynamic Environments using a 4D Implicit Neural Representation}}, booktitle = cvpr, year = 2024, codeurl = {https://github.com/PRBonn/4dNDF}, videourl ={https://youtu.be/pRNKRcTkxjs} }
- H. Yin, X. Xu, S. Lu, X. Chen, R. Xiong, S. Shen, C. Stachniss, and Y. Wang, “A Survey on Global LiDAR Localization: Challenges, Advances and Open Problems,” Intl. Journal of Computer Vision (IJCV), 2024. doi:10.1007/s11263-024-02019-5
[BibTeX] [PDF]@article{yin2024ijcv, author = {H. Yin and X. Xu and S. Lu and X. Chen and R. Xiong and S. Shen and C. Stachniss and Y. Wang}, title = {{A Survey on Global LiDAR Localization: Challenges, Advances and Open Problems}}, journal = {Intl. Journal of Computer Vision (IJCV)}, volume = {}, number = {}, pages = {}, year = 2024, doi = {10.1007/s11263-024-02019-5}, url = {https://www.ipb.uni-bonn.de/wp-content/papercite-data/pdf/yin2024ijcv-preprint.pdf}, }
- S. Pan, L. Jin, X. Huang, C. Stachniss, M. Popovic, and M. Bennewitz, “Exploiting Priors from 3D Diffusion Models for RGB-Based One-Shot View Planning,” in In Proc. of the ICRA Workshop On Neural Fields In Robotics (RoboNerF), 2024.
[BibTeX]@inproceedings{pan2024icraws, title={{Exploiting Priors from 3D Diffusion Models for {RGB}-Based One-Shot View Planning}}, author={S. Pan and L. Jin and X. Huang and C. Stachniss and M. Popovic and M. Bennewitz}, booktitle={In Proc. of the ICRA Workshop On Neural Fields In Robotics (RoboNerF)}, year={2024}, }
- I. Hroob, B. Mersch, C. Stachniss, and M. Hanheide, “Generalizable Stable Points Segmentation for 3D LiDAR Scan-to-Map Long-Term Localization,” IEEE Robotics and Automation Letters (RA-L), vol. 9, iss. 4, pp. 3546-3553, 2024. doi:10.1109/LRA.2024.3368236
[BibTeX] [PDF] [Code] [Video]@article{hroob2024ral, author = {I. Hroob and B. Mersch and C. Stachniss and M. Hanheide}, title = {{Generalizable Stable Points Segmentation for 3D LiDAR Scan-to-Map Long-Term Localization}}, journal = ral, volume = {9}, number = {4}, pages = {3546-3553}, year = 2024, doi = {10.1109/LRA.2024.3368236}, videourl = {https://youtu.be/aRLStFQEXbc}, codeurl = {https://github.com/ibrahimhroob/SPS}, }
- M. Zeller, D. Casado Herraez, J. Behley, M. Heidingsfeld, and C. Stachniss, “Radar Tracker: Moving Instance Tracking in Sparse and Noisy Radar Point Clouds,” in Proc. of the IEEE Intl. Conf. on Robotics & Automation (ICRA), 2024.
[BibTeX] [PDF] [Video]@inproceedings{zeller2024icra, author = {M. Zeller and Casado Herraez, Daniel and J. Behley and M. Heidingsfeld and C. Stachniss}, title = {{Radar Tracker: Moving Instance Tracking in Sparse and Noisy Radar Point Clouds}}, booktitle = icra, year = 2024, videourl = {https://youtu.be/PixfkN8cMig}, }
- D. Casado Herraez, M. Zeller, L. Chang, I. Vizzo, M. Heidingsfeld, and C. Stachniss, “Radar-Only Odometry and Mapping for Autonomous Vehicles,” in Proc. of the IEEE Intl. Conf. on Robotics & Automation (ICRA), 2024.
[BibTeX] [PDF] [Video]@inproceedings{casado-herraez2024icra, author = {Casado Herraez, Daniel and M. Zeller and Chang, Le and I. Vizzo and M. Heidingsfeld and C. Stachniss}, title = {{Radar-Only Odometry and Mapping for Autonomous Vehicles}}, booktitle = icra, year = 2024, videourl = {https://youtu.be/_xWDXyyKEok} }
- M. V. R. Malladi, T. Guadagnino, L. Lobefaro, M. Mattamala, H. Griess, J. Schweier, N. Chebrolu, M. Fallon, J. Behley, and C. Stachniss, “Tree Instance Segmentation and Traits Estimation for Forestry Environments Exploiting LiDAR Data ,” in Proc. of the IEEE Intl. Conf. on Robotics & Automation (ICRA), 2024.
[BibTeX] [PDF] [Code] [Video]@inproceedings{malladi2024icra, author = {M.V.R. Malladi and T. Guadagnino and L. Lobefaro and M. Mattamala and H. Griess and J. Schweier and N. Chebrolu and M. Fallon and J. Behley and C. Stachniss}, title = {{Tree Instance Segmentation and Traits Estimation for Forestry Environments Exploiting LiDAR Data }}, booktitle = icra, year = 2024, videourl = {https://youtu.be/14uuCxmfGco}, codeurl = {https://github.com/PRBonn/forest_inventory_pipeline}, }
- F. Magistri, R. Marcuzzi, E. A. Marks, M. Sodano, J. Behley, and C. Stachniss, “Efficient and Accurate Transformer-Based 3D Shape Completion and Reconstruction of Fruits for Agricultural Robots,” in Proc. of the IEEE Intl. Conf. on Robotics & Automation (ICRA), 2024.
[BibTeX] [PDF] [Code] [Video]@inproceedings{magistri2024icra, author = {F. Magistri and R. Marcuzzi and E.A. Marks and M. Sodano and J. Behley and C. Stachniss}, title = {{Efficient and Accurate Transformer-Based 3D Shape Completion and Reconstruction of Fruits for Agricultural Robots}}, booktitle = icra, year = 2024, videourl = {https://youtu.be/U1xxnUGrVL4}, codeurl = {https://github.com/PRBonn/TCoRe}, }
- S. Gupta, T. Guadagnino, B. Mersch, I. Vizzo, and C. Stachniss, “Effectively Detecting Loop Closures using Point Cloud Density Maps,” in Proc. of the IEEE Intl. Conf. on Robotics & Automation (ICRA), 2024.
[BibTeX] [PDF] [Code] [Video]@inproceedings{gupta2024icra, author = {S. Gupta and T. Guadagnino and B. Mersch and I. Vizzo and C. Stachniss}, title = {{Effectively Detecting Loop Closures using Point Cloud Density Maps}}, booktitle = icra, year = 2024, codeurl = {https://github.com/PRBonn/MapClosures}, videourl = {https://youtu.be/BpwR_aLXrNo}, }
- Y. Wu, T. Guadagnino, L. Wiesmann, L. Klingbeil, C. Stachniss, and H. Kuhlmann, “LIO-EKF: High Frequency LiDAR-Inertial Odometry using Extended Kalman Filters,” in Proc. of the IEEE Intl. Conf. on Robotics & Automation (ICRA), 2024.
[BibTeX] [PDF] [Code] [Video]@inproceedings{wu2024icra, author = {Y. Wu and T. Guadagnino and L. Wiesmann and L. Klingbeil and C. Stachniss and H. Kuhlmann}, title = {{LIO-EKF: High Frequency LiDAR-Inertial Odometry using Extended Kalman Filters}}, booktitle = icra, year = 2024, codeurl = {https://github.com/YibinWu/LIO-EKF}, videourl = {https://youtu.be/MoJTqEYl1ME}, }
- M. Zeller, V. S. Sandhu, B. Mersch, J. Behley, M. Heidingsfeld, and C. Stachniss, “Radar Instance Transformer: Reliable Moving Instance Segmentation in Sparse Radar Point Clouds,” IEEE Transactions on Robotics (TRO), vol. 40, pp. 2357-2372, 2024. doi:10.1109/TRO.2023.3338972
[BibTeX] [PDF] [Video]@article{zeller2024tro, author = {M. Zeller and Sandhu, V.S. and B. Mersch and J. Behley and M. Heidingsfeld and C. Stachniss}, title = {{Radar Instance Transformer: Reliable Moving Instance Segmentation in Sparse Radar Point Clouds}}, journal = tro, year = {2024}, volume = {40}, doi = {10.1109/TRO.2023.3338972}, pages = {2357-2372}, videourl = {https://www.youtube.com/watch?v=v-iXbJEcqPM} }
- J. Rückin, F. Magistri, C. Stachniss, and M. Popović, “Semi-Supervised Active Learning for Semantic Segmentation in Unknown Environments Using Informative Path Planning,” IEEE Robotics and Automation Letters (RA-L), vol. 9, iss. 3, pp. 2662-2669, 2024. doi:10.1109/LRA.2024.3359970
[BibTeX] [PDF] [Code]@article{rueckin2024ral, author = {J. R\"uckin and F. Magistri and C. Stachniss and M. Popovi\'c}, title = {{Semi-Supervised Active Learning for Semantic Segmentation in Unknown Environments Using Informative Path Planning}}, journal = ral, year = {2024}, volume = {9}, number = {3}, pages = {2662-2669}, issn = {2377-3766}, doi = {10.1109/LRA.2024.3359970}, codeurl = {https://github.com/dmar-bonn/ipp-ssl}, }
- J. Weyler, T. Läbe, J. Behley, and C. Stachniss, “Panoptic Segmentation with Partial Annotations for Agricultural Robots,” IEEE Robotics and Automation Letters (RA-L), vol. 9, iss. 2, pp. 1660-1667, 2024. doi:10.1109/LRA.2023.3346760
[BibTeX] [PDF] [Code]@article{weyler2024ral, author = {J. Weyler and T. L\"abe and J. Behley and C. Stachniss}, title = {{Panoptic Segmentation with Partial Annotations for Agricultural Robots}}, journal = ral, year = {2024}, volume = {9}, number = {2}, pages = {1660-1667}, issn = {2377-3766}, doi = {10.1109/LRA.2023.3346760}, codeurl = {https://github.com/PRBonn/PSPA} }
- C. Smitt, M. A. Halstead, P. Zimmer, T. Läbe, E. Guclu, C. Stachniss, and C. S. McCool, “PAg-NeRF: Towards fast and efficient end-to-end panoptic 3D representations for agricultural robotics,” IEEE Robotics and Automation Letters (RA-L), vol. 9, iss. 1, pp. 907-914, 2024. doi:10.1109/LRA.2023.3338515
[BibTeX] [PDF] [Code]@article{smitt2024ral-pagn, author = {C. Smitt and M.A. Halstead and P. Zimmer and T. L\"abe and E. Guclu and C. Stachniss and C.S. McCool}, title = {{PAg-NeRF: Towards fast and efficient end-to-end panoptic 3D representations for agricultural robotics}}, journal = ral, year = {2024}, volume = {9}, number = {1}, pages = {907-914}, issn = {2377-3766}, doi = {10.1109/LRA.2023.3338515}, codeurl = {https://github.com/Agricultural-Robotics-Bonn/pagnerf} }
2023
- R. Roscher, L. Roth, C. Stachniss, and A. Walter, “Data-Centric Digital Agriculture: A Perspective,” arXiv Preprint, 2023.
[BibTeX]@article{roscher2023arxiv-dcda, title={{Data-Centric Digital Agriculture: A Perspective}}, author={R. Roscher and L. Roth and C. Stachniss and A. Walter}, year={2023}, eprint={2312.03437}, journal = arxiv, year = {2023} }
- C. Gomez, A. C. Hernandez, R. Barber, and C. Stachniss, “Localization Exploiting Semantic and Metric Information in Non-static Indoor Environments,” Journal of Intelligent & Robotic Systems, vol. 109, iss. 86, 2023. doi:https://doi.org/10.1007/s10846-023-02021-y
[BibTeX] [PDF]@article{gomez2023jint, author = {C. Gomez and A.C. Hernandez and R. Barber and C. Stachniss}, title = {Localization Exploiting Semantic and Metric Information in Non-static Indoor Environments}, journal = jint, year = {2023}, volume = {109}, number = {86}, doi = {https://doi.org/10.1007/s10846-023-02021-y}, }
- R. Marcuzzi, L. Nunes, L. Wiesmann, E. Marks, J. Behley, and C. Stachniss, “Mask4D: End-to-End Mask-Based 4D Panoptic Segmentation for LiDAR Sequences,” IEEE Robotics and Automation Letters (RA-L), vol. 8, iss. 11, pp. 7487-7494, 2023. doi:10.1109/LRA.2023.3320020
[BibTeX] [PDF] [Code] [Video]@article{marcuzzi2023ral-meem, author = {R. Marcuzzi and L. Nunes and L. Wiesmann and E. Marks and J. Behley and C. Stachniss}, title = {{Mask4D: End-to-End Mask-Based 4D Panoptic Segmentation for LiDAR Sequences}}, journal = ral, year = {2023}, volume = {8}, number = {11}, pages = {7487-7494}, issn = {2377-3766}, doi = {10.1109/LRA.2023.3320020}, codeurl = {https://github.com/PRBonn/Mask4D}, videourl = {https://youtu.be/4WqK_gZlpfA}, }
- G. Roggiolani, F. Magistri, T. Guadagnino, J. Behley, and C. Stachniss, “Unsupervised Pre-Training for 3D Leaf Instance Segmentation,” IEEE Robotics and Automation Letters (RA-L), vol. 8, pp. 7448-7455, 2023. doi:10.1109/LRA.2023.3320018
[BibTeX] [PDF] [Code] [Video]@article{roggiolani2023ral, author = {G. Roggiolani and F. Magistri and T. Guadagnino and J. Behley and C. Stachniss}, title = {{Unsupervised Pre-Training for 3D Leaf Instance Segmentation}}, journal = ral, year = {2023}, volume = {8}, issue = {11}, codeurl = {https://github.com/PRBonn/Unsupervised-Pre-Training-for-3D-Leaf-Instance-Segmentation}, pages = {7448-7455}, doi = {10.1109/LRA.2023.3320018}, issn = {2377-3766}, videourl = {https://youtu.be/PbYVPPwVeKg}, }
- J. Rückin, F. Magistri, C. Stachniss, and M. Popovic, “An Informative Path Planning Framework for Active Learning in UAV-based Semantic Mapping,” IEEE Transactions on Robotics (TRO), vol. 39, iss. 6, pp. 4279-4296, 2023. doi:10.1109/TRO.2023.3313811
[BibTeX] [PDF] [Code]@article{rueckin2023tro, author = {J. R\"{u}ckin and F. Magistri and C. Stachniss and M. Popovic}, title = {{An Informative Path Planning Framework for Active Learning in UAV-based Semantic Mapping}}, journal = tro, year = {2023}, codeurl = {https://github.com/dmar-bonn/ipp-al-framework}, doi={10.1109/TRO.2023.3313811}, volume={39}, number={6}, pages={4279-4296}, }
- F. Magistri, J. Weyler, D. Gogoll, P. Lottes, J. Behley, N. Petrinic, and C. Stachniss, “From one Field to Another – Unsupervised Domain Adaptation for Semantic Segmentation in Agricultural Robotics,” Computers and Electronics in Agriculture, vol. 212, p. 108114, 2023. doi:https://doi.org/10.1016/j.compag.2023.108114
[BibTeX] [PDF]@article{magistri2023cea, author = {F. Magistri and J. Weyler and D. Gogoll and P. Lottes and J. Behley and N. Petrinic and C. Stachniss}, title = {From one Field to Another – Unsupervised Domain Adaptation for Semantic Segmentation in Agricultural Robotics}, journal = cea, year = {2023}, volume = {212}, pages = {108114}, doi = {https://doi.org/10.1016/j.compag.2023.108114}, }
- I. Vizzo, B. Mersch, L. Nunes, L. Wiesmann, T. Guadagnino, and C. Stachniss, “Toward Reproducible Version-Controlled Perception Platforms: Embracing Simplicity in Autonomous Vehicle Dataset Acquisition,” in Proc. of the Intl. Conf. on Intelligent Transportation Systems Workshops, 2023.
[BibTeX] [PDF] [Code]@inproceedings{vizzo2023itcsws, author = {I. Vizzo and B. Mersch and L. Nunes and L. Wiesmann and T. Guadagnino and C. Stachniss}, title = {{Toward Reproducible Version-Controlled Perception Platforms: Embracing Simplicity in Autonomous Vehicle Dataset Acquisition}}, booktitle = {Proc. of the Intl. Conf. on Intelligent Transportation Systems Workshops}, year = 2023, codeurl = {https://github.com/ipb-car/meta-workspace}, note = {accepted} }
- B. Mersch, T. Guadagnino, X. Chen, I. Vizzo, J. Behley, and C. Stachniss, “Building Volumetric Beliefs for Dynamic Environments Exploiting Map-Based Moving Object Segmentation,” IEEE Robotics and Automation Letters (RA-L), vol. 8, iss. 8, pp. 5180-5187, 2023. doi:10.1109/LRA.2023.3292583
[BibTeX] [PDF] [Code] [Video]@article{mersch2023ral, author = {B. Mersch and T. Guadagnino and X. Chen and I. Vizzo and J. Behley and C. Stachniss}, title = {{Building Volumetric Beliefs for Dynamic Environments Exploiting Map-Based Moving Object Segmentation}}, journal = ral, volume = {8}, number = {8}, pages = {5180-5187}, year = 2023, issn = {2377-3766}, doi = {10.1109/LRA.2023.3292583}, videourl = {https://youtu.be/aeXhvkwtDbI}, codeurl = {https://github.com/PRBonn/MapMOS}, }
- Y. L. Chong, J. Weyler, P. Lottes, J. Behley, and C. Stachniss, “Unsupervised Generation of Labeled Training Images for Crop-Weed Segmentation in New Fields and on Different Robotic Platforms,” IEEE Robotics and Automation Letters (RA-L), vol. 8, iss. 8, p. 5259–5266, 2023. doi:10.1109/LRA.2023.3293356
[BibTeX] [PDF] [Code] [Video]@article{chong2023ral, author = {Y.L. Chong and J. Weyler and P. Lottes and J. Behley and C. Stachniss}, title = {{Unsupervised Generation of Labeled Training Images for Crop-Weed Segmentation in New Fields and on Different Robotic Platforms}}, journal = ral, volume = {8}, number = {8}, pages = {5259--5266}, year = 2023, issn = {2377-3766}, doi = {10.1109/LRA.2023.3293356}, videourl = {https://youtu.be/SpvrR9sgf2k}, codeurl = {https://github.com/PRBonn/StyleGenForLabels} }
- L. Lobefaro, M. V. R. Malladi, O. Vysotska, T. Guadagnino, and C. Stachniss, “Estimating 4D Data Associations Towards Spatial-Temporal Mapping of Growing Plants for Agricultural Robots,” in Proc. of the IEEE/RSJ Intl. Conf. on Intelligent Robots and Systems (IROS), 2023.
[BibTeX] [PDF] [Code] [Video]@inproceedings{lobefaro2023iros, author = {L. Lobefaro and M.V.R. Malladi and O. Vysotska and T. Guadagnino and C. Stachniss}, title = {{Estimating 4D Data Associations Towards Spatial-Temporal Mapping of Growing Plants for Agricultural Robots}}, booktitle = iros, year = 2023, codeurl = {https://github.com/PRBonn/plants_temporal_matcher}, videourl = {https://youtu.be/HpJPIzmXoag} }
- Y. Pan, F. Magistri, T. Läbe, E. Marks, C. Smitt, C. S. McCool, J. Behley, and C. Stachniss, “Panoptic Mapping with Fruit Completion and Pose Estimation for Horticultural Robots,” in Proc. of the IEEE/RSJ Intl. Conf. on Intelligent Robots and Systems (IROS), 2023.
[BibTeX] [PDF] [Code] [Video]@inproceedings{pan2023iros, author = {Y. Pan and F. Magistri and T. L\"abe and E. Marks and C. Smitt and C.S. McCool and J. Behley and C. Stachniss}, title = {{Panoptic Mapping with Fruit Completion and Pose Estimation for Horticultural Robots}}, booktitle = iros, year = 2023, codeurl = {https://github.com/PRBonn/HortiMapping}, videourl = {https://youtu.be/fSyHBhskjqA} }
- Y. Goel, N. Vaskevicius, L. Palmieri, N. Chebrolu, K. O. Arras, and C. Stachniss, “Semantically Informed MPC for Context-Aware Robot Exploration,” in Proc. of the IEEE/RSJ Intl. Conf. on Intelligent Robots and Systems (IROS), 2023.
[BibTeX] [PDF]@inproceedings{goel2023iros, author = {Y. Goel and N. Vaskevicius and L. Palmieri and N. Chebrolu and K.O. Arras and C. Stachniss}, title = {{Semantically Informed MPC for Context-Aware Robot Exploration}}, booktitle = iros, year = 2023, }
- N. Zimmerman, M. Sodano, E. Marks, J. Behley, and C. Stachniss, “Constructing Metric-Semantic Maps using Floor Plan Priors for Long-Term Indoor Localization,” in Proc. of the IEEE/RSJ Intl. Conf. on Intelligent Robots and Systems (IROS), 2023.
[BibTeX] [PDF] [Code] [Video]@inproceedings{zimmerman2023iros, author = {N. Zimmerman and M. Sodano and E. Marks and J. Behley and C. Stachniss}, title = {{Constructing Metric-Semantic Maps using Floor Plan Priors for Long-Term Indoor Localization}}, booktitle = iros, year = 2023, codeurl = {https://github.com/PRBonn/SIMP}, videourl = {https://youtu.be/9ZGd5lJbG4s} }
- J. Weyler, F. Magistri, E. Marks, Y. L. Chong, M. Sodano, G. Roggiolani, N. Chebrolu, C. Stachniss, and J. Behley, “PhenoBench –- A Large Dataset and Benchmarks for Semantic Image Interpretation in the Agricultural Domain,” arXiv preprint, vol. arXiv:2306.04557, 2023.
[BibTeX] [PDF] [Code]@article{weyler2023arxiv, author = {Jan Weyler and Federico Magistri and Elias Marks and Yue Linn Chong and Matteo Sodano and Gianmarco Roggiolani and Nived Chebrolu and Cyrill Stachniss and Jens Behley}, title = {{PhenoBench --- A Large Dataset and Benchmarks for Semantic Image Interpretation in the Agricultural Domain}}, journal = {arXiv preprint}, volume = {arXiv:2306.04557}, year = {2023}, codeurl = {https://github.com/PRBonn/phenobench} }
- L. Wiesmann, T. Guadagnino, I. Vizzo, N. Zimmerman, Y. Pan, H. Kuang, J. Behley, and C. Stachniss, “LocNDF: Neural Distance Field Mapping for Robot Localization,” IEEE Robotics and Automation Letters (RA-L), vol. 8, iss. 8, p. 4999–5006, 2023. doi:10.1109/LRA.2023.3291274
[BibTeX] [PDF] [Code] [Video]@article{wiesmann2023ral-icra, author = {L. Wiesmann and T. Guadagnino and I. Vizzo and N. Zimmerman and Y. Pan and H. Kuang and J. Behley and C. Stachniss}, title = {{LocNDF: Neural Distance Field Mapping for Robot Localization}}, journal = ral, volume = {8}, number = {8}, pages = {4999--5006}, year = 2023, url = {https://www.ipb.uni-bonn.de/wp-content/papercite-data/pdf/wiesmann2023ral-icra.pdf}, issn = {2377-3766}, doi = {10.1109/LRA.2023.3291274}, codeurl = {https://github.com/PRBonn/LocNDF}, videourl = {https://youtu.be/-0idH21BpMI}, }
- E. Marks, M. Sodano, F. Magistri, L. Wiesmann, D. Desai, R. Marcuzzi, J. Behley, and C. Stachniss, “High Precision Leaf Instance Segmentation in Point Clouds Obtained Under Real Field Conditions,” IEEE Robotics and Automation Letters (RA-L), vol. 8, iss. 8, pp. 4791-4798, 2023. doi:10.1109/LRA.2023.3288383
[BibTeX] [PDF] [Code] [Video]@article{marks2023ral, author = {E. Marks and M. Sodano and F. Magistri and L. Wiesmann and D. Desai and R. Marcuzzi and J. Behley and C. Stachniss}, title = {{High Precision Leaf Instance Segmentation in Point Clouds Obtained Under Real Field Conditions}}, journal = ral, pages = {4791-4798}, volume = {8}, number = {8}, issn = {2377-3766}, year = {2023}, doi = {10.1109/LRA.2023.3288383}, codeurl = {https://github.com/PRBonn/plant_pcd_segmenter}, videourl = {https://youtu.be/dvA1SvQ4iEY} }
- L. Peters, V. Rubies Royo, C. Tomlin, L. Ferranti, J. Alonso-Mora, C. Stachniss, and D. Fridovich-Keil, “Online and Offline Learning of Player Objectives from Partial Observations in Dynamic Games,” The Intl. Journal of Robotics Research, 2023.
[BibTeX] [PDF] [Code] [Video]@article{peters2023ijrr, title = {{Online and Offline Learning of Player Objectives from Partial Observations in Dynamic Games}}, author = {Peters, L. and Rubies Royo, V. and Tomlin, C. and Ferranti, L. and Alonso-Mora, J. and Stachniss, C. and Fridovich-Keil, D.}, journal = ijrr, year = {2023}, url = {https://journals.sagepub.com/doi/reader/10.1177/02783649231182453}, codeurl = {https://github.com/PRBonn/PartiallyObservedInverseGames.jl}, videourl = {https://www.youtube.com/watch?v=BogCsYQX9Pc}, }
- H. Lim, L. Nunes, B. Mersch, X. Chen, J. Behley, H. Myung, and C. Stachniss, “ERASOR2: Instance-Aware Robust 3D Mapping of the Static World in Dynamic Scenes,” in Proc. of Robotics: Science and Systems (RSS), 2023.
[BibTeX] [PDF]@inproceedings{lim2023rss, author = {H. Lim and L. Nunes and B. Mersch and X. Chen and J. Behley and H. Myung and C. Stachniss}, title = {{ERASOR2: Instance-Aware Robust 3D Mapping of the Static World in Dynamic Scenes}}, booktitle = rss, year = 2023, }
- J. Weyler, T. Läbe, F. Magistri, J. Behley, and C. Stachniss, “Towards Domain Generalization in Crop and Weed Segmentation for Precision Farming Robots,” IEEE Robotics and Automation Letters (RA-L), vol. 8, iss. 6, pp. 3310-3317, 2023. doi:10.1109/LRA.2023.3262417
[BibTeX] [PDF] [Code]@article{weyler2023ral, author = {J. Weyler and T. L\"abe and F. Magistri and J. Behley and C. Stachniss}, title = {{Towards Domain Generalization in Crop and Weed Segmentation for Precision Farming Robots}}, journal = ral, pages = {3310-3317}, volume = 8, number = 6, issn = {2377-3766}, year = {2023}, doi = {10.1109/LRA.2023.3262417}, codeurl = {https://github.com/PRBonn/DG-CWS}, }
- L. Nunes, L. Wiesmann, R. Marcuzzi, X. Chen, J. Behley, and C. Stachniss, “Temporal Consistent 3D LiDAR Representation Learning for Semantic Perception in Autonomous Driving,” in Proc. of the IEEE/CVF Conf. on Computer Vision and Pattern Recognition (CVPR), 2023.
[BibTeX] [PDF] [Code] [Video]@inproceedings{nunes2023cvpr, author = {L. Nunes and L. Wiesmann and R. Marcuzzi and X. Chen and J. Behley and C. Stachniss}, title = {{Temporal Consistent 3D LiDAR Representation Learning for Semantic Perception in Autonomous Driving}}, booktitle = cvpr, year = 2023, codeurl = {https://github.com/PRBonn/TARL}, videourl = {https://youtu.be/0CtDbwRYLeo}, }
- H. Kuang, X. Chen, T. Guadagnino, N. Zimmerman, J. Behley, and C. Stachniss, “IR-MCL: Implicit Representation-Based Online Global Localization,” IEEE Robotics and Automation Letters (RA-L), vol. 8, iss. 3, p. 1627–1634, 2023. doi:10.1109/LRA.2023.3239318
[BibTeX] [PDF] [Code]@article{kuang2023ral, author = {Kuang, Haofei and Chen, Xieyuanli and Guadagnino, Tiziano and Zimmerman, Nicky and Behley, Jens and Stachniss, Cyrill}, title = {{IR-MCL: Implicit Representation-Based Online Global Localization}}, journal = ral, volume = {8}, number = {3}, pages = {1627--1634}, doi = {10.1109/LRA.2023.3239318}, year = {2023}, codeurl = {https://github.com/PRBonn/ir-mcl}, }
- X. Zhong, Y. Pan, J. Behley, and C. Stachniss, “SHINE-Mapping: Large-Scale 3D Mapping Using Sparse Hierarchical Implicit Neural Representations,” in Proc. of the IEEE Intl. Conf. on Robotics & Automation (ICRA), 2023.
[BibTeX] [PDF] [Code] [Video]@inproceedings{zhong2023icra, author = {Zhong, Xingguang and Pan, Yue and Behley, Jens and Stachniss, Cyrill}, title = {{SHINE-Mapping: Large-Scale 3D Mapping Using Sparse Hierarchical Implicit Neural Representations}}, booktitle = icra, year = 2023, codeurl = {https://github.com/PRBonn/SHINE_mapping}, videourl = {https://youtu.be/jRqIupJgQZE}, }
- M. Sodano, F. Magistri, T. Guadagnino, J. Behley, and C. Stachniss, “Robust Double-Encoder Network for RGB-D Panoptic Segmentation,” in Proc. of the IEEE Intl. Conf. on Robotics & Automation (ICRA), 2023.
[BibTeX] [PDF] [Code] [Video]@inproceedings{sodano2023icra, author = {Matteo Sodano and Federico Magistri and Tiziano Guadagnino and Jens Behley and Cyrill Stachniss}, title = {{Robust Double-Encoder Network for RGB-D Panoptic Segmentation}}, booktitle = icra, year = 2023, codeurl = {https://github.com/PRBonn/PS-res-excite}, videourl = {https://youtu.be/r1pabV3sQYk} }
- S. Kelly, A. Riccardi, E. Marks, F. Magistri, T. Guadagnino, M. Chli, and C. Stachniss, “Target-Aware Implicit Mapping for Agricultural Crop Inspection,” in Proc. of the IEEE Intl. Conf. on Robotics & Automation (ICRA), 2023.
[BibTeX] [PDF] [Video]@inproceedings{kelly2023icra, author = {Shane Kelly and Alessandro Riccardi and Elias Marks and Federico Magistri and Tiziano Guadagnino and Margarita Chli and Cyrill Stachniss}, title = {{Target-Aware Implicit Mapping for Agricultural Crop Inspection}}, booktitle = icra, year = 2023, videourl = {https://youtu.be/UAIqn0QnpKg} }
- A. Riccardi, S. Kelly, E. Marks, F. Magistri, T. Guadagnino, J. Behley, M. Bennewitz, and C. Stachniss, “Fruit Tracking Over Time Using High-Precision Point Clouds,” in Proc. of the IEEE Intl. Conf. on Robotics & Automation (ICRA), 2023.
[BibTeX] [PDF] [Video]@inproceedings{riccardi2023icra, author = {Alessandro Riccardi and Shane Kelly and Elias Marks and Federico Magistri and Tiziano Guadagnino and Jens Behley and Maren Bennewitz and Cyrill Stachniss}, title = {{Fruit Tracking Over Time Using High-Precision Point Clouds}}, booktitle = icra, year = 2023, videourl = {https://youtu.be/fBGSd0--PXY} }
- G. Roggiolani, M. Sodano, F. Magistri, T. Guadagnino, J. Behley, and C. Stachniss, “Hierarchical Approach for Joint Semantic, Plant Instance, and Leaf Instance Segmentation in the Agricultural Domain,” in Proc. of the IEEE Intl. Conf. on Robotics & Automation (ICRA), 2023.
[BibTeX] [PDF] [Code] [Video]@inproceedings{roggiolani2023icra-hajs, author = {G. Roggiolani and M. Sodano and F. Magistri and T. Guadagnino and J. Behley and C. Stachniss}, title = {{Hierarchical Approach for Joint Semantic, Plant Instance, and Leaf Instance Segmentation in the Agricultural Domain}}, booktitle = icra, year = {2023}, codeurl = {https://github.com/PRBonn/HAPT}, videourl = {https://youtu.be/miuOJjxlJic} }
- G. Roggiolani, F. Magistri, T. Guadagnino, J. Weyler, G. Grisetti, C. Stachniss, and J. Behley, “On Domain-Specific Pre-Training for Effective Semantic Perception in Agricultural Robotics,” in Proc. of the IEEE Intl. Conf. on Robotics & Automation (ICRA), 2023.
[BibTeX] [PDF] [Code] [Video]@inproceedings{roggiolani2023icra-odsp, author = {G. Roggiolani and F. Magistri and T. Guadagnino and J. Weyler and G. Grisetti and C. Stachniss and J. Behley}, title = {{On Domain-Specific Pre-Training for Effective Semantic Perception in Agricultural Robotics}}, booktitle = icra, year = 2023, codeurl= {https://github.com/PRBonn/agri-pretraining}, videourl = {https://youtu.be/FDWY_UnfsBs} }
- H. Dong, X. Chen, M. Dusmanu, V. Larsson, M. Pollefeys, and C. Stachniss, “Learning-Based Dimensionality Reduction for Computing Compact and Effective Local Feature Descriptors,” in Proc. of the IEEE Intl. Conf. on Robotics & Automation (ICRA), 2023.
[BibTeX] [PDF] [Code]@inproceedings{dong2023icra, author = {H. Dong and X. Chen and M. Dusmanu and V. Larsson and M. Pollefeys and C. Stachniss}, title = {{Learning-Based Dimensionality Reduction for Computing Compact and Effective Local Feature Descriptors}}, booktitle = icra, year = 2023, codeurl = {https://github.com/PRBonn/descriptor-dr} }
- M. Zeller, V. S. Sandhu, B. Mersch, J. Behley, M. Heidingsfeld, and C. Stachniss, “Radar Velocity Transformer: Single-scan Moving Object Segmentation in Noisy Radar Point Clouds,” in Proc. of the IEEE Intl. Conf. on Robotics & Automation (ICRA), 2023.
[BibTeX] [PDF] [Video]@inproceedings{zeller2023icra, author = {M. Zeller and V.S. Sandhu and B. Mersch and J. Behley and M. Heidingsfeld and C. Stachniss}, title = {{Radar Velocity Transformer: Single-scan Moving Object Segmentation in Noisy Radar Point Clouds}}, booktitle = icra, year = 2023, videourl = {https://youtu.be/dTDgzWIBgpE} }
- I. Vizzo, T. Guadagnino, B. Mersch, L. Wiesmann, J. Behley, and C. Stachniss, “KISS-ICP: In Defense of Point-to-Point ICP – Simple, Accurate, and Robust Registration If Done the Right Way,” IEEE Robotics and Automation Letters (RA-L), vol. 8, iss. 2, pp. 1-8, 2023. doi:10.1109/LRA.2023.3236571
[BibTeX] [PDF] [Code] [Video]@article{vizzo2023ral, author = {Vizzo, Ignacio and Guadagnino, Tiziano and Mersch, Benedikt and Wiesmann, Louis and Behley, Jens and Stachniss, Cyrill}, title = {{KISS-ICP: In Defense of Point-to-Point ICP -- Simple, Accurate, and Robust Registration If Done the Right Way}}, journal = ral, pages = {1-8}, doi = {10.1109/LRA.2023.3236571}, volume = {8}, number = {2}, year = {2023}, codeurl = {https://github.com/PRBonn/kiss-icp}, videourl = {https://youtu.be/h71aGiD-uxU} }
- R. Marcuzzi, L. Nunes, L. Wiesmann, J. Behley, and C. Stachniss, “Mask-Based Panoptic LiDAR Segmentation for Autonomous Driving,” IEEE Robotics and Automation Letters (RA-L), vol. 8, iss. 2, p. 1141–1148, 2023. doi:10.1109/LRA.2023.3236568
[BibTeX] [PDF] [Code] [Video]@article{marcuzzi2023ral, author = {R. Marcuzzi and L. Nunes and L. Wiesmann and J. Behley and C. Stachniss}, title = {{Mask-Based Panoptic LiDAR Segmentation for Autonomous Driving}}, journal = ral, volume = {8}, number = {2}, pages = {1141--1148}, year = 2023, doi = {10.1109/LRA.2023.3236568}, videourl = {https://youtu.be/I8G9VKpZux8}, codeurl = {https://github.com/PRBonn/MaskPLS}, }
- L. Wiesmann, L. Nunes, J. Behley, and C. Stachniss, “KPPR: Exploiting Momentum Contrast for Point Cloud-Based Place Recognition,” IEEE Robotics and Automation Letters (RA-L), vol. 8, iss. 2, pp. 592-599, 2023. doi:10.1109/LRA.2022.3228174
[BibTeX] [PDF] [Code] [Video]@article{wiesmann2023ral, author = {L. Wiesmann and L. Nunes and J. Behley and C. Stachniss}, title = {{KPPR: Exploiting Momentum Contrast for Point Cloud-Based Place Recognition}}, journal = ral, volume = {8}, number = {2}, pages = {592-599}, year = 2023, issn = {2377-3766}, doi = {10.1109/LRA.2022.3228174}, codeurl = {https://github.com/PRBonn/kppr}, videourl = {https://youtu.be/bICz1sqd8Xs} }
- M. Zeller, J. Behley, M. Heidingsfeld, and C. Stachniss, “Gaussian Radar Transformer for Semantic Segmentation in Noisy Radar Data,” IEEE Robotics and Automation Letters (RA-L), vol. 8, iss. 1, p. 344–351, 2023. doi:10.1109/LRA.2022.3226030
[BibTeX] [PDF] [Video]@article{zeller2023ral, author = {M. Zeller and J. Behley and M. Heidingsfeld and C. Stachniss}, title = {{Gaussian Radar Transformer for Semantic Segmentation in Noisy Radar Data}}, journal = ral, volume = {8}, number = {1}, pages = {344--351}, year = 2023, doi = {10.1109/LRA.2022.3226030}, videourl = {https://youtu.be/uNlNkYoG-tA} }
- N. Zimmerman, T. Guadagnino, X. Chen, J. Behley, and C. Stachniss, “Long-Term Localization using Semantic Cues in Floor Plan Maps,” IEEE Robotics and Automation Letters (RA-L), vol. 8, iss. 1, pp. 176-183, 2023. doi:10.1109/LRA.2022.3223556
[BibTeX] [PDF] [Code]@article{zimmerman2023ral, author = {N. Zimmerman and T. Guadagnino and X. Chen and J. Behley and C. Stachniss}, title = {{Long-Term Localization using Semantic Cues in Floor Plan Maps}}, journal = ral, year = {2023}, volume = {8}, number = {1}, pages = {176-183}, issn = {2377-3766}, doi = {10.1109/LRA.2022.3223556}, codeurl = {https://github.com/PRBonn/hsmcl} }
- H. Müller, N. Zimmerman, T. Polonelli, M. Magno, J. Behley, C. Stachniss, and L. Benini, “Fully On-board Low-Power Localization with Multizone Time-of-Flight Sensors on Nano-UAVs,” in Proc. of Design, Automation & Test in Europe Conference & Exhibition (DATE), 2023.
[BibTeX] [PDF]@inproceedings{mueller2023date, title = {{Fully On-board Low-Power Localization with Multizone Time-of-Flight Sensors on Nano-UAVs}}, author = {H. M{\"u}ller and N. Zimmerman and T. Polonelli and M. Magno and J. Behley and C. Stachniss and L. Benini}, booktitle = {Proc. of Design, Automation \& Test in Europe Conference \& Exhibition (DATE)}, year = {2023}, }
- M. Arora, L. Wiesmann, X. Chen, and C. Stachniss, “Static Map Generation from 3D LiDAR Point Clouds Exploiting Ground Segmentation,” Robotics and Autonomous Systems, vol. 159, p. 104287, 2023. doi:https://doi.org/10.1016/j.robot.2022.104287
[BibTeX] [PDF] [Code]@article{arora2023jras, author = {M. Arora and L. Wiesmann and X. Chen and C. Stachniss}, title = {{Static Map Generation from 3D LiDAR Point Clouds Exploiting Ground Segmentation}}, journal = jras, volume = {159}, pages = {104287}, year = {2023}, issn = {0921-8890}, doi = {https://doi.org/10.1016/j.robot.2022.104287}, codeurl = {https://github.com/PRBonn/dynamic-point-removal}, }
- F. Stache, J. Westheider, F. Magistri, C. Stachniss, and M. Popovic, “Adaptive Path Planning for UAVs for Multi-Resolution Semantic Segmentation,” Robotics and Autonomous Systems, vol. 159, p. 104288, 2023. doi:10.1016/j.robot.2022.104288
[BibTeX] [PDF]@article{stache2023jras, author = {F. Stache and J. Westheider and F. Magistri and C. Stachniss and M. Popovic}, title = {{Adaptive Path Planning for UAVs for Multi-Resolution Semantic Segmentation}}, journal = jras, volume = {159}, pages = {104288}, year = {2023}, issn = {0921-8890}, doi = {10.1016/j.robot.2022.104288}, }
- H. Dong, X. Chen, S. Särkkä, and C. Stachniss, “Online pole segmentation on range images for long-term LiDAR localization in urban environments,” Robotics and Autonomous Systems, vol. 159, p. 104283, 2023. doi:https://doi.org/10.1016/j.robot.2022.104283
[BibTeX] [PDF] [Code]@article{dong2023jras, title = {Online pole segmentation on range images for long-term LiDAR localization in urban environments}, journal = {Robotics and Autonomous Systems}, volume ={159}, pages = {104283}, year = {2023}, issn = {0921-8890}, doi = {https://doi.org/10.1016/j.robot.2022.104283}, author = {H. Dong and X. Chen and S. S{\"a}rkk{\"a} and C. Stachniss}, codeurl = {https://github.com/PRBonn/pole-localization}, url = {https://arxiv.org/pdf/2208.07364.pdf}, }
2022
- L. Di Giammarino, L. Brizi, T. Guadagnino, C. Stachniss, and G. Grisetti, “MD-SLAM: Multi-Cue Direct SLAM,” in Proc. of the IEEE/RSJ Intl. Conf. on Intelligent Robots and Systems (IROS), 2022.
[BibTeX] [PDF] [Code]@inproceedings{digiammarino2022iros, title={{MD-SLAM: Multi-Cue Direct SLAM}}, author={Di Giammarino, L. and Brizi, L. and Guadagnino, T. and Stachniss, C. and Grisetti, G.}, booktitle = iros, year = {2022}, codeurl = {https://github.com/digiamm/md_slam}, url = {https://www.ipb.uni-bonn.de/wp-content/papercite-data/pdf/digiammarino2022iros.pdf}, }
- N. Zimmerman, L. Wiesmann, T. Guadagnino, T. Läbe, J. Behley, and C. Stachniss, “Robust Onboard Localization in Changing Environments Exploiting Text Spotting,” in Proc. of the IEEE/RSJ Intl. Conf. on Intelligent Robots and Systems (IROS), 2022.
[BibTeX] [PDF] [Code]@inproceedings{zimmerman2022iros, title = {{Robust Onboard Localization in Changing Environments Exploiting Text Spotting}}, author = {N. Zimmerman and L. Wiesmann and T. Guadagnino and T. Läbe and J. Behley and C. Stachniss}, booktitle = iros, year = {2022}, codeurl = {https://github.com/PRBonn/tmcl}, }
- Y. Pan, Y. Kompis, L. Bartolomei, R. Mascaro, C. Stachniss, and M. Chli, “Voxfield: Non-Projective Signed Distance Fields for Online Planning and 3D Reconstruction,” in Proc. of the IEEE/RSJ Intl. Conf. on Intelligent Robots and Systems (IROS), 2022.
[BibTeX] [PDF] [Code] [Video]@inproceedings{pan2022iros, title = {{Voxfield: Non-Projective Signed Distance Fields for Online Planning and 3D Reconstruction}}, author = {Y. Pan and Y. Kompis and L. Bartolomei and R. Mascaro and C. Stachniss and M. Chli}, booktitle = iros, year = {2022}, codeurl = {https://github.com/VIS4ROB-lab/voxfield}, videourl ={https://youtu.be/JS_yeq-GR4A}, }
- J. Rückin, L. Jin, F. Magistri, C. Stachniss, and M. Popović, “Informative Path Planning for Active Learning in Aerial Semantic Mapping,” in Proc. of the IEEE/RSJ Intl. Conf. on Intelligent Robots and Systems (IROS), 2022.
[BibTeX] [PDF] [Code]@InProceedings{rueckin2022iros, author = {J. R{\"u}ckin and L. Jin and F. Magistri and C. Stachniss and M. Popovi\'c}, title = {{Informative Path Planning for Active Learning in Aerial Semantic Mapping}}, booktitle = iros, year = {2022}, codeurl = {https://github.com/dmar-bonn/ipp-al}, }
- F. Magistri, E. Marks, S. Nagulavancha, I. Vizzo, T. Läbe, J. Behley, M. Halstead, C. McCool, and C. Stachniss, “Contrastive 3D Shape Completion and Reconstruction for Agricultural Robots using RGB-D Frames,” IEEE Robotics and Automation Letters (RA-L), vol. 7, iss. 4, pp. 10120-10127, 2022.
[BibTeX] [PDF] [Video]@article{magistri2022ral-iros, author = {Federico Magistri and Elias Marks and Sumanth Nagulavancha and Ignacio Vizzo and Thomas L{\"a}be and Jens Behley and Michael Halstead and Chris McCool and Cyrill Stachniss}, title = {Contrastive 3D Shape Completion and Reconstruction for Agricultural Robots using RGB-D Frames}, journal = ral, url = {https://www.ipb.uni-bonn.de/wp-content/papercite-data/pdf/magistri2022ral-iros.pdf}, year = {2022}, volume={7}, number={4}, pages={10120-10127}, videourl = {https://www.youtube.com/watch?v=2ErUf9q7YOI}, }
- Y. Goel, N. Vaskevicius, L. Palmieri, N. Chebrolu, and C. Stachniss, “Predicting Dense and Context-aware Cost Maps for Semantic Robot Navigation,” in IROS Workshop on Perception and Navigation for Autonomous Robotics in Unstructured and Dynamic Environments, 2022.
[BibTeX] [PDF]@inproceedings{goel2022irosws, title = {{Predicting Dense and Context-aware Cost Maps for Semantic Robot Navigation}}, author = {Y. Goel and N. Vaskevicius and L. Palmieri and N. Chebrolu and C. Stachniss}, booktitle = {IROS Workshop on Perception and Navigation for Autonomous Robotics in Unstructured and Dynamic Environments}, year = {2022}, url = {https://www.ipb.uni-bonn.de/wp-content/papercite-data/pdf/goel2022irosws.pdf}, }
- I. Vizzo, B. Mersch, R. Marcuzzi, L. Wiesmann, J. Behley, and C. Stachniss, “Make it Dense: Self-Supervised Geometric Scan Completion of Sparse 3D LiDAR Scans in Large Outdoor Environments,” IEEE Robotics and Automation Letters (RA-L), vol. 7, iss. 3, pp. 8534-8541, 2022. doi:10.1109/LRA.2022.3187255
[BibTeX] [PDF] [Code] [Video]@article{vizzo2022ral, author = {I. Vizzo and B. Mersch and R. Marcuzzi and L. Wiesmann and J. Behley and C. Stachniss}, title = {Make it Dense: Self-Supervised Geometric Scan Completion of Sparse 3D LiDAR Scans in Large Outdoor Environments}, journal = ral, url = {https://www.ipb.uni-bonn.de/wp-content/papercite-data/pdf/vizzo2022ral-iros.pdf}, codeurl = {https://github.com/PRBonn/make_it_dense}, year = {2022}, volume = {7}, number = {3}, pages = {8534-8541}, doi = {10.1109/LRA.2022.3187255}, videourl = {https://youtu.be/NVjURcArHn8}, }
- J. Sun, Y. Wang, M. Feng, D. Wang, J. Zhao, C. Stachniss, and X. Chen, “ICK-Track: A Category-Level 6-DoF Pose Tracker Using Inter-Frame Consistent Keypoints for Aerial Manipulation,” in Proc. of the IEEE/RSJ Intl. Conf. on Intelligent Robots and Systems (IROS), 2022.
[BibTeX] [PDF] [Code]@inproceedings{sun2022iros, title = {{ICK-Track: A Category-Level 6-DoF Pose Tracker Using Inter-Frame Consistent Keypoints for Aerial Manipulation}}, author = {Jingtao Sun and Yaonan Wang and Mingtao Feng and Danwei Wang and Jiawen Zhao and Cyrill Stachniss and Xieyuanli Chen}, booktitle = iros, year = {2022}, codeurl = {https://github.com/S-JingTao/ICK-Track} }
- L. Nunes, X. Chen, R. Marcuzzi, A. Osep, L. Leal-Taixé, C. Stachniss, and J. Behley, “Unsupervised Class-Agnostic Instance Segmentation of 3D LiDAR Data for Autonomous Vehicles,” IEEE Robotics and Automation Letters (RA-L), 2022. doi:10.1109/LRA.2022.3187872
[BibTeX] [PDF] [Code] [Video]@article{nunes2022ral-3duis, author = {Lucas Nunes and Xieyuanli Chen and Rodrigo Marcuzzi and Aljosa Osep and Laura Leal-Taixé and Cyrill Stachniss and Jens Behley}, title = {{Unsupervised Class-Agnostic Instance Segmentation of 3D LiDAR Data for Autonomous Vehicles}}, journal = ral, url = {https://www.ipb.uni-bonn.de/pdfs/nunes2022ral-iros.pdf}, codeurl = {https://github.com/PRBonn/3DUIS}, videourl= {https://youtu.be/cgv0wUaqLAE}, doi = {10.1109/LRA.2022.3187872}, year = 2022 }
- B. Mersch, X. Chen, I. Vizzo, L. Nunes, J. Behley, and C. Stachniss, “Receding Moving Object Segmentation in 3D LiDAR Data Using Sparse 4D Convolutions,” IEEE Robotics and Automation Letters (RA-L), vol. 7, iss. 3, p. 7503–7510, 2022. doi:10.1109/LRA.2022.3183245
[BibTeX] [PDF] [Code] [Video]@article{mersch2022ral, author = {B. Mersch and X. Chen and I. Vizzo and L. Nunes and J. Behley and C. Stachniss}, title = {{Receding Moving Object Segmentation in 3D LiDAR Data Using Sparse 4D Convolutions}}, journal = ral, year = 2022, url = {https://www.ipb.uni-bonn.de/wp-content/papercite-data/pdf/mersch2022ral.pdf}, volume = {7}, number = {3}, pages = {7503--7510}, doi = {10.1109/LRA.2022.3183245}, codeurl = {https://github.com/PRBonn/4DMOS}, videourl = {https://youtu.be/5aWew6caPNQ}, }
- T. Guadagnino, X. Chen, M. Sodano, J. Behley, G. Grisetti, and C. Stachniss, “Fast Sparse LiDAR Odometry Using Self-Supervised Feature Selection on Intensity Images,” IEEE Robotics and Automation Letters (RA-L), vol. 7, iss. 3, pp. 7597-7604, 2022. doi:10.1109/LRA.2022.3184454
[BibTeX] [PDF]@article{guadagnino2022ral, author = {T. Guadagnino and X. Chen and M. Sodano and J. Behley and G. Grisetti and C. Stachniss}, title = {{Fast Sparse LiDAR Odometry Using Self-Supervised Feature Selection on Intensity Images}}, journal = ral, year = 2022, volume = {7}, number = {3}, pages = {7597-7604}, url = {https://www.ipb.uni-bonn.de/wp-content/papercite-data/pdf/guadagnino2022ral-iros.pdf}, issn = {2377-3766}, doi = {10.1109/LRA.2022.3184454} }
- L. Wiesmann, T. Guadagnino, I. Vizzo, G. Grisetti, J. Behley, and C. Stachniss, “DCPCR: Deep Compressed Point Cloud Registration in Large-Scale Outdoor Environments,” IEEE Robotics and Automation Letters (RA-L), vol. 7, iss. 3, pp. 6327-6334, 2022. doi:10.1109/LRA.2022.3171068
[BibTeX] [PDF] [Code] [Video]@article{wiesmann2022ral-iros, author = {L. Wiesmann and T. Guadagnino and I. Vizzo and G. Grisetti and J. Behley and C. Stachniss}, title = {{DCPCR: Deep Compressed Point Cloud Registration in Large-Scale Outdoor Environments}}, journal = ral, year = 2022, volume = 7, number = 3, pages = {6327-6334}, issn = {2377-3766}, doi = {10.1109/LRA.2022.3171068}, codeurl = {https://github.com/PRBonn/DCPCR}, videourl = {https://youtu.be/RqLr2RTGy1s}, }
- L. Peters, D. Fridovich-Keil, L. Ferranti, C. Stachniss, J. Alonso-Mora, and F. Laine, “Learning Mixed Strategies in Trajectory Games,” in Proc. of Robotics: Science and Systems (RSS), 2022.
[BibTeX] [PDF]@inproceedings{peters2022rss, title = {{Learning Mixed Strategies in Trajectory Games}}, author = {L. Peters and D. Fridovich-Keil and L. Ferranti and C. Stachniss and J. Alonso-Mora and F. Laine}, booktitle = rss, year = {2022}, url = {https://arxiv.org/pdf/2205.00291} }
- X. Chen, B. Mersch, L. Nunes, R. Marcuzzi, I. Vizzo, J. Behley, and C. Stachniss, “Automatic Labeling to Generate Training Data for Online LiDAR-Based Moving Object Segmentation,” IEEE Robotics and Automation Letters (RA-L), vol. 7, iss. 3, pp. 6107-6114, 2022. doi:10.1109/LRA.2022.3166544
[BibTeX] [PDF] [Code] [Video]@article{chen2022ral, author = {X. Chen and B. Mersch and L. Nunes and R. Marcuzzi and I. Vizzo and J. Behley and C. Stachniss}, title = {{Automatic Labeling to Generate Training Data for Online LiDAR-Based Moving Object Segmentation}}, journal = ral, year = 2022, volume = 7, number = 3, pages = {6107-6114}, url = {https://arxiv.org/pdf/2201.04501}, issn = {2377-3766}, doi = {10.1109/LRA.2022.3166544}, codeurl = {https://github.com/PRBonn/auto-mos}, videourl = {https://youtu.be/3V5RA1udL4c}, }
- I. Vizzo, T. Guadagnino, J. Behley, and C. Stachniss, “VDBFusion: Flexible and Efficient TSDF Integration of Range Sensor Data,” Sensors, vol. 22, iss. 3, 2022. doi:10.3390/s22031296
[BibTeX] [PDF] [Code]@article{vizzo2022sensors, author = {Vizzo, I. and Guadagnino, T. and Behley, J. and Stachniss, C.}, title = {VDBFusion: Flexible and Efficient TSDF Integration of Range Sensor Data}, journal = {Sensors}, volume = {22}, year = {2022}, number = {3}, article-number = {1296}, url = {https://www.mdpi.com/1424-8220/22/3/1296}, issn = {1424-8220}, doi = {10.3390/s22031296}, codeurl = {https://github.com/PRBonn/vdbfusion}, }
- L. Wiesmann, R. Marcuzzi, C. Stachniss, and J. Behley, “Retriever: Point Cloud Retrieval in Compressed 3D Maps,” in Proc. of the IEEE Intl. Conf. on Robotics & Automation (ICRA), 2022.
[BibTeX] [PDF]@inproceedings{wiesmann2022icra, author = {L. Wiesmann and R. Marcuzzi and C. Stachniss and J. Behley}, title = {{Retriever: Point Cloud Retrieval in Compressed 3D Maps}}, booktitle = icra, year = 2022, }
- E. Marks, F. Magistri, and C. Stachniss, “Precise 3D Reconstruction of Plants from UAV Imagery Combining Bundle Adjustment and Template Matching,” in Proc. of the IEEE Intl. Conf. on Robotics & Automation (ICRA), 2022.
[BibTeX] [PDF]@inproceedings{marks2022icra, author = {E. Marks and F. Magistri and C. Stachniss}, title = {{Precise 3D Reconstruction of Plants from UAV Imagery Combining Bundle Adjustment and Template Matching}}, booktitle = icra, year = 2022, }
- J. Weyler, J. Quakernack, P. Lottes, J. Behley, and C. Stachniss, “Joint Plant and Leaf Instance Segmentation on Field-Scale UAV Imagery,” IEEE Robotics and Automation Letters (RA-L), vol. 7, iss. 2, pp. 3787-3794, 2022. doi:10.1109/LRA.2022.3147462
[BibTeX] [PDF]@article{weyler2022ral, author = {J. Weyler and J. Quakernack and P. Lottes and J. Behley and C. Stachniss}, title = {{Joint Plant and Leaf Instance Segmentation on Field-Scale UAV Imagery}}, journal = ral, year = 2022, doi = {10.1109/LRA.2022.3147462}, issn = {377-3766}, volume = {7}, number = {2}, pages = {3787-3794}, }
- L. Nunes, R. Marcuzzi, X. Chen, J. Behley, and C. Stachniss, “SegContrast: 3D Point Cloud Feature Representation Learning through Self-supervised Segment Discrimination,” IEEE Robotics and Automation Letters (RA-L), vol. 7, iss. 2, pp. 2116-2123, 2022. doi:10.1109/LRA.2022.3142440
[BibTeX] [PDF] [Code] [Video]@article{nunes2022ral, author = {L. Nunes and R. Marcuzzi and X. Chen and J. Behley and C. Stachniss}, title = {{SegContrast: 3D Point Cloud Feature Representation Learning through Self-supervised Segment Discrimination}}, journal = ral, year = 2022, doi = {10.1109/LRA.2022.3142440}, issn = {2377-3766}, volume = {7}, number = {2}, pages = {2116-2123}, url = {https://www.ipb.uni-bonn.de/pdfs/nunes2022ral-icra.pdf}, codeurl = {https://github.com/PRBonn/segcontrast}, videourl = {https://youtu.be/kotRb_ySnIw}, }
- R. Marcuzzi, L. Nunes, L. Wiesmann, I. Vizzo, J. Behley, and C. Stachniss, “Contrastive Instance Association for 4D Panoptic Segmentation using Sequences of 3D LiDAR Scans,” IEEE Robotics and Automation Letters (RA-L), vol. 7, iss. 2, pp. 1550-1557, 2022. doi:10.1109/LRA.2022.3140439
[BibTeX] [PDF]@article{marcuzzi2022ral, author = {R. Marcuzzi and L. Nunes and L. Wiesmann and I. Vizzo and J. Behley and C. Stachniss}, title = {{Contrastive Instance Association for 4D Panoptic Segmentation using Sequences of 3D LiDAR Scans}}, journal = ral, year = 2022, doi = {10.1109/LRA.2022.3140439}, issn = {2377-3766}, volume = 7, number = 2, pages = {1550-1557}, }
- J. Weyler, F. Magistri, P. Seitz, J. Behley, and C. Stachniss, “In-Field Phenotyping Based on Crop Leaf and Plant Instance Segmentation,” in Proc. of the Winter Conf. on Applications of Computer Vision (WACV), 2022.
[BibTeX] [PDF]@inproceedings{weyler2022wacv, author = {J. Weyler and F. Magistri and P. Seitz and J. Behley and C. Stachniss}, title = {{In-Field Phenotyping Based on Crop Leaf and Plant Instance Segmentation}}, booktitle = wacv, year = 2022, }
- S. Li, X. Chen, Y. Liu, D. Dai, C. Stachniss, and J. Gall, “Multi-scale Interaction for Real-time LiDAR Data Segmentation on an Embedded Platform,” IEEE Robotics and Automation Letters (RA-L), vol. 7, iss. 2, pp. 738-745, 2022. doi:10.1109/LRA.2021.3132059
[BibTeX] [PDF] [Code] [Video]@article{li2022ral, author = {S. Li and X. Chen and Y. Liu and D. Dai and C. Stachniss and J. Gall}, title = {{Multi-scale Interaction for Real-time LiDAR Data Segmentation on an Embedded Platform}}, journal = ral, year = 2022, doi = {10.1109/LRA.2021.3132059}, issn = {2377-3766}, volume = 7, number = 2, pages = {738-745}, codeurl = {https://github.com/sj-li/MINet}, videourl = {https://youtu.be/WDhtz5tZ5vQ}, }
2021
- H. Kuang, Y. Zhu, Z. Zhang, X. Li, J. Tighe, S. Schwertfeger, C. Stachniss, and M. Li, “Video Contrastive Learning With Global Context,” in Proc. of the Intl.~Conf. on Computer Vision Workshops, 2021, pp. 3195-3204.
[BibTeX] [PDF] [Code]@inproceedings{kuang2021iccvws, author = {Kuang, Haofei and Zhu, Yi and Zhang, Zhi and Li, Xinyu and Tighe, Joseph and Schwertfeger, S\"oren and Stachniss, Cyrill and Li, Mu}, title = {{Video Contrastive Learning With Global Context}}, booktitle = iccvws, year = {2021}, pages = {3195-3204}, codeurl = {https://github.com/amazon-research/video-contrastive-learning}, url = {https://openaccess.thecvf.com/content/ICCV2021W/CVEU/papers/Kuang_Video_Contrastive_Learning_With_Global_Context_ICCVW_2021_paper.pdf}, }
- A. Barreto, P. Lottes, F. R. Ispizua, S. Baumgarten, N. A. Wolf, C. Stachniss, A. -K. Mahlein, and S. Paulus, “Automatic UAV-based counting of seedlings in sugar-beet field and extension to maize and strawberry,” Computers and Electronics in Agriculture, 2021.
[BibTeX] [PDF]@article{barreto2021cea, author = {A. Barreto and P. Lottes and F.R. Ispizua and S. Baumgarten and N.A. Wolf and C. Stachniss and A.-K. Mahlein and S. Paulus}, title = {Automatic UAV-based counting of seedlings in sugar-beet field and extension to maize and strawberry }, journal = {Computers and Electronics in Agriculture}, year = {2021}, }
- B. Mersch, X. Chen, J. Behley, and C. Stachniss, “Self-supervised Point Cloud Prediction Using 3D Spatio-temporal Convolutional Networks,” in Proc. of the Conf. on Robot Learning (CoRL), 2021.
[BibTeX] [PDF] [Code] [Video]@InProceedings{mersch2021corl, author = {B. Mersch and X. Chen and J. Behley and C. Stachniss}, title = {{Self-supervised Point Cloud Prediction Using 3D Spatio-temporal Convolutional Networks}}, booktitle = corl, year = {2021}, url = {https://www.ipb.uni-bonn.de/pdfs/mersch2021corl.pdf}, codeurl = {https://github.com/PRBonn/point-cloud-prediction}, videourl = {https://youtu.be/-pSZpPgFAso}, }
- J. Behley, M. Garbade, A. Milioto, J. Quenzel, S. Behnke, J. Gall, and C. Stachniss, “Towards 3D LiDAR-based semantic scene understanding of 3D point cloud sequences: The SemanticKITTI Dataset,” The Intl. Journal of Robotics Research, vol. 40, iss. 8-9, pp. 959-967, 2021. doi:10.1177/02783649211006735
[BibTeX] [PDF]@article{behley2021ijrr, author = {J. Behley and M. Garbade and A. Milioto and J. Quenzel and S. Behnke and J. Gall and C. Stachniss}, title = {Towards 3D LiDAR-based semantic scene understanding of 3D point cloud sequences: The SemanticKITTI Dataset}, journal = ijrr, volume = {40}, number = {8-9}, pages = {959-967}, year = {2021}, doi = {10.1177/02783649211006735}, url = {https://www.ipb.uni-bonn.de/pdfs/behley2021ijrr.pdf} }
- A. Pretto, S. Aravecchia, W. Burgard, N. Chebrolu, C. Dornhege, T. Falck, F. Fleckenstein, A. Fontenla, M. Imperoli, R. Khanna, F. Liebisch, P. Lottes, A. Milioto, D. Nardi, S. Nardi, J. Pfeifer, M. Popovic, C. Potena, C. Pradalier, E. Rothacker-Feder, I. Sa, A. Schaefer, R. Siegwart, C. Stachniss, A. Walter, V. Winterhalter, X. Wu, and J. Nieto, “Building an Aerial-Ground Robotics Systemfor Precision Farming: An Adaptable Solution,” IEEE Robotics & Automation Magazine, vol. 28, iss. 3, 2021.
[BibTeX] [PDF]@Article{pretto2021ram, title = {{Building an Aerial-Ground Robotics Systemfor Precision Farming: An Adaptable Solution}}, author = {A. Pretto and S. Aravecchia and W. Burgard and N. Chebrolu and C. Dornhege and T. Falck and F. Fleckenstein and A. Fontenla and M. Imperoli and R. Khanna and F. Liebisch and P. Lottes and A. Milioto and D. Nardi and S. Nardi and J. Pfeifer and M. Popovic and C. Potena and C. Pradalier and E. Rothacker-Feder and I. Sa and A. Schaefer and R. Siegwart and C. Stachniss and A. Walter and V. Winterhalter and X. Wu and J. Nieto}, journal = ram, volume = 28, number = 3, year = {2021}, url={https://www.ipb.uni-bonn.de/pdfs/pretto2021ram.pdf} }
- D. Schunck, F. Magistri, R. A. Rosu, A. Cornelißen, N. Chebrolu, S. Paulus, J. Léon, S. Behnke, C. Stachniss, H. Kuhlmann, and L. Klingbeil, “Pheno4D: A spatio-temporal dataset of maize and tomato plant point clouds for phenotyping and advanced plant analysis ,” PLoS ONE, vol. 16, iss. 8, pp. 1-18, 2021. doi:10.1371/journal.pone.0256340
[BibTeX] [PDF]
Understanding the growth and development of individual plants is of central importance in modern agriculture, crop breeding, and crop science. To this end, using 3D data for plant analysis has gained attention over the last years. High-resolution point clouds offer the potential to derive a variety of plant traits, such as plant height, biomass, as well as the number and size of relevant plant organs. Periodically scanning the plants even allows for performing spatio-temporal growth analysis. However, highly accurate 3D point clouds from plants recorded at different growth stages are rare, and acquiring this kind of data is costly. Besides, advanced plant analysis methods from machine learning require annotated training data and thus generate intense manual labor before being able to perform an analysis. To address these issues, we present with this dataset paper a multi-temporal dataset featuring high-resolution registered point clouds of maize and tomato plants, which we manually labeled for computer vision tasks, such as for instance segmentation and 3D reconstruction, providing approximately 260 million labeled 3D points. To highlight the usability of the data and to provide baselines for other researchers, we show a variety of applications ranging from point cloud segmentation to non-rigid registration and surface reconstruction. We believe that our dataset will help to develop new algorithms to advance the research for plant phenotyping, 3D reconstruction, non-rigid registration, and deep learning on raw point clouds. The dataset is freely accessible at https://www.ipb.uni-bonn.de/data/pheno4d/.
@article{schunck2021plosone, author = {D. Schunck and F. Magistri and R.A. Rosu and A. Corneli{\ss}en and N. Chebrolu and S. Paulus and J. L\'eon and S. Behnke and C. Stachniss and H. Kuhlmann and L. Klingbeil}, title = {{Pheno4D: A spatio-temporal dataset of maize and tomato plant point clouds for phenotyping and advanced plant analysis }}, journal = plosone, year = 2021, url = {https://journals.plos.org/plosone/article/file?id=10.1371/journal.pone.0256340&type=printable}, volume = {16}, number = {8}, doi = {10.1371/journal.pone.0256340}, pages = {1-18}, abstract = {Understanding the growth and development of individual plants is of central importance in modern agriculture, crop breeding, and crop science. To this end, using 3D data for plant analysis has gained attention over the last years. High-resolution point clouds offer the potential to derive a variety of plant traits, such as plant height, biomass, as well as the number and size of relevant plant organs. Periodically scanning the plants even allows for performing spatio-temporal growth analysis. However, highly accurate 3D point clouds from plants recorded at different growth stages are rare, and acquiring this kind of data is costly. Besides, advanced plant analysis methods from machine learning require annotated training data and thus generate intense manual labor before being able to perform an analysis. To address these issues, we present with this dataset paper a multi-temporal dataset featuring high-resolution registered point clouds of maize and tomato plants, which we manually labeled for computer vision tasks, such as for instance segmentation and 3D reconstruction, providing approximately 260 million labeled 3D points. To highlight the usability of the data and to provide baselines for other researchers, we show a variety of applications ranging from point cloud segmentation to non-rigid registration and surface reconstruction. We believe that our dataset will help to develop new algorithms to advance the research for plant phenotyping, 3D reconstruction, non-rigid registration, and deep learning on raw point clouds. The dataset is freely accessible at https://www.ipb.uni-bonn.de/data/pheno4d/.}, }
- F. Stache, J. Westheider, F. Magistri, M. Popović, and C. Stachniss, “Adaptive Path Planning for UAV-based Multi-Resolution Semantic Segmentation,” in Proc. of the European Conf. on Mobile Robots (ECMR), 2021.
[BibTeX] [PDF]@InProceedings{stache2021ecmr, author = {F. Stache and J. Westheider and F. Magistri and M. Popovi\'c and C. Stachniss}, title = {{Adaptive Path Planning for UAV-based Multi-Resolution Semantic Segmentation}}, booktitle = ecmr, year = {2021}, }
- M. Arora, L. Wiesmann, X. Chen, and C. Stachniss, “Mapping the Static Parts of Dynamic Scenes from 3D LiDAR Point Clouds Exploiting Ground Segmentation,” in Proc. of the European Conf. on Mobile Robots (ECMR), 2021.
[BibTeX] [PDF] [Code]@InProceedings{arora2021ecmr, author = {M. Arora and L. Wiesmann and X. Chen and C. Stachniss}, title = {{Mapping the Static Parts of Dynamic Scenes from 3D LiDAR Point Clouds Exploiting Ground Segmentation}}, booktitle = ecmr, codeurl = {https://github.com/humbletechy/Dynamic-Point-Removal}, year = {2021}, }
- H. Dong, X. Chen, and C. Stachniss, “Online Range Image-based Pole Extractor for Long-term LiDAR Localization in Urban Environments,” in Proc. of the European Conf. on Mobile Robots (ECMR), 2021.
[BibTeX] [PDF] [Code]@InProceedings{dong2021ecmr, author = {H. Dong and X. Chen and C. Stachniss}, title = {{Online Range Image-based Pole Extractor for Long-term LiDAR Localization in Urban Environments}}, booktitle = ecmr, year = {2021}, codeurl = {https://github.com/PRBonn/pole-localization}, url = {https://www.ipb.uni-bonn.de/pdfs/dong2021ecmr.pdf} }
- X. Chen, T. Läbe, A. Milioto, T. Röhling, J. Behley, and C. Stachniss, “OverlapNet: A Siamese Network for Computing LiDAR Scan Similarity with Applications to Loop Closing and Localization,” Autonomous Robots, vol. 46, p. 61–81, 2021. doi:10.1007/s10514-021-09999-0
[BibTeX] [PDF] [Code]@article{chen2021auro, author = {X. Chen and T. L\"abe and A. Milioto and T. R\"ohling and J. Behley and C. Stachniss}, title = {{OverlapNet: A Siamese Network for Computing LiDAR Scan Similarity with Applications to Loop Closing and Localization}}, journal = {Autonomous Robots}, year = {2021}, doi = {10.1007/s10514-021-09999-0}, issn = {1573-7527}, volume=46, pages={61--81}, codeurl = {https://github.com/PRBonn/OverlapNet}, url = {https://www.ipb.uni-bonn.de/pdfs/chen2021auro.pdf} }
- L. Di Giammarino, I. Aloise, C. Stachniss, and G. Grisetti, “Visual Place Recognition using LiDAR Intensity Information ,” in Proc. of the IEEE/RSJ Intl. Conf. on Intelligent Robots and Systems (IROS), 2021.
[BibTeX] [PDF]@inproceedings{digiammarino2021iros, title = {{Visual Place Recognition using LiDAR Intensity Information }}, author = {Di Giammarino, L. and I. Aloise and C. Stachniss and G. Grisetti}, booktitle = iros, year = {2021} }
- P. Rottmann, T. Posewsky, A. Milioto, C. Stachniss, and J. Behley, “Improving Monocular Depth Estimation by Semantic Pre-training,” in Proc. of the IEEE/RSJ Intl. Conf. on Intelligent Robots and Systems (IROS), 2021.
[BibTeX] [PDF]@inproceedings{rottmann2021iros, title = {{Improving Monocular Depth Estimation by Semantic Pre-training}}, author = {P. Rottmann and T. Posewsky and A. Milioto and C. Stachniss and J. Behley}, booktitle = iros, year = {2021}, url = {https://www.ipb.uni-bonn.de/pdfs/rottmann2021iros.pdf} }
- B. Mersch, T. Höllen, K. Zhao, C. Stachniss, and R. Roscher, “Maneuver-based Trajectory Prediction for Self-driving Cars Using Spatio-temporal Convolutional Networks,” in Proc. of the IEEE/RSJ Intl. Conf. on Intelligent Robots and Systems (IROS), 2021.
[BibTeX] [PDF] [Video]@inproceedings{mersch2021iros, title = {{Maneuver-based Trajectory Prediction for Self-driving Cars Using Spatio-temporal Convolutional Networks}}, author = {B. Mersch and T. H\"ollen and K. Zhao and C. Stachniss and R. Roscher}, booktitle = iros, year = {2021}, videourl = {https://youtu.be/5RRGWUn4qAw}, url = {https://www.ipb.uni-bonn.de/pdfs/mersch2021iros.pdf} }
- M. Zhou, X. Chen, N. Samano, C. Stachniss, and A. Calway, “Efficient Localisation Using Images and OpenStreetMaps,” in Proc. of the IEEE/RSJ Intl. Conf. on Intelligent Robots and Systems (IROS), 2021.
[BibTeX] [PDF]@inproceedings{zhou2021iros, title = {Efficient Localisation Using Images and OpenStreetMaps}, author = {Zhou, Mengjie and Chen, Xieyuanli and Samano, Noe and Stachniss, Cyrill and Calway, Andrew}, booktitle = iros, year = {2021}, url = {https://www.ipb.uni-bonn.de/pdfs/zhou2021iros.pdf} }
- C. Shi, X. Chen, K. Huang, J. Xiao, H. Lu, and C. Stachniss, “Keypoint Matching for Point Cloud Registration using Multiplex Dynamic Graph Attention Networks,” IEEE Robotics and Automation Letters (RA-L), vol. 6, pp. 8221-8228, 2021. doi:10.1109/LRA.2021.3097275
[BibTeX] [PDF]@article{shi2021ral, title={{Keypoint Matching for Point Cloud Registration using Multiplex Dynamic Graph Attention Networks}}, author={C. Shi and X. Chen and K. Huang and J. Xiao and H. Lu and C. Stachniss}, year={2021}, journal=ral, volume=6, issue=4, pages={8221-8228}, doi = {10.1109/LRA.2021.3097275}, issn = {2377-3766}, }
- X. Chen, S. Li, B. Mersch, L. Wiesmann, J. Gall, J. Behley, and C. Stachniss, “Moving Object Segmentation in 3D LiDAR Data: A Learning-based Approach Exploiting Sequential Data,” IEEE Robotics and Automation Letters (RA-L), vol. 6, pp. 6529-6536, 2021. doi:10.1109/LRA.2021.3093567
[BibTeX] [PDF] [Code] [Video]@article{chen2021ral, title={{Moving Object Segmentation in 3D LiDAR Data: A Learning-based Approach Exploiting Sequential Data}}, author={X. Chen and S. Li and B. Mersch and L. Wiesmann and J. Gall and J. Behley and C. Stachniss}, year={2021}, volume=6, issue=4, pages={6529-6536}, journal=ral, url = {https://www.ipb.uni-bonn.de/pdfs/chen2021ral-iros.pdf}, codeurl = {https://github.com/PRBonn/LiDAR-MOS}, videourl = {https://youtu.be/NHvsYhk4dhw}, doi = {10.1109/LRA.2021.3093567}, issn = {2377-3766}, }
- L. Peters, D. Fridovich-Keil, V. Rubies-Royo, C. J. Tomlin, and C. Stachniss, “Inferring Objectives in Continuous Dynamic Games from Noise-Corrupted Partial State Observations,” in Proc. of Robotics: Science and Systems (RSS), 2021.
[BibTeX] [PDF] [Code] [Video]@inproceedings{peters2021rss, title = {Inferring Objectives in Continuous Dynamic Games from Noise-Corrupted Partial State Observations}, author = {Peters, Lasse and Fridovich-Keil, David and Rubies-Royo, Vicenc and Tomlin, Claire J. and Stachniss, Cyrill}, booktitle = rss, year = {2021}, codeurl = {https://github.com/PRBonn/PartiallyObservedInverseGames.jl}, videourl = {https://www.youtube.com/watch?v=BogCsYQX9Pc}, url = {https://arxiv.org/abs/2106.03611} }
- M. Aygün, A. Osep, M. Weber, M. Maximov, C. Stachniss, J. Behley, and L. Leal-Taixe, “4D Panoptic Segmentation,” in Proc. of the IEEE/CVF Conf. on Computer Vision and Pattern Recognition (CVPR), 2021.
[BibTeX] [PDF]@inproceedings{ayguen2021cvpr, author = {M. Ayg\"un and A. Osep and M. Weber and M. Maximov and C. Stachniss and J. Behley and L. Leal-Taixe}, title = {{4D Panoptic Segmentation}}, booktitle = cvpr, year = 2021, url = {https://www.ipb.uni-bonn.de/pdfs/ayguen2021cvpr.pdf} }
- F. Magistri, N. Chebrolu, J. Behley, and C. Stachniss, “Towards In-Field Phenotyping Exploiting Differentiable Rendering with Self-Consistency Loss,” in Proc. of the IEEE Intl. Conf. on Robotics & Automation (ICRA), 2021.
[BibTeX] [PDF] [Video]@inproceedings{magistri2021icra, author = {F. Magistri and N. Chebrolu and J. Behley and C. Stachniss}, title = {{Towards In-Field Phenotyping Exploiting Differentiable Rendering with Self-Consistency Loss}}, booktitle = icra, year = 2021, videourl = {https://youtu.be/MF2A4ihY2lE}, }
- I. Vizzo, X. Chen, N. Chebrolu, J. Behley, and C. Stachniss, “Poisson Surface Reconstruction for LiDAR Odometry and Mapping,” in Proc. of the IEEE Intl. Conf. on Robotics & Automation (ICRA), 2021.
[BibTeX] [PDF] [Code] [Video]@inproceedings{vizzo2021icra, author = {I. Vizzo and X. Chen and N. Chebrolu and J. Behley and C. Stachniss}, title = {{Poisson Surface Reconstruction for LiDAR Odometry and Mapping}}, booktitle = icra, year = 2021, url = {https://www.ipb.uni-bonn.de/pdfs/vizzo2021icra.pdf}, codeurl = {https://github.com/PRBonn/puma}, videourl = {https://youtu.be/7yWtYWaO5Nk} }
- X. Chen, I. Vizzo, T. Läbe, J. Behley, and C. Stachniss, “Range Image-based LiDAR Localization for Autonomous Vehicles,” in Proc. of the IEEE Intl. Conf. on Robotics & Automation (ICRA), 2021.
[BibTeX] [PDF] [Code] [Video]@inproceedings{chen2021icra, author = {X. Chen and I. Vizzo and T. L{\"a}be and J. Behley and C. Stachniss}, title = {{Range Image-based LiDAR Localization for Autonomous Vehicles}}, booktitle = icra, year = 2021, url = {https://www.ipb.uni-bonn.de/pdfs/chen2021icra.pdf}, codeurl = {https://github.com/PRBonn/range-mcl}, videourl = {https://youtu.be/hpOPXX9oPqI}, }
- A. Reinke, X. Chen, and C. Stachniss, “Simple But Effective Redundant Odometry for Autonomous Vehicles,” in Proc. of the IEEE Intl. Conf. on Robotics & Automation (ICRA), 2021.
[BibTeX] [PDF] [Code] [Video]@inproceedings{reinke2021icra, title={{Simple But Effective Redundant Odometry for Autonomous Vehicles}}, author={A. Reinke and X. Chen and C. Stachniss}, booktitle=icra, year=2021, url = {https://www.ipb.uni-bonn.de/pdfs/reinke2021icra.pdf}, codeurl = {https://github.com/PRBonn/MutiverseOdometry}, videourl = {https://youtu.be/zLpnPEyDKfM} }
- J. Behley, A. Milioto, and C. Stachniss, “A Benchmark for LiDAR-based Panoptic Segmentation based on KITTI,” in Proc. of the IEEE Intl. Conf. on Robotics & Automation (ICRA), 2021.
[BibTeX] [PDF]@inproceedings{behley2021icra, author = {J. Behley and A. Milioto and C. Stachniss}, title = {{A Benchmark for LiDAR-based Panoptic Segmentation based on KITTI}}, booktitle = icra, year = 2021, }
- L. Peters, D. Fridovich-Keil, V. Rubies-Royo, C. J. Tomlin, and C. Stachniss, “Cost Inference in Smooth Dynamic Games from Noise-Corrupted Partial State Observations ,” in Proc. of the RSS Workshop on Social Robot Navigation, 2021.
[BibTeX] [PDF] [Code] [Video]@inproceedings{peters2021rssws, title = {{Cost Inference in Smooth Dynamic Games from Noise-Corrupted Partial State Observations }}, author = {Peters, Lasse and Fridovich-Keil, David and Rubies-Royo, Vicenc and Tomlin, Claire J. and Stachniss, Cyrill}, booktitle = {Proc. of the RSS Workshop on Social Robot Navigation}, year = {2021}, codeurl = {https://github.com/PRBonn/PartiallyObservedInverseGames.jl}, videourl = {https://www.youtube.com/watch?v=BogCsYQX9Pc}, url = {https://socialrobotnavigation.github.io/papers/paper13.pdf} }
- N. Chebrolu, T. Läbe, O. Vysotska, J. Behley, and C. Stachniss, “Adaptive Robust Kernels for Non-Linear Least Squares Problems,” IEEE Robotics and Automation Letters (RA-L), vol. 6, pp. 2240-2247, 2021. doi:10.1109/LRA.2021.3061331
[BibTeX] [PDF] [Video]@article{chebrolu2021ral, author = {N. Chebrolu and T. L\"{a}be and O. Vysotska and J. Behley and C. Stachniss}, title = {{Adaptive Robust Kernels for Non-Linear Least Squares Problems}}, journal = ral, volume = 6, issue = 2, pages = {2240-2247}, doi = {10.1109/LRA.2021.3061331}, year = 2021, videourl = {https://youtu.be/34Zp3ZX0Bnk} }
- J. Weyler, A. Milioto, T. Falck, J. Behley, and C. Stachniss, “Joint Plant Instance Detection and Leaf Count Estimation for In-Field Plant Phenotyping,” IEEE Robotics and Automation Letters (RA-L), vol. 6, pp. 3599-3606, 2021. doi:10.1109/LRA.2021.3060712
[BibTeX] [PDF] [Video]@article{weyler2021ral, author = {J. Weyler and A. Milioto and T. Falck and J. Behley and C. Stachniss}, title = {{Joint Plant Instance Detection and Leaf Count Estimation for In-Field Plant Phenotyping}}, journal = ral, volume = 6, issue = 2, pages = {3599-3606}, doi = {10.1109/LRA.2021.3060712}, year = 2021, videourl = {https://youtu.be/Is18Rey625I}, }
- L. Wiesmann, A. Milioto, X. Chen, C. Stachniss, and J. Behley, “Deep Compression for Dense Point Cloud Maps,” IEEE Robotics and Automation Letters (RA-L), vol. 6, pp. 2060-2067, 2021. doi:10.1109/LRA.2021.3059633
[BibTeX] [PDF] [Code] [Video]@article{wiesmann2021ral, author = {L. Wiesmann and A. Milioto and X. Chen and C. Stachniss and J. Behley}, title = {{Deep Compression for Dense Point Cloud Maps}}, journal = ral, volume = 6, issue = 2, pages = {2060-2067}, doi = {10.1109/LRA.2021.3059633}, year = 2021, url = {https://www.ipb.uni-bonn.de/pdfs/wiesmann2021ral.pdf}, codeurl = {https://github.com/PRBonn/deep-point-map-compression}, videourl = {https://youtu.be/fLl9lTlZrI0} }
- N. Chebrolu, F. Magistri, T. Läbe, and C. Stachniss, “Registration of Spatio-Temporal Point Clouds of Plants for Phenotyping,” PLoS ONE, vol. 16, iss. 2, 2021.
[BibTeX] [PDF] [Video]@article{chebrolu2021plosone, author = {N. Chebrolu and F. Magistri and T. L{\"a}be and C. Stachniss}, title = {{Registration of Spatio-Temporal Point Clouds of Plants for Phenotyping}}, journal = plosone, year = 2021, volume = 16, number = 2, videourl = {https://youtu.be/OV39kb5Nqg8}, }
- F. Görlich, E. Marks, A. Mahlein, K. König, P. Lottes, and C. Stachniss, “UAV-Based Classification of Cercospora Leaf Spot Using RGB Images,” Drones, vol. 5, iss. 2, 2021. doi:10.3390/drones5020034
[BibTeX] [PDF]
Plant diseases can impact crop yield. Thus, the detection of plant diseases using sensors that can be mounted on aerial vehicles is in the interest of farmers to support decision-making in integrated pest management and to breeders for selecting tolerant or resistant genotypes. This paper investigated the detection of Cercospora leaf spot (CLS), caused by Cercospora beticola in sugar beet using RGB imagery. We proposed an approach to tackle the CLS detection problem using fully convolutional neural networks, which operate directly on RGB images captured by a UAV. This efficient approach does not require complex multi- or hyper-spectral sensors, but provides reliable results and high sensitivity. We provided a detection pipeline for pixel-wise semantic segmentation of CLS symptoms, healthy vegetation, and background so that our approach can automatically quantify the grade of infestation. We thoroughly evaluated our system using multiple UAV datasets recorded from different sugar beet trial fields. The dataset consisted of a training and a test dataset and originated from different fields. We used it to evaluate our approach under realistic conditions and analyzed its generalization capabilities to unseen environments. The obtained results correlated to visual estimation by human experts significantly. The presented study underlined the potential of high-resolution RGB imaging and convolutional neural networks for plant disease detection under field conditions. The demonstrated procedure is particularly interesting for applications under practical conditions, as no complex and cost-intensive measuring system is required.
@Article{goerlich2021drones, AUTHOR = {Görlich, Florian and Marks, Elias and Mahlein, Anne-Katrin and König, Kathrin and Lottes, Philipp and Stachniss, Cyrill}, TITLE = {{UAV-Based Classification of Cercospora Leaf Spot Using RGB Images}}, JOURNAL = {Drones}, VOLUME = {5}, YEAR = {2021}, NUMBER = {2}, ARTICLE-NUMBER = {34}, URL = {https://www.mdpi.com/2504-446X/5/2/34/pdf}, ISSN = {2504-446X}, ABSTRACT = {Plant diseases can impact crop yield. Thus, the detection of plant diseases using sensors that can be mounted on aerial vehicles is in the interest of farmers to support decision-making in integrated pest management and to breeders for selecting tolerant or resistant genotypes. This paper investigated the detection of Cercospora leaf spot (CLS), caused by Cercospora beticola in sugar beet using RGB imagery. We proposed an approach to tackle the CLS detection problem using fully convolutional neural networks, which operate directly on RGB images captured by a UAV. This efficient approach does not require complex multi- or hyper-spectral sensors, but provides reliable results and high sensitivity. We provided a detection pipeline for pixel-wise semantic segmentation of CLS symptoms, healthy vegetation, and background so that our approach can automatically quantify the grade of infestation. We thoroughly evaluated our system using multiple UAV datasets recorded from different sugar beet trial fields. The dataset consisted of a training and a test dataset and originated from different fields. We used it to evaluate our approach under realistic conditions and analyzed its generalization capabilities to unseen environments. The obtained results correlated to visual estimation by human experts significantly. The presented study underlined the potential of high-resolution RGB imaging and convolutional neural networks for plant disease detection under field conditions. The demonstrated procedure is particularly interesting for applications under practical conditions, as no complex and cost-intensive measuring system is required.}, DOI = {10.3390/drones5020034} }
- C. Carbone, D. Albani, F. Magistri, D. Ognibene, C. Stachniss, G. Kootstra, D. Nardi, and V. Trianni, “Monitoring and Mapping of Crop Fields with UAV Swarms Based on Information Gain,” in Proc. of the Intl. Symp. on Distributed Autonomous Robotic Systems (DARS), 2021.
[BibTeX] [PDF]@inproceedings{carbone2021dars, author = {C. Carbone and D. Albani and F. Magistri and D. Ognibene and C. Stachniss and G. Kootstra and D. Nardi and V. Trianni}, title = {{Monitoring and Mapping of Crop Fields with UAV Swarms Based on Information Gain}}, booktitle = dars, year = 2021, }
- C. Stachniss, “Achievements Needed for Becoming a Professor,” Academia Letters, iss. 281, 2021. doi:https://doi.org/10.20935/AL281
[BibTeX] [PDF] [Video]
What is needed to become a professor? This article summarizes what selection committees often regard as the minimum achievements when recruiting new professors. My goal is to give early-career researchers a brief guideline on their way towards becoming a faculty member.
@article{stachniss2021al, author = {C. Stachniss}, title = {{Achievements Needed for Becoming a Professor}}, year = {2021}, journal = {Academia Letters}, number = {281}, doi = {https://doi.org/10.20935/AL281}, url = {https://www.ipb.uni-bonn.de/pdfs/stachniss2021al.pdf}, abstract = {What is needed to become a professor? This article summarizes what selection committees often regard as the minimum achievements when recruiting new professors. My goal is to give early-career researchers a brief guideline on their way towards becoming a faculty member.}, videourl = {https://youtu.be/223cMIgN5p0} }
2020
- C. Stachniss, I. Vizzo, L. Wiesmann, and N. Berning, How To Setup and Run a 100\% Digital Conf.: DIGICROP 2020, 2020.
[BibTeX] [PDF]
The purpose of this record is to document the setup and execution of DIGICROP 2020 and to simplify conducting future online events of that kind. DIGICROP 2020 was a 100\% virtual conference run via Zoom with around 900 registered people in November 2020. It consisted of video presentations available via our website and a single-day live event for Q&A. We had around 450 people attending the Q&A session overall, most of the time 200-250 people have been online at the same time. This document is a collection of notes, instructions, and todo lists. It is not a polished manual, however, we believe these notes will be useful for other conference organizers and for us in the future.
@misc{stachniss2020digitalconf, author = {C. Stachniss and I. Vizzo and L. Wiesmann and N. Berning}, title = {{How To Setup and Run a 100\% Digital Conf.: DIGICROP 2020}}, year = {2020}, url = {https://www.ipb.uni-bonn.de/pdfs/stachniss2020digitalconf.pdf}, abstract = {The purpose of this record is to document the setup and execution of DIGICROP 2020 and to simplify conducting future online events of that kind. DIGICROP 2020 was a 100\% virtual conference run via Zoom with around 900 registered people in November 2020. It consisted of video presentations available via our website and a single-day live event for Q&A. We had around 450 people attending the Q&A session overall, most of the time 200-250 people have been online at the same time. This document is a collection of notes, instructions, and todo lists. It is not a polished manual, however, we believe these notes will be useful for other conference organizers and for us in the future.}, }
- A. Milioto, J. Behley, C. McCool, and C. Stachniss, “LiDAR Panoptic Segmentation for Autonomous Driving,” in Proc. of the IEEE/RSJ Intl. Conf. on Intelligent Robots and Systems (IROS), 2020.
[BibTeX] [PDF] [Video]@inproceedings{milioto2020iros, author = {A. Milioto and J. Behley and C. McCool and C. Stachniss}, title = {{LiDAR Panoptic Segmentation for Autonomous Driving}}, booktitle = iros, year = {2020}, videourl = {https://www.youtube.com/watch?v=C9CTQSosr9I}, }
- X. Chen, T. Läbe, L. Nardi, J. Behley, and C. Stachniss, “Learning an Overlap-based Observation Model for 3D LiDAR Localization,” in Proc. of the IEEE/RSJ Intl. Conf. on Intelligent Robots and Systems (IROS), 2020.
[BibTeX] [PDF] [Code] [Video]@inproceedings{chen2020iros, author = {X. Chen and T. L\"abe and L. Nardi and J. Behley and C. Stachniss}, title = {{Learning an Overlap-based Observation Model for 3D LiDAR Localization}}, booktitle = iros, year = {2020}, codeurl = {https://github.com/PRBonn/overlap_localization}, url={https://www.ipb.uni-bonn.de/pdfs/chen2020iros.pdf}, videourl = {https://www.youtube.com/watch?v=BozPqy_6YcE}, }
- F. Langer, A. Milioto, A. Haag, J. Behley, and C. Stachniss, “Domain Transfer for Semantic Segmentation of LiDAR Data using Deep Neural Networks,” in Proc. of the IEEE/RSJ Intl. Conf. on Intelligent Robots and Systems (IROS), 2020.
[BibTeX] [PDF] [Code] [Video]@inproceedings{langer2020iros, author = {F. Langer and A. Milioto and A. Haag and J. Behley and C. Stachniss}, title = {{Domain Transfer for Semantic Segmentation of LiDAR Data using Deep Neural Networks}}, booktitle = iros, year = {2020}, url = {https://www.ipb.uni-bonn.de/pdfs/langer2020iros.pdf}, videourl = {https://youtu.be/6FNGF4hKBD0}, codeurl = {https://github.com/PRBonn/lidar_transfer}, }
- F. Magistri, N. Chebrolu, and C. Stachniss, “Segmentation-Based 4D Registration of Plants Point Clouds for Phenotyping,” in Proc. of the IEEE/RSJ Intl. Conf. on Intelligent Robots and Systems (IROS), 2020.
[BibTeX] [PDF] [Video]@inproceedings{magistri2020iros, author = {F. Magistri and N. Chebrolu and C. Stachniss}, title = {{Segmentation-Based 4D Registration of Plants Point Clouds for Phenotyping}}, booktitle = iros, year = {2020}, url={https://www.ipb.uni-bonn.de/pdfs/magistri2020iros.pdf}, videourl = {https://youtu.be/OV39kb5Nqg8}, }
- D. Gogoll, P. Lottes, J. Weyler, N. Petrinic, and C. Stachniss, “Unsupervised Domain Adaptation for Transferring Plant Classification Systems to New Field Environments, Crops, and Robots,” in Proc. of the IEEE/RSJ Intl. Conf. on Intelligent Robots and Systems (IROS), 2020.
[BibTeX] [PDF] [Video]@inproceedings{gogoll2020iros, author = {D. Gogoll and P. Lottes and J. Weyler and N. Petrinic and C. Stachniss}, title = {{Unsupervised Domain Adaptation for Transferring Plant Classification Systems to New Field Environments, Crops, and Robots}}, booktitle = iros, year = {2020}, url = {https://www.ipb.uni-bonn.de/pdfs/gogoll2020iros.pdf}, videourl = {https://www.youtube.com/watch?v=6K79Ih6KXTs}, }
- X. Chen, T. Läbe, A. Milioto, T. Röhling, O. Vysotska, A. Haag, J. Behley, and C. Stachniss, “OverlapNet: Loop Closing for LiDAR-based SLAM,” in Proc. of Robotics: Science and Systems (RSS), 2020.
[BibTeX] [PDF] [Code] [Video]@inproceedings{chen2020rss, author = {X. Chen and T. L\"abe and A. Milioto and T. R\"ohling and O. Vysotska and A. Haag and J. Behley and C. Stachniss}, title = {{OverlapNet: Loop Closing for LiDAR-based SLAM}}, booktitle = rss, year = {2020}, codeurl = {https://github.com/PRBonn/OverlapNet/}, videourl = {https://youtu.be/YTfliBco6aw}, }
- N. Chebrolu, T. Laebe, O. Vysotska, J. Behley, and C. Stachniss, “Adaptive Robust Kernels for Non-Linear Least Squares Problems,” arXiv Preprint, 2020.
[BibTeX] [PDF]@article{chebrolu2020arxiv, title={Adaptive Robust Kernels for Non-Linear Least Squares Problems}, author={N. Chebrolu and T. Laebe and O. Vysotska and J. Behley and C. Stachniss}, journal = arxiv, year=2020, eprint={2004.14938}, keywords={cs.RO}, url={https://arxiv.org/pdf/2004.14938v2} }
- J. Behley, A. Milioto, and C. Stachniss, “A Benchmark for LiDAR-based Panoptic Segmentation based on KITTI,” arXiv Preprint, 2020.
[BibTeX] [PDF]
Panoptic segmentation is the recently introduced task that tackles semantic segmentation and instance segmentation jointly. In this paper, we present an extension of SemanticKITTI, which is a large-scale dataset providing dense point-wise semantic labels for all sequences of the KITTI Odometry Benchmark, for training and evaluation of laser-based panoptic segmentation. We provide the data and discuss the processing steps needed to enrich a given semantic annotation with temporally consistent instance information, i.e., instance information that supplements the semantic labels and identifies the same instance over sequences of LiDAR point clouds. Additionally, we present two strong baselines that combine state-of-the-art LiDAR-based semantic segmentation approaches with a state-of-the-art detector enriching the segmentation with instance information and that allow other researchers to compare their approaches against. We hope that our extension of SemanticKITTI with strong baselines enables the creation of novel algorithms for LiDAR-based panoptic segmentation as much as it has for the original semantic segmentation and semantic scene completion tasks. Data, code, and an online evaluation using a hidden test set will be published on https://semantic-kitti.org.
@article{behley2020arxiv, author = {J. Behley and A. Milioto and C. Stachniss}, title = {{A Benchmark for LiDAR-based Panoptic Segmentation based on KITTI}}, journal = arxiv, year = 2020, eprint = {2003.02371v1}, url = {https://arxiv.org/pdf/2003.02371v1}, keywords = {cs.CV}, abstract = {Panoptic segmentation is the recently introduced task that tackles semantic segmentation and instance segmentation jointly. In this paper, we present an extension of SemanticKITTI, which is a large-scale dataset providing dense point-wise semantic labels for all sequences of the KITTI Odometry Benchmark, for training and evaluation of laser-based panoptic segmentation. We provide the data and discuss the processing steps needed to enrich a given semantic annotation with temporally consistent instance information, i.e., instance information that supplements the semantic labels and identifies the same instance over sequences of LiDAR point clouds. Additionally, we present two strong baselines that combine state-of-the-art LiDAR-based semantic segmentation approaches with a state-of-the-art detector enriching the segmentation with instance information and that allow other researchers to compare their approaches against. We hope that our extension of SemanticKITTI with strong baselines enables the creation of novel algorithms for LiDAR-based panoptic segmentation as much as it has for the original semantic segmentation and semantic scene completion tasks. Data, code, and an online evaluation using a hidden test set will be published on https://semantic-kitti.org.} }
- X. Wu, S. Aravecchia, P. Lottes, C. Stachniss, and C. Pradalier, “Robotic Weed Control Using Automated Weed and Crop Classification,” Journal of Field Robotics, vol. 37, pp. 322-340, 2020.
[BibTeX] [PDF]@Article{wu2020jfr, title = {Robotic Weed Control Using Automated Weed and Crop Classification}, author = {X. Wu and S. Aravecchia and P. Lottes and C. Stachniss and C. Pradalier}, journal = jfr, year = {2020}, volume = {37}, numer = {2}, pages = {322-340}, url = {https://www.ipb.uni-bonn.de/pdfs/wu2020jfr.pdf}, }
- P. Lottes, J. Behley, N. Chebrolu, A. Milioto, and C. Stachniss, “Robust joint stem detection and crop-weed classification using image sequences for plant-specific treatment in precision farming,” Journal of Field Robotics, vol. 37, pp. 20-34, 2020. doi:https://doi.org/10.1002/rob.21901
[BibTeX] [PDF]@Article{lottes2020jfr, title = {Robust joint stem detection and crop-weed classification using image sequences for plant-specific treatment in precision farming}, author = {Lottes, P. and Behley, J. and Chebrolu, N. and Milioto, A. and Stachniss, C.}, journal = jfr, volume = {37}, numer = {1}, pages = {20-34}, year = {2020}, doi = {https://doi.org/10.1002/rob.21901}, url = {https://www.ipb.uni-bonn.de/pdfs/lottes2019jfr.pdf}, }
- N. Chebrolu, T. Laebe, and C. Stachniss, “Spatio-Temporal Non-Rigid Registration of 3D Point Clouds of Plants,” in Proc. of the IEEE Intl. Conf. on Robotics & Automation (ICRA), 2020.
[BibTeX] [PDF] [Video]@InProceedings{chebrolu2020icra, title = {Spatio-Temporal Non-Rigid Registration of 3D Point Clouds of Plants}, author = {N. Chebrolu and T. Laebe and C. Stachniss}, booktitle = icra, year = {2020}, url = {https://www.ipb.uni-bonn.de/pdfs/chebrolu2020icra.pdf}, videourl = {https://www.youtube.com/watch?v=uGkep_aelBc}, }
- A. Ahmadi, L. Nardi, N. Chebrolu, and C. Stachniss, “Visual Servoing-based Navigation for Monitoring Row-Crop Fields,” in Proc. of the IEEE Intl. Conf. on Robotics & Automation (ICRA), 2020.
[BibTeX] [PDF] [Code] [Video]@InProceedings{ahmadi2020icra, title = {Visual Servoing-based Navigation for Monitoring Row-Crop Fields}, author = {A. Ahmadi and L. Nardi and N. Chebrolu and C. Stachniss}, booktitle = icra, year = {2020}, url = {https://arxiv.org/pdf/1909.12754}, codeurl = {https://github.com/PRBonn/visual-crop-row-navigation}, videourl = {https://youtu.be/0qg6n4sshHk}, }
- L. Nardi and C. Stachniss, “Long-Term Robot Navigation in Indoor Environments Estimating Patterns in Traversability Changes,” in Proc. of the IEEE Intl. Conf. on Robotics & Automation (ICRA), 2020.
[BibTeX] [PDF] [Video]@InProceedings{nardi2020icra, title = {Long-Term Robot Navigation in Indoor Environments Estimating Patterns in Traversability Changes}, author = {L. Nardi and C. Stachniss}, booktitle = icra, year = {2020}, url = {https://arxiv.org/pdf/1909.12733}, videourl = {https://www.youtube.com/watch?v=9lNcA3quzwU}, }
- R. Sheikh, A. Milioto, P. Lottes, C. Stachniss, M. Bennewitz, and T. Schultz, “Gradient and Log-based Active Learning for Semantic Segmentation of Crop and Weed for Agricultural Robots,” in Proc. of the IEEE Intl. Conf. on Robotics & Automation (ICRA), 2020.
[BibTeX] [PDF] [Video]@InProceedings{sheikh2020icra, title = {Gradient and Log-based Active Learning for Semantic Segmentation of Crop and Weed for Agricultural Robots}, author = {R. Sheikh and A. Milioto and P. Lottes and C. Stachniss and M. Bennewitz and T. Schultz}, booktitle = icra, year = {2020}, url = {https://www.ipb.uni-bonn.de/pdfs/sheikh2020icra.pdf}, videourl = {https://www.youtube.com/watch?v=NySa59gxFAg}, }
- J. Quenzel, R. A. Rosu, T. Laebe, C. Stachniss, and S. Behnke, “Beyond Photometric Consistency: Gradient-based Dissimilarity for Improving Visual Odometry and Stereo Matching,” in Proc. of the IEEE Intl. Conf. on Robotics & Automation (ICRA), 2020.
[BibTeX] [PDF] [Video]@InProceedings{quenzel020icra, title = {Beyond Photometric Consistency: Gradient-based Dissimilarity for Improving Visual Odometry and Stereo Matching}, author = {J. Quenzel and R.A. Rosu and T. Laebe and C. Stachniss and S. Behnke}, booktitle = icra, year = {2020}, url = {https://www.ipb.uni-bonn.de/pdfs/quenzel2020icra.pdf}, videourl = {https://www.youtube.com/watch?v=cqv7k-BK0g0}, }
- P. Regier, A. Milioto, C. Stachniss, and M. Bennewitz, “Classifying Obstacles and Exploiting Class Information for Humanoid Navigation Through Cluttered Environments,” The Intl. Journal of Humanoid Robotics (IJHR), vol. 17, iss. 02, p. 2050013, 2020. doi:10.1142/S0219843620500139
[BibTeX] [PDF]
Humanoid robots are often supposed to share their workspace with humans and thus have to deal with objects used by humans in their everyday life. In this article, we present our novel approach to humanoid navigation through cluttered environments, which exploits knowledge about different obstacle classes to decide how to deal with obstacles and select appropriate robot actions. To classify objects from RGB images and decide whether an obstacle can be overcome by the robot with a corresponding action, e.g., by pushing or carrying it aside or stepping over or onto it, we train and exploit a convolutional neural network (CNN). Based on associated action costs, we compute a cost grid containing newly observed objects in addition to static obstacles on which a 2D path can be efficiently planned. This path encodes the necessary actions that need to be carried out by the robot to reach the goal. We implemented our framework in the Robot Operating System (ROS) and tested it in various scenarios with a Nao robot as well as in simulation with the REEM-C robot. As the experiments demonstrate, using our CNN, the robot can robustly classify the observed obstacles into the different classes and decide on suitable actions to find efficient solution paths. Our system finds paths also through regions where traditional motion planning methods are not able to calculate a solution or require substantially more time.
@article{regier2020ijhr, author = {Regier, P. and Milioto, A. and Stachniss, C. and Bennewitz, M.}, title = {{Classifying Obstacles and Exploiting Class Information for Humanoid Navigation Through Cluttered Environments}}, journal = ijhr, volume = {17}, number = {02}, pages = {2050013}, year = {2020}, doi = {10.1142/S0219843620500139}, abstract = {Humanoid robots are often supposed to share their workspace with humans and thus have to deal with objects used by humans in their everyday life. In this article, we present our novel approach to humanoid navigation through cluttered environments, which exploits knowledge about different obstacle classes to decide how to deal with obstacles and select appropriate robot actions. To classify objects from RGB images and decide whether an obstacle can be overcome by the robot with a corresponding action, e.g., by pushing or carrying it aside or stepping over or onto it, we train and exploit a convolutional neural network (CNN). Based on associated action costs, we compute a cost grid containing newly observed objects in addition to static obstacles on which a 2D path can be efficiently planned. This path encodes the necessary actions that need to be carried out by the robot to reach the goal. We implemented our framework in the Robot Operating System (ROS) and tested it in various scenarios with a Nao robot as well as in simulation with the REEM-C robot. As the experiments demonstrate, using our CNN, the robot can robustly classify the observed obstacles into the different classes and decide on suitable actions to find efficient solution paths. Our system finds paths also through regions where traditional motion planning methods are not able to calculate a solution or require substantially more time. } }
2019
- J. Behley, M. Garbade, A. Milioto, J. Quenzel, S. Behnke, C. Stachniss, and J. Gall, “SemanticKITTI: A Dataset for Semantic Scene Understanding of LiDAR Sequences,” in Proc. of the IEEE/CVF Intl. Conf. on Computer Vision (ICCV), 2019.
[BibTeX] [PDF] [Video]@InProceedings{behley2019iccv, author = {J. Behley and M. Garbade and A. Milioto and J. Quenzel and S. Behnke and C. Stachniss and J. Gall}, title = {{SemanticKITTI: A Dataset for Semantic Scene Understanding of LiDAR Sequences}}, booktitle = iccv, year = {2019}, videourl = {https://www.ipb.uni-bonn.de/html/projects/semantic_kitti/videos/teaser.mp4}, }
- A. Pretto, S. Aravecchia, W. Burgard, N. Chebrolu, C. Dornhege, T. Falck, F. Fleckenstein, A. Fontenla, M. Imperoli, R. Khanna, F. Liebisch, P. Lottes, A. Milioto, D. Nardi, S. Nardi, J. Pfeifer, M. Popović, C. Potena, C. Pradalier, E. Rothacker-Feder, I. Sa, A. Schaefer, R. Siegwart, C. Stachniss, A. Walter, W. Winterhalter, X. Wu, and J. Nieto, “Building an Aerial-Ground Robotics System for Precision Farming,” arXiv Preprint, 2019.
[BibTeX] [PDF]@article{pretto2019arxiv, author = {A. Pretto and S. Aravecchia and W. Burgard and N. Chebrolu and C. Dornhege and T. Falck and F. Fleckenstein and A. Fontenla and M. Imperoli and R. Khanna and F. Liebisch and P. Lottes and A. Milioto and D. Nardi and S. Nardi and J. Pfeifer and M. Popović and C. Potena and C. Pradalier and E. Rothacker-Feder and I. Sa and A. Schaefer and R. Siegwart and C. Stachniss and A. Walter and W. Winterhalter and X. Wu and J. Nieto}, title = {{Building an Aerial-Ground Robotics System for Precision Farming}}, journal = arxiv, year = 2019, eprint = {1911.03098v1}, url = {https://arxiv.org/pdf/1911.03098v1}, keywords = {cs.RO}, }
- O. Vysotska and C. Stachniss, “Effective Visual Place Recognition Using Multi-Sequence Maps,” IEEE Robotics and Automation Letters (RA-L), vol. 4, pp. 1730-1736, 2019.
[BibTeX] [PDF] [Video]@article{vysotska2019ral, author = {O. Vysotska and C. Stachniss}, title = {{Effective Visual Place Recognition Using Multi-Sequence Maps}}, journal = ral, year = 2019, volume = 4, issue = 2, pages = {1730-1736}, url = {https://www.ipb.uni-bonn.de/pdfs/vysotska2019ral.pdf}, videourl = {https://youtu.be/wFU0JoXTH3c}, }
- E. Palazzolo, J. Behley, P. Lottes, P. Giguère, and C. Stachniss, “ReFusion: 3D Reconstruction in Dynamic Environments for RGB-D Cameras Exploiting Residuals,” in Proc. of the IEEE/RSJ Intl. Conf. on Intelligent Robots and Systems (IROS), 2019.
[BibTeX] [PDF] [Code] [Video]@InProceedings{palazzolo2019iros, author = {E. Palazzolo and J. Behley and P. Lottes and P. Gigu\`ere and C. Stachniss}, title = {{ReFusion: 3D Reconstruction in Dynamic Environments for RGB-D Cameras Exploiting Residuals}}, booktitle = iros, year = {2019}, url = {https://www.ipb.uni-bonn.de/pdfs/palazzolo2019iros.pdf}, codeurl = {https://github.com/PRBonn/refusion}, videourl = {https://youtu.be/1P9ZfIS5-p4}, }
- X. Chen, A. Milioto, E. Palazzolo, P. Giguère, J. Behley, and C. Stachniss, “SuMa++: Efficient LiDAR-based Semantic SLAM,” in Proc. of the IEEE/RSJ Intl. Conf. on Intelligent Robots and Systems (IROS), 2019.
[BibTeX] [PDF] [Code] [Video]@inproceedings{chen2019iros, author = {X. Chen and A. Milioto and E. Palazzolo and P. Giguère and J. Behley and C. Stachniss}, title = {{SuMa++: Efficient LiDAR-based Semantic SLAM}}, booktitle = iros, year = 2019, codeurl = {https://github.com/PRBonn/semantic_suma/}, videourl = {https://youtu.be/uo3ZuLuFAzk}, }
- A. Milioto, I. Vizzo, J. Behley, and C. Stachniss, “RangeNet++: Fast and Accurate LiDAR Semantic Segmentation,” in Proc. of the IEEE/RSJ Intl. Conf. on Intelligent Robots and Systems (IROS), 2019.
[BibTeX] [PDF] [Code] [Video]@inproceedings{milioto2019iros, author = {A. Milioto and I. Vizzo and J. Behley and C. Stachniss}, title = {{RangeNet++: Fast and Accurate LiDAR Semantic Segmentation}}, booktitle = iros, year = 2019, codeurl = {https://github.com/PRBonn/lidar-bonnetal}, videourl = {https://youtu.be/wuokg7MFZyU}, }
- F. Yan, O. Vysotska, and C. Stachniss, ” Global Localization on OpenStreetMap Using 4-bit Semantic Descriptors,” in Proc. of the European Conf. on Mobile Robots (ECMR), 2019.
[BibTeX] [PDF]@InProceedings{yan2019ecmr, author = {F. Yan and O. Vysotska and C. Stachniss}, title = {{ Global Localization on OpenStreetMap Using 4-bit Semantic Descriptors}}, booktitle = ecmr, year = {2019}, }
- O. Vysotska, H. Kuhlmann, and C. Stachniss, “UAVs Towards Sustainable Crop Production,” in Workshop at Robotics: Science and Systems, 2019.
[BibTeX] [PDF]@InProceedings{vysotska2019rsswsabstract, author = {O. Vysotska and H. Kuhlmann and C. Stachniss}, title = {{UAVs Towards Sustainable Crop Production}}, booktitle = {Workshop at Robotics: Science and Systems}, year = {2019}, note = {Abstract}, }
- A. Milioto and C. Stachniss, “Bonnet: An Open-Source Training and Deployment Framework for Semantic Segmentation in Robotics using CNNs,” in Proc. of the IEEE Intl. Conf. on Robotics & Automation (ICRA), 2019.
[BibTeX] [PDF] [Code] [Video]@InProceedings{milioto2019icra, author = {A. Milioto and C. Stachniss}, title = {{Bonnet: An Open-Source Training and Deployment Framework for Semantic Segmentation in Robotics using CNNs}}, booktitle = icra, year = 2019, codeurl = {https://github.com/Photogrammetry-Robotics-Bonn/bonnet}, videourl = {https://www.youtube.com/watch?v=tfeFHCq6YJs}, }
- A. Milioto, L. Mandtler, and C. Stachniss, “Fast Instance and Semantic Segmentation Exploiting Local Connectivity, Metric Learning, and One-Shot Detection for Robotics ,” in Proc. of the IEEE Intl. Conf. on Robotics & Automation (ICRA), 2019.
[BibTeX] [PDF]@InProceedings{milioto2019icra-fiass, author = {A. Milioto and L. Mandtler and C. Stachniss}, title = {{Fast Instance and Semantic Segmentation Exploiting Local Connectivity, Metric Learning, and One-Shot Detection for Robotics }}, booktitle = icra, year = 2019, }
- L. Nardi and C. Stachniss, “Uncertainty-Aware Path Planning for Navigation on Road Networks Using Augmented MDPs ,” in Proc. of the IEEE Intl. Conf. on Robotics & Automation (ICRA), 2019.
[BibTeX] [PDF] [Video]@InProceedings{nardi2019icra-uapp, author = {L. Nardi and C. Stachniss}, title = {{Uncertainty-Aware Path Planning for Navigation on Road Networks Using Augmented MDPs }}, booktitle = icra, year = 2019, url={https://www.ipb.uni-bonn.de/pdfs/nardi2019icra-uapp.pdf}, videourl = {https://youtu.be/3PMSamgYzi4}, }
- L. Nardi and C. Stachniss, “Actively Improving Robot Navigation On Different Terrains Using Gaussian Process Mixture Models,” in Proc. of the IEEE Intl. Conf. on Robotics & Automation (ICRA), 2019.
[BibTeX] [PDF] [Video]@InProceedings{nardi2019icra-airn, author = {L. Nardi and C. Stachniss}, title = {{Actively Improving Robot Navigation On Different Terrains Using Gaussian Process Mixture Models}}, booktitle = icra, year = 2019, url={https://www.ipb.uni-bonn.de/pdfs/nardi2019icra-airn.pdf}, videourl = {https://youtu.be/DlMbP3u1g2Y}, }
- D. Wilbers, C. Merfels, and C. Stachniss, “Localization with Sliding Window Factor Graphs on Third-Party Maps for Automated Driving,” in Proc. of the IEEE Intl. Conf. on Robotics & Automation (ICRA), 2019.
[BibTeX] [PDF]@InProceedings{wilbers2019icra, author = {D. Wilbers and Ch. Merfels and C. Stachniss}, title = {{Localization with Sliding Window Factor Graphs on Third-Party Maps for Automated Driving}}, booktitle = icra, year = 2019, }
- N. Chebrolu, P. Lottes, T. Laebe, and C. Stachniss, “Robot Localization Based on Aerial Images for Precision Agriculture Tasks in Crop Fields,” in Proc. of the IEEE Intl. Conf. on Robotics & Automation (ICRA), 2019.
[BibTeX] [PDF] [Video]@InProceedings{chebrolu2019icra, author = {N. Chebrolu and P. Lottes and T. Laebe and C. Stachniss}, title = {{Robot Localization Based on Aerial Images for Precision Agriculture Tasks in Crop Fields}}, booktitle = icra, year = 2019, url = {https://www.ipb.uni-bonn.de/pdfs/chebrolu2019icra.pdf}, videourl = {https://youtu.be/TlijLgoRLbc}, }
- K. Huang, J. Xiao, and C. Stachniss, “Accurate Direct Visual-Laser Odometry with Explicit Occlusion Handling and Plane Detection,” in Proc. of the IEEE Intl. Conf. on Robotics & Automation (ICRA), 2019.
[BibTeX] [PDF]@InProceedings{huang2019icra, author = {K. Huang and J. Xiao and C. Stachniss}, title = {{Accurate Direct Visual-Laser Odometry with Explicit Occlusion Handling and Plane Detection}}, booktitle = icra, year = 2019, }
- R. Schirmer, P. Bieber, and C. Stachniss, “Coverage Path Planning in Belief Space ,” in Proc. of the IEEE Intl. Conf. on Robotics & Automation (ICRA), 2019.
[BibTeX] [PDF]@InProceedings{schirmer2019icra, author = {R. Schirmer and P. Bieber and C. Stachniss}, title = {{Coverage Path Planning in Belief Space }}, booktitle = icra, year = 2019, }
- D. Wilbers, L. Rumberg, and C. Stachniss, “Approximating Marginalization with Sparse Global Priors for Sliding Window SLAM-Graphs,” in Proc. of the IEEE Intl. Conf. on Robotic Computing (IRC), 2019.
[BibTeX] [PDF]
Most autonomous vehicles rely on some kind of map for localization or navigation. Outdated maps however are a risk to the performance of any map-based localization system applied in autonomous vehicles. It is necessary to update the used maps to ensure stable and long-term operation. We address the problem of computing landmark updates live in the vehicle, which requires efficient use of the computational resources. In particular, we employ a graph-based sliding window approach for simultaneous localization and incremental map refinement. We propose a novel method that approximates sliding window marginalization without inducing fill-in. Our method maintains the exact same sparsity pattern as without performing marginalization, but simultaneously improves the landmark estimates. The main novelty of this work is the derivation of sparse global priors that approximate dense marginalization. In comparison to state-of-the-art work, our approach utilizes global instead of local linearization points, but still minimizes linearization errors. We first approximate marginalization via Kullback-Leibler divergence and then recalculate the mean to compensate linearization errors. We evaluate our approach on simulated and real data from a prototype vehicle and compare our approach to state-of-the-art sliding window marginalization.
@InProceedings{wilbers2019irc-amws, author = {D. Wilbers and L. Rumberg and C. Stachniss}, title = {{Approximating Marginalization with Sparse Global Priors for Sliding Window SLAM-Graphs}}, booktitle = {Proc. of the IEEE Intl. Conf. on Robotic Computing (IRC)}, year = 2019, abstract = {Most autonomous vehicles rely on some kind of map for localization or navigation. Outdated maps however are a risk to the performance of any map-based localization system applied in autonomous vehicles. It is necessary to update the used maps to ensure stable and long-term operation. We address the problem of computing landmark updates live in the vehicle, which requires efficient use of the computational resources. In particular, we employ a graph-based sliding window approach for simultaneous localization and incremental map refinement. We propose a novel method that approximates sliding window marginalization without inducing fill-in. Our method maintains the exact same sparsity pattern as without performing marginalization, but simultaneously improves the landmark estimates. The main novelty of this work is the derivation of sparse global priors that approximate dense marginalization. In comparison to state-of-the-art work, our approach utilizes global instead of local linearization points, but still minimizes linearization errors. We first approximate marginalization via Kullback-Leibler divergence and then recalculate the mean to compensate linearization errors. We evaluate our approach on simulated and real data from a prototype vehicle and compare our approach to state-of-the-art sliding window marginalization.}, }
- D. Wilbers, C. Merfels, and C. Stachniss, “A Comparison of Particle Filter and Graph-based Optimization for Localization with Landmarks in Automated Vehicles,” in Proc. of the IEEE Intl. Conf. on Robotic Computing (IRC), 2019.
[BibTeX] [PDF]@InProceedings{wilbers2019irc-cpfg, author = {D. Wilbers and Ch. Merfels and C. Stachniss}, title = {{A Comparison of Particle Filter and Graph-based Optimization for Localization with Landmarks in Automated Vehicles}}, booktitle = {Proc. of the IEEE Intl. Conf. on Robotic Computing (IRC)}, year = 2019, }
- P. Lottes, N. Chebrolu, F. Liebisch, and C. Stachniss, “UAV-based Field Monitoring for Precision Farming,” in Proc. of the 25th Workshop für Computer-Bildanalyse und unbemannte autonom fliegende Systeme in der Landwirtschaft, 2019.
[BibTeX] [PDF]@InProceedings{lottes2019cbaws, title={UAV-based Field Monitoring for Precision Farming}, author={P. Lottes and N. Chebrolu and F. Liebisch and C. Stachniss}, booktitle= {Proc. of the 25th Workshop f\"ur Computer-Bildanalyse und unbemannte autonom fliegende Systeme in der Landwirtschaft}, year= {2019}, url= {https://www.ipb.uni-bonn.de/wp-content/papercite-data/pdf/lottes2019cbaws.pdf}, }
2018
- I. Sa, M. Popovic, R. Khanna, Z. Chen, P. Lottes, F. Liebisch, J. Nieto, C. Stachniss, and R. Siegwart, “WeedMap: A Large-Scale Semantic Weed Mapping Framework Using Aerial Multispectral Imaging and Deep Neural Network for Precision Farming,” , vol. 10, 2018. doi:10.3390/rs10091423
[BibTeX] [PDF]
{The ability to automatically monitor agricultural fields is an important capability in precision farming, enabling steps towards more sustainable agriculture. Precise, high-resolution monitoring is a key prerequisite for targeted intervention and the selective application of agro-chemicals. The main goal of this paper is developing a novel crop/weed segmentation and mapping framework that processes multispectral images obtained from an unmanned aerial vehicle (UAV) using a deep neural network (DNN). Most studies on crop/weed semantic segmentation only consider single images for processing and classification. Images taken by UAVs often cover only a few hundred square meters with either color only or color and near-infrared (NIR) channels. Although a map can be generated by processing single segmented images incrementally, this requires additional complex information fusion techniques which struggle to handle high fidelity maps due to their computational costs and problems in ensuring global consistency. Moreover, computing a single large and accurate vegetation map (e.g., crop/weed) using a DNN is non-trivial due to difficulties arising from: (1) limited ground sample distances (GSDs) in high-altitude datasets, (2) sacrificed resolution resulting from downsampling high-fidelity images, and (3) multispectral image alignment. To address these issues, we adopt a stand sliding window approach that operates on only small portions of multispectral orthomosaic maps (tiles), which are channel-wise aligned and calibrated radiometrically across the entire map. We define the tile size to be the same as that of the DNN input to avoid resolution loss. Compared to our baseline model (i.e., SegNet with 3 channel RGB inputs) yielding an area under the curve (AUC) of [background=0.607
@Article{sa2018rs, author = {I. Sa and M. Popovic and R. Khanna and Z. Chen and P. Lottes and F. Liebisch and J. Nieto and C. Stachniss and R. Siegwart}, title = {{WeedMap: A Large-Scale Semantic Weed Mapping Framework Using Aerial Multispectral Imaging and Deep Neural Network for Precision Farming}}, journal = rs, year = 2018, volume = 10, issue = 9, url = {https://www.mdpi.com/2072-4292/10/9/1423/pdf}, doi = {10.3390/rs10091423}, abstract = {The ability to automatically monitor agricultural fields is an important capability in precision farming, enabling steps towards more sustainable agriculture. Precise, high-resolution monitoring is a key prerequisite for targeted intervention and the selective application of agro-chemicals. The main goal of this paper is developing a novel crop/weed segmentation and mapping framework that processes multispectral images obtained from an unmanned aerial vehicle (UAV) using a deep neural network (DNN). Most studies on crop/weed semantic segmentation only consider single images for processing and classification. Images taken by UAVs often cover only a few hundred square meters with either color only or color and near-infrared (NIR) channels. Although a map can be generated by processing single segmented images incrementally, this requires additional complex information fusion techniques which struggle to handle high fidelity maps due to their computational costs and problems in ensuring global consistency. Moreover, computing a single large and accurate vegetation map (e.g., crop/weed) using a DNN is non-trivial due to difficulties arising from: (1) limited ground sample distances (GSDs) in high-altitude datasets, (2) sacrificed resolution resulting from downsampling high-fidelity images, and (3) multispectral image alignment. To address these issues, we adopt a stand sliding window approach that operates on only small portions of multispectral orthomosaic maps (tiles), which are channel-wise aligned and calibrated radiometrically across the entire map. We define the tile size to be the same as that of the DNN input to avoid resolution loss. Compared to our baseline model (i.e., SegNet with 3 channel RGB inputs) yielding an area under the curve (AUC) of [background=0.607, crop=0.681, weed=0.576], our proposed model with 9 input channels achieves [0.839, 0.863, 0.782]. Additionally, we provide an extensive analysis of 20 trained models, both qualitatively and quantitatively, in order to evaluate the effects of varying input channels and tunable network hyperparameters. Furthermore, we release a large sugar beet/weed aerial dataset with expertly guided annotations for further research in the fields of remote sensing, precision agriculture, and agricultural robotics.}, }
- N. Chebrolu, T. Läbe, and C. Stachniss, “Robust Long-Term Registration of UAV Images of Crop Fields for Precision Agriculture,” IEEE Robotics and Automation Letters (RA-L), vol. 3, iss. 4, pp. 3097-3104, 2018. doi:10.1109/LRA.2018.2849603
[BibTeX] [PDF]@Article{chebrolu2018ral, author={N. Chebrolu and T. L\"abe and C. Stachniss}, journal=ral, title={Robust Long-Term Registration of UAV Images of Crop Fields for Precision Agriculture}, year={2018}, volume={3}, number={4}, pages={3097-3104}, keywords={Agriculture;Cameras;Geometry;Monitoring;Robustness;Three-dimensional displays;Visualization;Robotics in agriculture and forestry;SLAM}, doi={10.1109/LRA.2018.2849603}, url={https://www.ipb.uni-bonn.de/pdfs/chebrolu2018ral.pdf} }
- P. Lottes, J. Behley, A. Milioto, and C. Stachniss, “Fully Convolutional Networks with Sequential Information for Robust Crop and Weed Detection in Precision Farming,” IEEE Robotics and Automation Letters (RA-L), vol. 3, pp. 3097-3104, 2018. doi:10.1109/LRA.2018.2846289
[BibTeX] [PDF] [Video]@Article{lottes2018ral, author = {P. Lottes and J. Behley and A. Milioto and C. Stachniss}, title = {Fully Convolutional Networks with Sequential Information for Robust Crop and Weed Detection in Precision Farming}, journal = ral, year = {2018}, volume = {3}, issue = {4}, pages = {3097-3104}, doi = {10.1109/LRA.2018.2846289}, url = {https://www.ipb.uni-bonn.de/pdfs/lottes2018ral.pdf}, videourl = {https://www.youtube.com/watch?v=vTepw9HRLh8}, }
- P. Regier, A. Milioto, P. Karkowski, C. Stachniss, and M. Bennewitz, “Classifying Obstacles and Exploiting Knowledge about Classes for Efficient Humanoid Navigation,” in Proc. of the IEEE-RAS Int. Conf. on Humanoid Robots (HUMANOIDS), 2018.
[BibTeX] [PDF]@InProceedings{regier2018humanoids, author = {P. Regier and A. Milioto and P. Karkowski and C. Stachniss and M. Bennewitz}, title = {{Classifying Obstacles and Exploiting Knowledge about Classes for Efficient Humanoid Navigation}}, booktitle = {Proc. of the IEEE-RAS Int. Conf. on Humanoid Robots (HUMANOIDS)}, year = 2018, }
- K. H. Huang and C. Stachniss, “Joint Ego-motion Estimation Using a Laser Scanner and a Monocular Camera Through Relative Orientation Estimation and 1-DoF ICP,” in Proc. of the IEEE/RSJ Intl. Conf. on Intelligent Robots and Systems (IROS), 2018.
[BibTeX] [PDF] [Video]
Pose estimation and mapping are key capabilities of most autonomous vehicles and thus a number of localization and SLAM algorithms have been developed in the past. Autonomous robots and cars are typically equipped with multiple sensors. Often, the sensor suite includes a camera and a laser range finder. In this paper, we consider the problem of incremental ego-motion estimation, using both, a monocular camera and a laser range finder jointly. We propose a new algorithm, that exploits the advantages of both sensors–-the ability of cameras to determine orientations well and the ability of laser range finders to estimate the scale and to directly obtain 3D point clouds. Our approach estimates the five degree of freedom relative orientation from image pairs through feature point correspondences and formulates the remaining scale estimation as a new variant of the iterative closet point problem with only one degree of freedom. We furthermore exploit the camera information in a new way to constrain the data association between laser point clouds. The experiments presented in this paper suggest that our approach is able to accurately estimate the ego-motion of a vehicle and that we obtain more accurate frame-to-frame alignments than with one sensor modality alone.
@InProceedings{huang2018iros, author = {K.H. Huang and C. Stachniss}, title = {{Joint Ego-motion Estimation Using a Laser Scanner and a Monocular Camera Through Relative Orientation Estimation and 1-DoF ICP}}, booktitle = iros, year = 2018, videourl = {https://www.youtube.com/watch?v=Glv0UT_KqoM}, abstract = {Pose estimation and mapping are key capabilities of most autonomous vehicles and thus a number of localization and SLAM algorithms have been developed in the past. Autonomous robots and cars are typically equipped with multiple sensors. Often, the sensor suite includes a camera and a laser range finder. In this paper, we consider the problem of incremental ego-motion estimation, using both, a monocular camera and a laser range finder jointly. We propose a new algorithm, that exploits the advantages of both sensors---the ability of cameras to determine orientations well and the ability of laser range finders to estimate the scale and to directly obtain 3D point clouds. Our approach estimates the five degree of freedom relative orientation from image pairs through feature point correspondences and formulates the remaining scale estimation as a new variant of the iterative closet point problem with only one degree of freedom. We furthermore exploit the camera information in a new way to constrain the data association between laser point clouds. The experiments presented in this paper suggest that our approach is able to accurately estimate the ego-motion of a vehicle and that we obtain more accurate frame-to-frame alignments than with one sensor modality alone.} }
- P. Lottes, J. Behley, N. Chebrolu, A. Milioto, and C. Stachniss, “Joint Stem Detection and Crop-Weed Classification for Plant-specific Treatment in Precision Farming,” in Proc. of the IEEE/RSJ Intl. Conf. on Intelligent Robots and Systems (IROS), 2018.
[BibTeX] [PDF] [Video]
Applying agrochemicals is the default procedure for conventional weed control in crop production, but has negative impacts on the environment. Robots have the potential to treat every plant in the field individually and thus can reduce the required use of such chemicals. To achieve that, robots need the ability to identify crops and weeds in the field and must additionally select effective treatments. While certain types of weed can be treated mechanically, other types need to be treated by (selective) spraying. In this paper, we present an approach that provides the necessary information for effective plant-specific treatment. It outputs the stem location for weeds, which allows for mechanical treatments, and the covered area of the weed for selective spraying. Our approach uses an end-to- end trainable fully convolutional network that simultaneously estimates stem positions as well as the covered area of crops and weeds. It jointly learns the class-wise stem detection and the pixel-wise semantic segmentation. Experimental evaluations on different real-world datasets show that our approach is able to reliably solve this problem. Compared to state-of-the-art approaches, our approach not only substantially improves the stem detection accuracy, i.e., distinguishing crop and weed stems, but also provides an improvement in the semantic segmentation performance.
@InProceedings{lottes2018iros, author = {P. Lottes and J. Behley and N. Chebrolu and A. Milioto and C. Stachniss}, title = {Joint Stem Detection and Crop-Weed Classification for Plant-specific Treatment in Precision Farming}, booktitle = iros, year = 2018, url = {https://www.ipb.uni-bonn.de/pdfs/lottes18iros.pdf}, videourl = {https://www.youtube.com/watch?v=C9mjZxE_Sxg}, abstract = {Applying agrochemicals is the default procedure for conventional weed control in crop production, but has negative impacts on the environment. Robots have the potential to treat every plant in the field individually and thus can reduce the required use of such chemicals. To achieve that, robots need the ability to identify crops and weeds in the field and must additionally select effective treatments. While certain types of weed can be treated mechanically, other types need to be treated by (selective) spraying. In this paper, we present an approach that provides the necessary information for effective plant-specific treatment. It outputs the stem location for weeds, which allows for mechanical treatments, and the covered area of the weed for selective spraying. Our approach uses an end-to- end trainable fully convolutional network that simultaneously estimates stem positions as well as the covered area of crops and weeds. It jointly learns the class-wise stem detection and the pixel-wise semantic segmentation. Experimental evaluations on different real-world datasets show that our approach is able to reliably solve this problem. Compared to state-of-the-art approaches, our approach not only substantially improves the stem detection accuracy, i.e., distinguishing crop and weed stems, but also provides an improvement in the semantic segmentation performance.} }
- J. Jung, C. Stachniss, S. Ju, and J. Heo, “Automated 3D volumetric reconstruction of multiple-room building interiors for as-built BIM,” Advanced Engineering Informatics, vol. 38, pp. 811-825, 2018. doi:10.1016/j.aei.2018.10.007
[BibTeX]
Currently, fully automated as-built modeling of building interiors using point-cloud data still remains an open challenge, due to several problems that repeatedly arise: (1) complex indoor environments containing multiple rooms; (2) time-consuming and labor-intensive noise filtering; (3) difficulties of representation of volumetric and detail-rich objects such as windows and doors. This study aimed to overcome such limitations while improving the amount of details reproduced within the model for further utilization in BIM. First, we input just the registered three-dimensional (3D) point-cloud data and segmented the point cloud into separate rooms for more effective performance of the later modeling phases for each room. For noise filtering, an offset space from the ceiling height was used to determine whether the scan points belonged to clutter or architectural components. The filtered points were projected onto a binary map in order to trace the floor-wall boundary, which was further refined through subsequent segmentation and regularization procedures. Then, the wall volumes were estimated in two ways: inside- and outside-wall-component modeling. Finally, the wall points were segmented and projected onto an inverse binary map, thereby enabling detection and modeling of the hollow areas as windows or doors. The experimental results on two real-world data sets demonstrated, through comparison with manually-generated models, the effectiveness of our approach: the calculated RMSEs of the two resulting models were 0.089m and 0.074m, respectively.
@article{jung2018aei, title = {Automated 3D volumetric reconstruction of multiple-room building interiors for as-built BIM}, journal = aei, author = {J. Jung and C. Stachniss and S. Ju and J. Heo}, volume = {38}, pages = {811-825}, year = 2018, issn = {1474-0346}, doi = {10.1016/j.aei.2018.10.007}, _weburl = {https://www.sciencedirect.com/science/article/pii/S1474034618300600}, abstract = {Currently, fully automated as-built modeling of building interiors using point-cloud data still remains an open challenge, due to several problems that repeatedly arise: (1) complex indoor environments containing multiple rooms; (2) time-consuming and labor-intensive noise filtering; (3) difficulties of representation of volumetric and detail-rich objects such as windows and doors. This study aimed to overcome such limitations while improving the amount of details reproduced within the model for further utilization in BIM. First, we input just the registered three-dimensional (3D) point-cloud data and segmented the point cloud into separate rooms for more effective performance of the later modeling phases for each room. For noise filtering, an offset space from the ceiling height was used to determine whether the scan points belonged to clutter or architectural components. The filtered points were projected onto a binary map in order to trace the floor-wall boundary, which was further refined through subsequent segmentation and regularization procedures. Then, the wall volumes were estimated in two ways: inside- and outside-wall-component modeling. Finally, the wall points were segmented and projected onto an inverse binary map, thereby enabling detection and modeling of the hollow areas as windows or doors. The experimental results on two real-world data sets demonstrated, through comparison with manually-generated models, the effectiveness of our approach: the calculated RMSEs of the two resulting models were 0.089m and 0.074m, respectively.} }
- J. Behley and C. Stachniss, “Efficient Surfel-Based SLAM using 3D Laser Range Data in Urban Environments,” in Proc. of Robotics: Science and Systems (RSS), 2018.
[BibTeX] [PDF] [Video]@InProceedings{behley2018rss, author = {J. Behley and C. Stachniss}, title = {Efficient Surfel-Based SLAM using 3D Laser Range Data in Urban Environments}, booktitle = rss, year = 2018, videourl = {https://www.youtube.com/watch?v=-AEX203rXkE}, url = {https://www.roboticsproceedings.org/rss14/p16.pdf}, }
- T. Naseer, W. Burgard, and C. Stachniss, “Robust Visual Localization Across Seasons,” IEEE Transactions on Robotics, pp. 1-14, 2018. doi:10.1109/tro.2017.2788045
[BibTeX] [PDF]@Article{naseer2018tro, author = {T. Naseer and W. Burgard and C. Stachniss}, title = {Robust Visual Localization Across Seasons}, journal = ieeetransrob, year = 2018, pages = {1-14}, doi = {10.1109/tro.2017.2788045}, url = {https://www.ipb.uni-bonn.de/pdfs/naseer2018tro.pdf}, }
- B. Della Corte, I. Bogoslavskyi, C. Stachniss, and G. Grisetti, “A General Framework for Flexible Multi-Cue Photometric Point Cloud Registration,” in Proc. of the IEEE Intl. Conf. on Robotics & Automation (ICRA), 2018.
[BibTeX] [PDF] [Code] [Video]@InProceedings{della-corte2018icra, author = {Della Corte, B. and I. Bogoslavskyi and C. Stachniss and G. Grisetti}, title = {A General Framework for Flexible Multi-Cue Photometric Point Cloud Registration}, year = 2018, booktitle = icra, codeurl = {https://gitlab.com/srrg-software/srrg_mpr}, videourl = {https://www.youtube.com/watch?v=_z98guJTqfk}, url = {https://www.ipb.uni-bonn.de/wp-content/papercite-data/pdf/della-corte2018icra.pdf}, }
- A. Milioto, P. Lottes, and C. Stachniss, “Real-time Semantic Segmentation of Crop and Weed for Precision Agriculture Robots Leveraging Background Knowledge in CNNs,” in Proc. of the IEEE Intl. Conf. on Robotics & Automation (ICRA), 2018.
[BibTeX] [PDF] [Video]
Precision farming robots, which target to reduce the amount of herbicides that need to be brought out in the fields, must have the ability to identify crops and weeds in real time to trigger weeding actions. In this paper, we address the problem of CNN-based semantic segmentation of crop fields separating sugar beet plants, weeds, and background solely based on RGB data. We propose a CNN that exploits existing vegetation indexes and provides a classification in real time. Furthermore, it can be effectively re-trained to so far unseen fields with a comparably small amount of training data. We implemented and thoroughly evaluated our system on a real agricultural robot operating in different fields in Germany and Switzerland. The results show that our system generalizes well, can operate at around 20Hz, and is suitable for online operation in the fields.
@InProceedings{milioto2018icra, author = {A. Milioto and P. Lottes and C. Stachniss}, title = {Real-time Semantic Segmentation of Crop and Weed for Precision Agriculture Robots Leveraging Background Knowledge in CNNs}, year = {2018}, booktitle = icra, abstract = {Precision farming robots, which target to reduce the amount of herbicides that need to be brought out in the fields, must have the ability to identify crops and weeds in real time to trigger weeding actions. In this paper, we address the problem of CNN-based semantic segmentation of crop fields separating sugar beet plants, weeds, and background solely based on RGB data. We propose a CNN that exploits existing vegetation indexes and provides a classification in real time. Furthermore, it can be effectively re-trained to so far unseen fields with a comparably small amount of training data. We implemented and thoroughly evaluated our system on a real agricultural robot operating in different fields in Germany and Switzerland. The results show that our system generalizes well, can operate at around 20Hz, and is suitable for online operation in the fields.}, url = {https://arxiv.org/abs/1709.06764}, videourl = {https://youtu.be/DXcTkJmdWFQ}, }
- A. Milioto and C. Stachniss, “Bonnet: An Open-Source Training and Deployment Framework for Semantic Segmentation in Robotics using CNNs,” ICRA Worshop on Perception, Inference, and Learning for Joint Semantic, Geometric, and Physical Understanding, 2018.
[BibTeX] [PDF] [Code] [Video]@Article{milioto2018icraws, author = {A. Milioto and C. Stachniss}, title = "{Bonnet: An Open-Source Training and Deployment Framework for Semantic Segmentation in Robotics using CNNs}", journal = {ICRA Worshop on Perception, Inference, and Learning for Joint Semantic, Geometric, and Physical Understanding}, eprint = {1802.08960}, primaryclass = "cs.RO", keywords = {Computer Science - Robotics, Computer Science - Computer Vision and Pattern Recognition}, year = 2018, month = may, url = {https://arxiv.org/abs/1802.08960}, codeurl = {https://github.com/Photogrammetry-Robotics-Bonn/bonnet}, videourl = {https://www.youtube.com/watch?v=tfeFHCq6YJs}, }
- E. Palazzolo and C. Stachniss, “Effective Exploration for MAVs Based on the Expected Information Gain,” Drones, vol. 2, iss. 1, 2018. doi:10.3390/drones2010009
[BibTeX] [PDF]
Micro aerial vehicles (MAVs) are an excellent platform for autonomous exploration. Most MAVs rely mainly on cameras for buliding a map of the 3D environment. Therefore, vision-based MAVs require an efficient exploration algorithm to select viewpoints that provide informative measurements. In this paper, we propose an exploration approach that selects in real time the next-best-view that maximizes the expected information gain of new measurements. In addition, we take into account the cost of reaching a new viewpoint in terms of distance and predictability of the flight path for a human observer. Finally, our approach selects a path that reduces the risk of crashes when the expected battery life comes to an end, while still maximizing the information gain in the process. We implemented and thoroughly tested our approach and the experiments show that it offers an improved performance compared to other state-of-the-art algorithms in terms of precision of the reconstruction, execution time, and smoothness of the path.
@Article{palazzolo2018drones, author = {E. Palazzolo and C. Stachniss}, title = {{Effective Exploration for MAVs Based on the Expected Information Gain}}, journal = {Drones}, volume = {2}, year = {2018}, number = {1}, article-number= {9}, url = {https://www.ipb.uni-bonn.de/pdfs/palazzolo2018drones.pdf}, issn = {2504-446X}, abstract = {Micro aerial vehicles (MAVs) are an excellent platform for autonomous exploration. Most MAVs rely mainly on cameras for buliding a map of the 3D environment. Therefore, vision-based MAVs require an efficient exploration algorithm to select viewpoints that provide informative measurements. In this paper, we propose an exploration approach that selects in real time the next-best-view that maximizes the expected information gain of new measurements. In addition, we take into account the cost of reaching a new viewpoint in terms of distance and predictability of the flight path for a human observer. Finally, our approach selects a path that reduces the risk of crashes when the expected battery life comes to an end, while still maximizing the information gain in the process. We implemented and thoroughly tested our approach and the experiments show that it offers an improved performance compared to other state-of-the-art algorithms in terms of precision of the reconstruction, execution time, and smoothness of the path.}, doi = {10.3390/drones2010009}, }
- E. Palazzolo and C. Stachniss, “Fast Image-Based Geometric Change Detection Given a 3D Model,” in Proc. of the IEEE Intl. Conf. on Robotics & Automation (ICRA), 2018.
[BibTeX] [PDF] [Code] [Video]@InProceedings{palazzolo2018icra, title = {{Fast Image-Based Geometric Change Detection Given a 3D Model}}, author = {E. Palazzolo and C. Stachniss}, booktitle = icra, year = {2018}, url = {https://www.ipb.uni-bonn.de/pdfs/palazzolo2018icra.pdf}, codeurl = {https://github.com/PRBonn/fast_change_detection}, videourl = {https://youtu.be/DEkOYf4Zzh4}, }
- K. H. Huang and C. Stachniss, “On Geometric Models and Their Accuracy for Extrinsic Sensor Calibration,” in Proc. of the IEEE Intl. Conf. on Robotics & Automation (ICRA), 2018.
[BibTeX] [PDF]@InProceedings{huang2018icra, author = {K.H. Huang and C. Stachniss}, title = {On Geometric Models and Their Accuracy for Extrinsic Sensor Calibration}, booktitle = icra, year = 2018, url = {https://www.ipb.uni-bonn.de/pdfs/huang2018icra.pdf}, }
- A. Walter, R. Khanna, P. Lottes, C. Stachniss, R. Siegwart, J. Nieto, and F. Liebisch, “Flourish – A robotic approach for automation in crop management,” in Proc. of the Intl. Conf. on Precision Agriculture (ICPA), 2018.
[BibTeX] [PDF]
The Flourish project aims to bridge the gap between current and desired capabilities of agricultural robots by developing an adaptable robotic solution for precision farming. Combining the aerial survey capabilities of a small autonomous multi-copter Unmanned Aerial Vehicle (UAV) with a multi-purpose agricultural Unmanned Ground Vehicle (UGV), the system will be able to survey a field from the air, perform targeted intervention on the ground, and provide detailed information for decision support, all with minimal user intervention. The system can be adapted to a wide range of farm management activities and to different crops by choosing different sensors, status indicators and ground treatment packages. The research project thereby touches a selection of topics addressed by ICPA such as sensor application in managing in-season crop variability, precision nutrient management and crop protection as well as remote sensing applications in precision agriculture and engineering technologies and advances. This contribution will introduce the Flourish consortium and concept using the results of three years of active development, testing, and measuring in field campaigns. Two key parts of the project will be shown in more detail: First, mapping of the field by drones for detection of sugar beet nitrogen status variation and weed pressure in the field and second the perception of the UGV as related to weed classification and subsequent precision weed management. The field mapping by means of an UAV will be shown for crop nitrogen status estimation and weed pressure with examples for subsequent crop management decision support. For nitrogen status, the results indicate that drones are up to the task to deliver crop nitrogen variability maps utilized for variable rate application that are of comparable quality to current on-tractor systems. The weed pressure mapping is viable as basis for the UGV showcase of precision weed management. For this, we show the automated image acquisition by the UGV and a subsequent plant classification with a four-step pipeline, differentiating crop from weed in real time. Advantages and disadvantages as well as future prospects of such approaches will be discussed.
@InProceedings{walter2018icpa, Title = {Flourish - A robotic approach for automation in crop management}, Author = {A. Walter and R. Khanna and P. Lottes and C. Stachniss and R. Siegwart and J. Nieto and F. Liebisch}, Booktitle = icpa, Year = 2018, abstract = {The Flourish project aims to bridge the gap between current and desired capabilities of agricultural robots by developing an adaptable robotic solution for precision farming. Combining the aerial survey capabilities of a small autonomous multi-copter Unmanned Aerial Vehicle (UAV) with a multi-purpose agricultural Unmanned Ground Vehicle (UGV), the system will be able to survey a field from the air, perform targeted intervention on the ground, and provide detailed information for decision support, all with minimal user intervention. The system can be adapted to a wide range of farm management activities and to different crops by choosing different sensors, status indicators and ground treatment packages. The research project thereby touches a selection of topics addressed by ICPA such as sensor application in managing in-season crop variability, precision nutrient management and crop protection as well as remote sensing applications in precision agriculture and engineering technologies and advances. This contribution will introduce the Flourish consortium and concept using the results of three years of active development, testing, and measuring in field campaigns. Two key parts of the project will be shown in more detail: First, mapping of the field by drones for detection of sugar beet nitrogen status variation and weed pressure in the field and second the perception of the UGV as related to weed classification and subsequent precision weed management. The field mapping by means of an UAV will be shown for crop nitrogen status estimation and weed pressure with examples for subsequent crop management decision support. For nitrogen status, the results indicate that drones are up to the task to deliver crop nitrogen variability maps utilized for variable rate application that are of comparable quality to current on-tractor systems. The weed pressure mapping is viable as basis for the UGV showcase of precision weed management. For this, we show the automated image acquisition by the UGV and a subsequent plant classification with a four-step pipeline, differentiating crop from weed in real time. Advantages and disadvantages as well as future prospects of such approaches will be discussed.}, }
- F. Langer, L. Mandtler, A. Milioto, E. Palazzolo, and C. Stachniss, “Geometrical Stem Detection from Image Data for Precision Agriculture,” arXiv Preprint, 2018.
[BibTeX] [PDF]@article{langer2018arxiv, author = {F. Langer and L. Mandtler and A. Milioto and E. Palazzolo and C. Stachniss}, title = {{Geometrical Stem Detection from Image Data for Precision Agriculture}}, journal = arxiv, year = 2018, eprint = {1812.05415v1}, url = {https://arxiv.org/pdf/1812.05415v1}, keywords = {cs.RO}, }
- L. Nardi and C. Stachniss, “Towards Uncertainty-Aware Path Planning for Navigation on Road Networks Using Augmented MDPs,” in 10th Workshop on Planning, Perception and Navigation for Intelligent Vehicles at the IEEE/RSJ Int. Conf. on Intelligent Robots and Systems (IROS), 2018.
[BibTeX] [PDF] [Video]@InProceedings{nardi2018ppniv, title = {Towards Uncertainty-Aware Path Planning for Navigation on Road Networks Using Augmented MDPs}, author = {L. Nardi and C. Stachniss}, booktitle = {10th Workshop on Planning, Perception and Navigation for Intelligent Vehicles at the IEEE/RSJ Int. Conf. on Intelligent Robots and Systems (IROS)}, year = {2018}, videourl = {https://youtu.be/SLp5YVplJAQ} }
2017
- C. Beekmans, J. Schneider, T. Laebe, M. Lennefer, C. Stachniss, and C. Simmer, “3D-Cloud Morphology and Motion from Dense Stereo for Fisheye Cameras,” in In Proc. of the European Geosciences Union General Assembly (EGU), 2017.
[BibTeX] [PDF]@InProceedings{beekmans2017egu, title = {3D-Cloud Morphology and Motion from Dense Stereo for Fisheye Cameras}, author = {Ch. Beekmans and J. Schneider and T. Laebe and M. Lennefer and C. Stachniss and C. Simmer}, booktitle = {In Proc. of the European Geosciences Union General Assembly (EGU)}, year = {2017}, }
- I. Bogoslavskyi and C. Stachniss, “Analyzing the Quality of Matched 3D Point Clouds of Objects,” in Proc. of the IEEE/RSJ Intl. Conf. on Intelligent Robots and Systems (IROS), 2017.
[BibTeX] [PDF]
[none]
@InProceedings{bogoslavskyi2017iros, title = {Analyzing the Quality of Matched 3D Point Clouds of Objects}, author = {I. Bogoslavskyi and C. Stachniss}, booktitle = iros, year = {2017}, abstract = {[none]}, url = {https://www.ipb.uni-bonn.de/pdfs/bogoslavskyi17iros.pdf}, }
- I. Bogoslavskyi and C. Stachniss, “Efficient Online Segmentation for Sparse 3D Laser Scans,” in Journal of Photogrammetry, Remote Sensing and Geoinformation Science (PFG), 2017, p. 41–52.
[BibTeX] [PDF] [Code] [Video]
The ability to extract individual objects in the scene is key for a large number of autonomous navigation systems such as mobile robots or autonomous cars. Such systems navigating in dynamic environments need to be aware of objects that may change or move. In most perception cues, a pre-segmentation of the current image or laser scan into individual objects is the first processing step before a further analysis is performed. In this paper, we present an effective method that first removes the ground from the scan and then segments the 3D data in a range image representation into different objects. A key focus of our work is a fast execution with several hundred Hertz. Our implementation has small computational demands so that it can run online on most mobile systems. We explicitly avoid the computation of the 3D point cloud and operate directly on a 2.5D range image, which enables a fast segmentation for each 3D scan. This approach can furthermore handle sparse 3D data well, which is important for scanners such as the new Velodyne VLP-16 scanner. We implemented our approach in C++ and ROS, thoroughly tested it using different 3D scanners, and will release the source code of our implementation. Our method can operate at frame rates that are substantially higher than those of the sensors while using only a single core of a mobile CPU and producing high-quality segmentation results.
@InProceedings{bogoslavskyi2017pfg, title = {Efficient Online Segmentation for Sparse 3D Laser Scans}, author = {Bogoslavskyi, Igor and Stachniss, Cyrill}, booktitle = pfg, year = {2017}, pages = {41--52}, volume = {85}, issue = {1}, abstract = {The ability to extract individual objects in the scene is key for a large number of autonomous navigation systems such as mobile robots or autonomous cars. Such systems navigating in dynamic environments need to be aware of objects that may change or move. In most perception cues, a pre-segmentation of the current image or laser scan into individual objects is the first processing step before a further analysis is performed. In this paper, we present an effective method that first removes the ground from the scan and then segments the 3D data in a range image representation into different objects. A key focus of our work is a fast execution with several hundred Hertz. Our implementation has small computational demands so that it can run online on most mobile systems. We explicitly avoid the computation of the 3D point cloud and operate directly on a 2.5D range image, which enables a fast segmentation for each 3D scan. This approach can furthermore handle sparse 3D data well, which is important for scanners such as the new Velodyne VLP-16 scanner. We implemented our approach in C++ and ROS, thoroughly tested it using different 3D scanners, and will release the source code of our implementation. Our method can operate at frame rates that are substantially higher than those of the sensors while using only a single core of a mobile CPU and producing high-quality segmentation results.}, url = {https://www.ipb.uni-bonn.de/pdfs/bogoslavskyi16pfg.pdf}, codeurl = {https://github.com/Photogrammetry-Robotics-Bonn/depth_clustering}, videourl = {https://www.youtube.com/watch?v=6WqsOlHGTLA}, }
- F. Liebisch, M. Popovic, J. Pfeifer, R. Khanna, P. Lottes, C. Stachniss, A. Pretto, I. S. Kyu, J. Nieto, R. Siegwart, and A. Walter, “Automatic UAV-based field inspection campaigns for weeding in row crops,” in Proc. of the 10th EARSeL SIG Imaging Spectroscopy Workshop, 2017.
[BibTeX]@InProceedings{liebisch2017earsel, title = {Automatic UAV-based field inspection campaigns for weeding in row crops}, author = {F. Liebisch and M. Popovic and J. Pfeifer and R. Khanna and P. Lottes and C. Stachniss and A. Pretto and S. In Kyu and J. Nieto and R. Siegwart and A. Walter}, booktitle = {Proc. of the 10th EARSeL SIG Imaging Spectroscopy Workshop}, year = {2017}, }
- P. Lottes, M. Höferlin, S. Sander, and C. Stachniss, “Effective Vision-based Classification for Separating Sugar Beets and Weeds for Precision Farming,” Journal of Field Robotics, vol. 34, pp. 1160-1178, 2017. doi:10.1002/rob.21675
[BibTeX] [PDF]@Article{lottes2017jfr, title = {Effective Vision-based Classification for Separating Sugar Beets and Weeds for Precision Farming}, author = {Lottes, Philipp and H\"oferlin, Markus and Sander, Slawomir and Stachniss, Cyrill}, journal = {Journal of Field Robotics}, year = {2017}, volume = {34}, issue = {6}, pages = {1160-1178}, doi = {10.1002/rob.21675}, issn = {1556-4967}, timestamp = {2016.10.5}, url = {https://www.ipb.uni-bonn.de/wp-content/papercite-data/pdf/lottes16jfr.pdf}, }
- N. Chebrolu, P. Lottes, A. Schaefer, W. Winterhalter, W. Burgard, and C. Stachniss, “Agricultural robot dataset for plant classification, localization and mapping on sugar beet fields,” The Intl. Journal of Robotics Research, 2017. doi:10.1177/0278364917720510
[BibTeX] [PDF]@Article{chebrolu2017ijrr, title = {Agricultural robot dataset for plant classification, localization and mapping on sugar beet fields}, author = {N. Chebrolu and P. Lottes and A. Schaefer and W. Winterhalter and W. Burgard and C. Stachniss}, journal = ijrr, year = {2017}, doi = {10.1177/0278364917720510}, url = {https://www.ipb.uni-bonn.de/wp-content/papercite-data/pdf/chebrolu2017ijrr.pdf}, }
- P. Lottes, R. Khanna, J. Pfeifer, R. Siegwart, and C. Stachniss, “UAV-Based Crop and Weed Classification for Smart Farming,” in Proc. of the IEEE Intl. Conf. on Robotics & Automation (ICRA), 2017.
[BibTeX] [PDF]@InProceedings{lottes2017icra, title = {UAV-Based Crop and Weed Classification for Smart Farming}, author = {P. Lottes and R. Khanna and J. Pfeifer and R. Siegwart and C. Stachniss}, booktitle = icra, year = {2017}, url = {https://www.ipb.uni-bonn.de/wp-content/papercite-data/pdf/lottes17icra.pdf}, }
- P. Lottes and C. Stachniss, “Semi-Supervised Online Visual Crop and Weed Classification in Precision Farming Exploiting Plant Arrangement,” in Proc. of the IEEE/RSJ Intl. Conf. on Intelligent Robots and Systems (IROS), 2017.
[BibTeX] [PDF]@InProceedings{lottes2017iros, title = {Semi-Supervised Online Visual Crop and Weed Classification in Precision Farming Exploiting Plant Arrangement}, author = {P. Lottes and C. Stachniss}, booktitle = iros, year = {2017}, url = {https://www.ipb.uni-bonn.de/wp-content/papercite-data/pdf/lottes17iros.pdf}, }
- C. Merfels and C. Stachniss, “Sensor Fusion for Self-Localisation of Automated Vehicles,” Journal of Photogrammetry, Remote Sensing and Geoinformation Science (PFG), 2017.
[BibTeX] [PDF]@Article{merfels2017pfg, title = {Sensor Fusion for Self-Localisation of Automated Vehicles}, author = {Merfels, C. and Stachniss, C.}, journal = pfg, year = {2017}, url = {https://link.springer.com/article/10.1007/s41064-017-0008-1}, }
- A. Milioto, P. Lottes, and C. Stachniss, “Real-time Blob-wise Sugar Beets vs Weeds Classification for Monitoring Fields using Convolutional Neural Networks,” in Proc. of the ISPRS Conf. on Unmanned Aerial Vehicles in Geomatics (UAV-g), 2017.
[BibTeX] [PDF]
UAVs are becoming an important tool for field monitoring and precision farming. A prerequisite for observing and analyzing fields is the ability to identify crops and weeds from image data. In this paper, we address the problem of detecting the sugar beet plants and weeds in the field based solely on image data. We propose a system that combines vegetation detection and deep learning to obtain a high-quality classification of the vegetation in the field into value crops and weeds. We implemented and thoroughly evaluated our system on image data collected from different sugar beet fields and illustrate that our approach allows for accurately identifying the weeds on the field.
@InProceedings{milioto2017uavg, title = {Real-time Blob-wise Sugar Beets vs Weeds Classification for Monitoring Fields using Convolutional Neural Networks}, author = {A. Milioto and P. Lottes and C. Stachniss}, booktitle = uavg, year = {2017}, abstract = {UAVs are becoming an important tool for field monitoring and precision farming. A prerequisite for observing and analyzing fields is the ability to identify crops and weeds from image data. In this paper, we address the problem of detecting the sugar beet plants and weeds in the field based solely on image data. We propose a system that combines vegetation detection and deep learning to obtain a high-quality classification of the vegetation in the field into value crops and weeds. We implemented and thoroughly evaluated our system on image data collected from different sugar beet fields and illustrate that our approach allows for accurately identifying the weeds on the field.}, url = {https://www.ipb.uni-bonn.de/pdfs/milioto17uavg.pdf}, }
- L. Nardi and C. Stachniss, “User Preferred Behaviors for Robot Navigation Exploiting Previous Experiences,” in Robotics and Autonomous Systems, 2017. doi:10.1016/j.robot.2017.08.014
[BibTeX] [PDF]
Industry demands flexible robots that are able to accomplish different tasks at different locations such as navigation and mobile manipulation. Operators often require mobile robots operating on factory floors to follow definite and predictable behaviors. This becomes particularly important when a robot shares the workspace with other moving entities. In this paper, we present a system for robot navigation that exploits previous experiences to generate predictable behaviors that meet user’s preferences. Preferences are not explicitly formulated but implicitly extracted from robot experiences and automatically considered to plan paths for the successive tasks without requiring experts to hard-code rules or strategies. Our system aims at accomplishing navigation behaviors that follow user’s preferences also to avoid dynamic obstacles. We achieve this by considering a probabilistic approach for modeling uncertain trajectories of the moving entities that share the workspace with the robot. We implemented and thoroughly tested our system both in simulation and on a real mobile robot. The extensive experiments presented in this paper demonstrate that our approach allows a robot for successfully navigating while performing predictable behaviors and meeting user’s preferences
@InProceedings{nardi2017jras, title = {User Preferred Behaviors for Robot Navigation Exploiting Previous Experiences}, author = {L. Nardi and C. Stachniss}, booktitle = jras, year = {2017}, doi = {10.1016/j.robot.2017.08.014}, abstract = {Industry demands flexible robots that are able to accomplish different tasks at different locations such as navigation and mobile manipulation. Operators often require mobile robots operating on factory floors to follow definite and predictable behaviors. This becomes particularly important when a robot shares the workspace with other moving entities. In this paper, we present a system for robot navigation that exploits previous experiences to generate predictable behaviors that meet user’s preferences. Preferences are not explicitly formulated but implicitly extracted from robot experiences and automatically considered to plan paths for the successive tasks without requiring experts to hard-code rules or strategies. Our system aims at accomplishing navigation behaviors that follow user’s preferences also to avoid dynamic obstacles. We achieve this by considering a probabilistic approach for modeling uncertain trajectories of the moving entities that share the workspace with the robot. We implemented and thoroughly tested our system both in simulation and on a real mobile robot. The extensive experiments presented in this paper demonstrate that our approach allows a robot for successfully navigating while performing predictable behaviors and meeting user’s preferences}, url = {https://www.ipb.uni-bonn.de/pdfs/nardi17jras.pdf}, }
- E. Palazzolo and C. Stachniss, “Information-Driven Autonomous Exploration for a Vision-Based MAV,” in ISPRS Annals of the Photogrammetry, Remote Sensing and Spatial Information Sciences, 2017.
[BibTeX] [PDF]@InProceedings{palazzolo2017uavg, title = {Information-Driven Autonomous Exploration for a Vision-Based MAV}, author = {E. Palazzolo and C. Stachniss}, booktitle = {ISPRS Annals of the Photogrammetry, Remote Sensing and Spatial Information Sciences}, year = {2017}, url = {https://www.ipb.uni-bonn.de/pdfs/palazzolo2017uavg.pdf}, }
- E. Palazzolo and C. Stachniss, “Change Detection in 3D Models Based on Camera Images,” in 9th Workshop on Planning, Perception and Navigation for Intelligent Vehicles at the IEEE/RSJ Int. Conf. on Intelligent Robots and Systems (IROS), 2017.
[BibTeX] [PDF]@InProceedings{palazzolo2017irosws, title = {Change Detection in 3D Models Based on Camera Images}, author = {E. Palazzolo and C. Stachniss}, booktitle = {9th Workshop on Planning, Perception and Navigation for Intelligent Vehicles at the IEEE/RSJ Int. Conf. on Intelligent Robots and Systems (IROS)}, year = {2017}, url = {https://www.ipb.uni-bonn.de/pdfs/palazzolo2017irosws}, }
- J. Schneider, C. Stachniss, and W. Förstner, “On the Quality and Efficiency of Approximate Solutions to Bundle Adjustment with Epipolar and Trifocal Constraints,” in ISPRS Annals of Photogrammetry, Remote Sensing and Spatial Information Sciences, 2017, pp. 81-88. doi:10.5194/isprs-annals-IV-2-W3-81-2017
[BibTeX] [PDF]
Bundle adjustment is a central part of most visual SLAM and Structure from Motion systems and thus a relevant component of UAVs equipped with cameras. This paper makes two contributions to bundle adjustment. First, we present a novel approach which exploits trifocal constraints, i.e., constraints resulting from corresponding points observed in three camera images, which allows to estimate the camera pose parameters without 3D point estimation. Second, we analyze the quality loss compared to the optimal bundle adjustment solution when applying different types of approximations to the constrained optimization problem to increase efficiency. We implemented and thoroughly evaluated our approach using a UAV performing mapping tasks in outdoor environments. Our results indicate that the complexity of the constraint bundle adjustment can be decreased without loosing too much accuracy.
@InProceedings{schneider2017uavg, title = {On the Quality and Efficiency of Approximate Solutions to Bundle Adjustment with Epipolar and Trifocal Constraints}, author = {J. Schneider and C. Stachniss and W. F\"orstner}, booktitle = {ISPRS Annals of Photogrammetry, Remote Sensing and Spatial Information Sciences}, year = {2017}, pages = {81-88}, volume = {IV-2/W3}, abstract = {Bundle adjustment is a central part of most visual SLAM and Structure from Motion systems and thus a relevant component of UAVs equipped with cameras. This paper makes two contributions to bundle adjustment. First, we present a novel approach which exploits trifocal constraints, i.e., constraints resulting from corresponding points observed in three camera images, which allows to estimate the camera pose parameters without 3D point estimation. Second, we analyze the quality loss compared to the optimal bundle adjustment solution when applying different types of approximations to the constrained optimization problem to increase efficiency. We implemented and thoroughly evaluated our approach using a UAV performing mapping tasks in outdoor environments. Our results indicate that the complexity of the constraint bundle adjustment can be decreased without loosing too much accuracy.}, doi = {10.5194/isprs-annals-IV-2-W3-81-2017}, url = {https://www.isprs-ann-photogramm-remote-sens-spatial-inf-sci.net/IV-2-W3/81/2017/isprs-annals-IV-2-W3-81-2017.pdf}, }
- O. Vysotska and C. Stachniss, “Improving SLAM by Exploiting Building Information from Publicly Available Maps and Localization Priors,” Journal of Photogrammetry, Remote Sensing and Geoinformation Science (PFG), vol. 85, iss. 1, pp. 53-65, 2017.
[BibTeX] [PDF] [Video]@Article{vysotska2017pfg, title = {Improving SLAM by Exploiting Building Information from Publicly Available Maps and Localization Priors}, author = {Vysotska, O. and Stachniss, C.}, journal = pfg, year = {2017}, number = {1}, pages = {53-65}, volume = {85}, url = {https://www.ipb.uni-bonn.de/pdfs/vysotska2016pfg.pdf}, videourl = {https://www.youtube.com/watch?v=dKHlF3OkEV4}, }
- O. Vysotska and C. Stachniss, “Relocalization under Substantial Appearance Changes using Hashing,” in IROS Workshop on Planning, Perception and Navigation for Intelligent Vehicles, 2017.
[BibTeX] [PDF] [Code]
[none]
@InProceedings{vysotska2017irosws, title = {Relocalization under Substantial Appearance Changes using Hashing}, author = {O. Vysotska and C. Stachniss}, booktitle = {IROS Workshop on Planning, Perception and Navigation for Intelligent Vehicles}, year = {2017}, abstract = {[none]}, url = {https://www.ipb.uni-bonn.de/pdfs/vysotska2017irosws.pdf}, codeurl = {https://github.com/Photogrammetry-Robotics-Bonn/vpr_relocalization}, }
- J. Jung, C. Stachniss, and C. Kim, “Automatic room segmentation of 3D laser data using morphological processing,” ISPRS International Journal of Geo-Information, 2017.
[BibTeX] [PDF]@Article{jung2017ijgi, author = {J. Jung and C. Stachniss and C. Kim}, title = {Automatic room segmentation of 3D laser data using morphological processing}, journal = {ISPRS International Journal of Geo-Information}, year = {2017}, url = {https://www.mdpi.com/2220-9964/6/7/206}, }
- R. Schirmer, P. Biber, and C. Stachniss, “Efficient Path Planning in Belief Space for Safe Navigation,” in Proc. of the IEEE/RSJ Intl. Conf. on Intelligent Robots and Systems (IROS), 2017.
[BibTeX] [PDF]
Robotic lawn-mowers are required to stay within a predefined working area, otherwise they may drive into a pond or on the street. This turns navigation and path planning into safety critical components. If we consider using SLAM techniques in that context, we must be able to provide safety guarantees in the presence of sensor/actuator noise and featureless areas in the environment. In this paper, we tackle the problem of planning a path that maximizes robot safety while navigating inside the working area and under the constraints of limited computing resources and cheap sensors. Our approach uses a map of the environment to estimate localizability at all locations, and it uses these estimates to search for a path from start to goal in belief space using an extended heuristic search algorithm. We implemented our approach using C++ and ROS and thoroughly tested it on simulation data recorded on eight different gardens, as well as on a real robot. The experiments presented in this paper show that our approach leads to short computation times and short paths while maximizing robot safety under certain assumptions.
@InProceedings{schirmer2017iros, author = {R. Schirmer and P. Biber and C. Stachniss}, title = {Efficient Path Planning in Belief Space for Safe Navigation}, booktitle = iros, year = {2017}, abstract = {Robotic lawn-mowers are required to stay within a predefined working area, otherwise they may drive into a pond or on the street. This turns navigation and path planning into safety critical components. If we consider using SLAM techniques in that context, we must be able to provide safety guarantees in the presence of sensor/actuator noise and featureless areas in the environment. In this paper, we tackle the problem of planning a path that maximizes robot safety while navigating inside the working area and under the constraints of limited computing resources and cheap sensors. Our approach uses a map of the environment to estimate localizability at all locations, and it uses these estimates to search for a path from start to goal in belief space using an extended heuristic search algorithm. We implemented our approach using C++ and ROS and thoroughly tested it on simulation data recorded on eight different gardens, as well as on a real robot. The experiments presented in this paper show that our approach leads to short computation times and short paths while maximizing robot safety under certain assumptions.}, url = {https://www.ipb.uni-bonn.de/pdfs/schirmer17iros.pdf}, }
- K. H. Huang and C. Stachniss, “Extrinsic Multi-Sensor Calibration For Mobile Robots Using the Gauss-Helmert Model,” in Proc. of the IEEE/RSJ Intl. Conf. on Intelligent Robots and Systems (IROS), 2017.
[BibTeX] [PDF]@InProceedings{huang2017iros, author = {K.H. Huang and C. Stachniss}, title = {Extrinsic Multi-Sensor Calibration For Mobile Robots Using the Gauss-Helmert Model}, booktitle = iros, year = 2017, url = {https://www.ipb.uni-bonn.de/pdfs/huang2017iros.pdf}, }
2016
- N. Abdo, C. Stachniss, L. Spinello, and W. Burgard, “Organizing Objects by Predicting User Preferences Through Collaborative Filtering,” The Intl. Journal of Robotics Research, 2016.
[BibTeX] [PDF]
[none]
@Article{abdo16ijrr, title = {Organizing Objects by Predicting User Preferences Through Collaborative Filtering}, author = {N. Abdo and C. Stachniss and L. Spinello and W. Burgard}, journal = ijrr, year = {2016}, note = {arXiv:1512.06362}, abstract = {[none]}, url = {https://arxiv.org/abs/1512.06362}, }
- C. Beekmans, J. Schneider, T. Läbe, M. Lennefer, C. Stachniss, and C. Simmer, “Cloud Photogrammetry with Dense Stereo for Fisheye Cameras,” Atmospheric Chemistry and Physics (ACP), vol. 16, iss. 22, pp. 14231-14248, 2016. doi:10.5194/acp-16-14231-2016
[BibTeX] [PDF]
We present a novel approach for dense 3-D cloud reconstruction above an area of 10 × 10 km2 using two hemispheric sky imagers with fisheye lenses in a stereo setup. We examine an epipolar rectification model designed for fisheye cameras, which allows the use of efficient out-of-the-box dense matching algorithms designed for classical pinhole-type cameras to search for correspondence information at every pixel. The resulting dense point cloud allows to recover a detailed and more complete cloud morphology compared to previous approaches that employed sparse feature-based stereo or assumed geometric constraints on the cloud field. Our approach is very efficient and can be fully automated. From the obtained 3-D shapes, cloud dynamics, size, motion, type and spacing can be derived, and used for radiation closure under cloudy conditions, for example. Fisheye lenses follow a different projection function than classical pinhole-type cameras and provide a large field of view with a single image. However, the computation of dense 3-D information is more complicated and standard implementations for dense 3-D stereo reconstruction cannot be easily applied. Together with an appropriate camera calibration, which includes internal camera geometry, global position and orientation of the stereo camera pair, we use the correspondence information from the stereo matching for dense 3-D stereo reconstruction of clouds located around the cameras. We implement and evaluate the proposed approach using real world data and present two case studies. In the first case, we validate the quality and accuracy of the method by comparing the stereo reconstruction of a stratocumulus layer with reflectivity observations measured by a cloud radar and the cloud-base height estimated from a Lidar-ceilometer. The second case analyzes a rapid cumulus evolution in the presence of strong wind shear.
@Article{beekmans16acp, title = {Cloud Photogrammetry with Dense Stereo for Fisheye Cameras}, author = {C. Beekmans and J. Schneider and T. L\"abe and M. Lennefer and C. Stachniss and C. Simmer}, journal = {Atmospheric Chemistry and Physics (ACP)}, year = {2016}, number = {22}, pages = {14231-14248}, volume = {16}, abstract = {We present a novel approach for dense 3-D cloud reconstruction above an area of 10 × 10 km2 using two hemispheric sky imagers with fisheye lenses in a stereo setup. We examine an epipolar rectification model designed for fisheye cameras, which allows the use of efficient out-of-the-box dense matching algorithms designed for classical pinhole-type cameras to search for correspondence information at every pixel. The resulting dense point cloud allows to recover a detailed and more complete cloud morphology compared to previous approaches that employed sparse feature-based stereo or assumed geometric constraints on the cloud field. Our approach is very efficient and can be fully automated. From the obtained 3-D shapes, cloud dynamics, size, motion, type and spacing can be derived, and used for radiation closure under cloudy conditions, for example. Fisheye lenses follow a different projection function than classical pinhole-type cameras and provide a large field of view with a single image. However, the computation of dense 3-D information is more complicated and standard implementations for dense 3-D stereo reconstruction cannot be easily applied. Together with an appropriate camera calibration, which includes internal camera geometry, global position and orientation of the stereo camera pair, we use the correspondence information from the stereo matching for dense 3-D stereo reconstruction of clouds located around the cameras. We implement and evaluate the proposed approach using real world data and present two case studies. In the first case, we validate the quality and accuracy of the method by comparing the stereo reconstruction of a stratocumulus layer with reflectivity observations measured by a cloud radar and the cloud-base height estimated from a Lidar-ceilometer. The second case analyzes a rapid cumulus evolution in the presence of strong wind shear.}, doi = {10.5194/acp-16-14231-2016}, url = {https://www.ipb.uni-bonn.de/pdfs/beekmans16acp.pdf}, }
- I. Bogoslavskyi, M. Mazuran, and C. Stachniss, “Robust Homing for Autonomous Robots,” in Proc. of the IEEE Intl. Conf. on Robotics & Automation (ICRA), 2016.
[BibTeX] [PDF] [Video]
[none]
@InProceedings{bogoslavskyi16icra, title = {Robust Homing for Autonomous Robots}, author = {I. Bogoslavskyi and M. Mazuran and C. Stachniss}, booktitle = icra, year = {2016}, abstract = {[none]}, url = {https://www.ipb.uni-bonn.de/pdfs/bogoslavskyi16icra.pdf}, videourl = {https://www.youtube.com/watch?v=sUvDvq91Vpw}, }
- I. Bogoslavskyi and C. Stachniss, “Fast Range Image-Based Segmentation of Sparse 3D Laser Scans for Online Operation,” in Proc. of the IEEE/RSJ Intl. Conf. on Intelligent Robots and Systems (IROS), 2016.
[BibTeX] [PDF] [Code] [Video]
[none]
@InProceedings{bogoslavskyi16iros, title = {Fast Range Image-Based Segmentation of Sparse 3D Laser Scans for Online Operation}, author = {I. Bogoslavskyi and C. Stachniss}, booktitle = iros, year = {2016}, abstract = {[none]}, url = {https://www.ipb.uni-bonn.de/pdfs/bogoslavskyi16iros.pdf}, codeurl = {https://github.com/Photogrammetry-Robotics-Bonn/depth_clustering}, videourl = {https://www.youtube.com/watch?v=6WqsOlHGTLA}, }
- F. Liebisch, J. Pfeifer, R. Khanna, P. Lottes, C. Stachniss, T. Falck, S. Sander, R. Siegwart, A. Walter, and E. Galceran, “Flourish – A robotic approach for automation in crop management,” in Proc. of the Workshop für Computer-Bildanalyse und unbemannte autonom fliegende Systeme in der Landwirtschaft, 2016.
[BibTeX] [PDF]@InProceedings{liebisch16wslw, title = {Flourish -- A robotic approach for automation in crop management}, author = {F. Liebisch and J. Pfeifer and R. Khanna and P. Lottes and C. Stachniss and T. Falck and S. Sander and R. Siegwart and A. Walter and E. Galceran}, booktitle = {Proc. of the Workshop f\"ur Computer-Bildanalyse und unbemannte autonom fliegende Systeme in der Landwirtschaft}, year = {2016}, timestamp = {2016.06.15}, url = {https://www.ipb.uni-bonn.de/wp-content/papercite-data/pdf/liebisch16cbaws.pdf}, }
- C. Stachniss, J. Leonard, and S. Thrun, “Springer Handbook of Robotics, 2nd edition,” , B. Siciliano and O. Khatib, Eds., Springer, 2016.
[BibTeX]@InBook{springerbook-slamchapter, author = {C. Stachniss and J. Leonard and S. Thrun}, editor = {B. Siciliano and O. Khatib}, title = {Springer Handbook of Robotics, 2nd edition}, chapter = {Chapt.~46: Simultaneous Localization and Mapping}, publisher = {Springer}, year = 2016, }
- P. Lottes, M. Höferlin, S. Sander, M. Müter, P. Schulze-Lammers, and C. Stachniss, “An Effective Classification System for Separating Sugar Beets and Weeds for Precision Farming Applications,” in Proc. of the IEEE Intl. Conf. on Robotics & Automation (ICRA), 2016.
[BibTeX] [PDF]@InProceedings{lottes2016icra, title = {An Effective Classification System for Separating Sugar Beets and Weeds for Precision Farming Applications}, author = {P. Lottes and M. H\"oferlin and S. Sander and M. M\"uter and P. Schulze-Lammers and C. Stachniss}, booktitle = icra, year = {2016}, timestamp = {2016.01.15}, url = {https://www.ipb.uni-bonn.de/wp-content/papercite-data/pdf/lottes16icra.pdf}, }
- C. Merfels and C. Stachniss, “Pose Fusion with Chain Pose Graphs for Automated Driving,” in Proc. of the IEEE/RSJ Intl. Conf. on Intelligent Robots and Systems (IROS), 2016.
[BibTeX] [PDF]@InProceedings{merfels16iros, title = {Pose Fusion with Chain Pose Graphs for Automated Driving}, author = {Ch. Merfels and C. Stachniss}, booktitle = iros, year = {2016}, url = {https://www.ipb.uni-bonn.de/pdfs/merfels16iros.pdf}, }
- L. Nardi and C. Stachniss, “Experience-Based Path Planning for Mobile Robots Exploiting User Preferences,” in Proc. of the IEEE/RSJ Intl. Conf. on Intelligent Robots and Systems (IROS), 2016. doi:10.1109/IROS.2016.7759197
[BibTeX] [PDF]
The demand for flexible industrial robotic solutions that are able to accomplish tasks at different locations in a factory is growing more and more. When deploying mobile robots in a factory environment, the predictability and reproducibility of their behaviors become important and are often requested. In this paper, we propose an easy-to-use motion planning scheme that can take into account user preferences for robot navigation. The preferences are extracted implicitly from the previous experiences or from demonstrations and are automatically considered in the subsequent planning steps. This leads to reproducible and thus better to predict navigation behaviors of the robot, without requiring experts to hard-coding control strategies or cost functions within a planner. Our system has been implemented and evaluated on a simulated KUKA mobile robot in different environments.
@InProceedings{nardi16iros, title = {Experience-Based Path Planning for Mobile Robots Exploiting User Preferences}, author = {L. Nardi and C. Stachniss}, booktitle = iros, year = {2016}, doi = {10.1109/IROS.2016.7759197}, abstract = {The demand for flexible industrial robotic solutions that are able to accomplish tasks at different locations in a factory is growing more and more. When deploying mobile robots in a factory environment, the predictability and reproducibility of their behaviors become important and are often requested. In this paper, we propose an easy-to-use motion planning scheme that can take into account user preferences for robot navigation. The preferences are extracted implicitly from the previous experiences or from demonstrations and are automatically considered in the subsequent planning steps. This leads to reproducible and thus better to predict navigation behaviors of the robot, without requiring experts to hard-coding control strategies or cost functions within a planner. Our system has been implemented and evaluated on a simulated KUKA mobile robot in different environments.}, url = {https://www.ipb.uni-bonn.de/pdfs/nardi16iros.pdf}, }
- S. Osswald, M. Bennewitz, W. Burgard, and C. Stachniss, “Speeding-Up Robot Exploration by Exploiting Background Information,” IEEE Robotics and Automation Letters (RA-L), 2016.
[BibTeX] [PDF]@Article{osswald16ral, title = {Speeding-Up Robot Exploration by Exploiting Background Information}, author = {S. Osswald and M. Bennewitz and W. Burgard and C. Stachniss}, journal = ral, year = {2016}, url = {https://www.ipb.uni-bonn.de/wp-content/papercite-data/pdf/osswald16ral.pdf}, }
- D. Perea-Ström, I. Bogoslavskyi, and C. Stachniss, “Robust Exploration and Homing for Autonomous Robots,” in Robotics and Autonomous Systems, 2016.
[BibTeX] [PDF]@InProceedings{perea16jras, title = {Robust Exploration and Homing for Autonomous Robots}, author = {D. Perea-Str{\"o}m and I. Bogoslavskyi and C. Stachniss}, booktitle = jras, year = {2016}, url = {https://www.ipb.uni-bonn.de/wp-content/papercite-data/pdf/perea16jras.pdf}, }
- J. Schneider, C. Eling, L. Klingbeil, H. Kuhlmann, W. Förstner, and C. Stachniss, “Fast and Effective Online Pose Estimation and Mapping for UAVs,” in Proc. of the IEEE Intl. Conf. on Robotics & Automation (ICRA), 2016, p. 4784–4791. doi:10.1109/ICRA.2016.7487682
[BibTeX] [PDF]
Online pose estimation and mapping in unknown environments is essential for most mobile robots. Especially autonomous unmanned aerial vehicles require good pose estimates at comparably high frequencies. In this paper, we propose an effective system for online pose and simultaneous map estimation designed for light-weight UAVs. Our system consists of two components: (1) real-time pose estimation combining RTK-GPS and IMU at 100 Hz and (2) an effective SLAM solution running at 10 Hz using image data from an omnidirectional multi-fisheye-camera system. The SLAM procedure combines spatial resection computed based on the map that is incrementally refined through bundle adjustment and combines the image data with raw GPS observations and IMU data on keyframes. The overall system yields a real-time, georeferenced pose at 100 Hz in GPS-friendly situations. Additionally, we obtain a precise pose and feature map at 10 Hz even in cases where the GPS is not observable or underconstrained. Our system has been implemented and thoroughly tested on a 5 kg copter and yields accurate and reliable pose estimation at high frequencies. We compare the point cloud obtained by our method with a model generated from georeferenced terrestrial laser scanner.
@InProceedings{schneider16icra, title = {Fast and Effective Online Pose Estimation and Mapping for UAVs}, author = {J. Schneider and C. Eling and L. Klingbeil and H. Kuhlmann and W. F\"orstner and C. Stachniss}, booktitle = icra, year = {2016}, pages = {4784--4791}, abstract = {Online pose estimation and mapping in unknown environments is essential for most mobile robots. Especially autonomous unmanned aerial vehicles require good pose estimates at comparably high frequencies. In this paper, we propose an effective system for online pose and simultaneous map estimation designed for light-weight UAVs. Our system consists of two components: (1) real-time pose estimation combining RTK-GPS and IMU at 100 Hz and (2) an effective SLAM solution running at 10 Hz using image data from an omnidirectional multi-fisheye-camera system. The SLAM procedure combines spatial resection computed based on the map that is incrementally refined through bundle adjustment and combines the image data with raw GPS observations and IMU data on keyframes. The overall system yields a real-time, georeferenced pose at 100 Hz in GPS-friendly situations. Additionally, we obtain a precise pose and feature map at 10 Hz even in cases where the GPS is not observable or underconstrained. Our system has been implemented and thoroughly tested on a 5 kg copter and yields accurate and reliable pose estimation at high frequencies. We compare the point cloud obtained by our method with a model generated from georeferenced terrestrial laser scanner.}, doi = {10.1109/ICRA.2016.7487682}, url = {https://www.ipb.uni-bonn.de/pdfs/schneider16icra.pdf}, }
- J. Schneider, C. Stachniss, and W. Förstner, “Dichtes Stereo mit Fisheye-Kameras,” in UAV 2016 – Vermessung mit unbemannten Flugsystemen, 2016, pp. 247-264.
[BibTeX]@InProceedings{schneider16dvw, title = {Dichtes Stereo mit Fisheye-Kameras}, author = {J. Schneider and C. Stachniss and W. F\"orstner}, booktitle = {UAV 2016 -- Vermessung mit unbemannten Flugsystemen}, year = {2016}, pages = {247-264}, publisher = {Wi{\ss}ner Verlag}, series = {Schriftenreihe des DVW}, volume = {82}, }
- J. Schneider, C. Stachniss, and W. Förstner, “On the Accuracy of Dense Fisheye Stereo,” IEEE Robotics and Automation Letters (RA-L), vol. 1, iss. 1, pp. 227-234, 2016. doi:10.1109/LRA.2016.2516509
[BibTeX] [PDF]
Fisheye cameras offer a large field of view, which is important for several robotics applications as a larger field of view allows for covering a large area with a single image. In contrast to classical cameras, however, fisheye cameras cannot be approximated well using the pinhole camera model and this renders the computation of depth information from fisheye stereo image pairs more complicated. In this work, we analyze the combination of an epipolar rectification model for fisheye stereo cameras with existing dense methods. This has the advantage that existing dense stereo systems can be applied as a black-box even with cameras that have field of view of more than 180 deg to obtain dense disparity information. We thoroughly investigate the accuracy potential of such fisheye stereo systems using image data from our UAV. The empirical analysis is based on image pairs of a calibrated fisheye stereo camera system and two state-of-the-art algorithms for dense stereo applied to adequately rectified image pairs from fisheye stereo cameras. The canonical stochastic model for sensor points assumes homogeneous uncertainty and we generalize this model based on an empirical analysis using a test scene consisting of mutually orthogonal planes. We show (1) that the combination of adequately rectified fisheye image pairs and dense methods provides dense 3D point clouds at 6-7 Hz on our autonomous multi-copter UAV, (2) that the uncertainty of points depends on their angular distance from the optical axis, (3) how to estimate the variance component as a function of that distance, and (4) how the improved stochastic model improves the accuracy of the scene points.
@Article{schneider16ral, title = {On the Accuracy of Dense Fisheye Stereo}, author = {J. Schneider and C. Stachniss and W. F\"orstner}, journal = ral, year = {2016}, number = {1}, pages = {227-234}, volume = {1}, abstract = {Fisheye cameras offer a large field of view, which is important for several robotics applications as a larger field of view allows for covering a large area with a single image. In contrast to classical cameras, however, fisheye cameras cannot be approximated well using the pinhole camera model and this renders the computation of depth information from fisheye stereo image pairs more complicated. In this work, we analyze the combination of an epipolar rectification model for fisheye stereo cameras with existing dense methods. This has the advantage that existing dense stereo systems can be applied as a black-box even with cameras that have field of view of more than 180 deg to obtain dense disparity information. We thoroughly investigate the accuracy potential of such fisheye stereo systems using image data from our UAV. The empirical analysis is based on image pairs of a calibrated fisheye stereo camera system and two state-of-the-art algorithms for dense stereo applied to adequately rectified image pairs from fisheye stereo cameras. The canonical stochastic model for sensor points assumes homogeneous uncertainty and we generalize this model based on an empirical analysis using a test scene consisting of mutually orthogonal planes. We show (1) that the combination of adequately rectified fisheye image pairs and dense methods provides dense 3D point clouds at 6-7 Hz on our autonomous multi-copter UAV, (2) that the uncertainty of points depends on their angular distance from the optical axis, (3) how to estimate the variance component as a function of that distance, and (4) how the improved stochastic model improves the accuracy of the scene points.}, doi = {10.1109/LRA.2016.2516509}, url = {https://www.ipb.uni-bonn.de/pdfs/schneider16ral.pdf}, }
- T. Schubert, S. Wenzel, R. Roscher, and C. Stachniss, “Investigation of Latent Traces Using Infrared Reflectance Hyperspectral Imaging,” in ISPRS Annals of Photogrammetry, Remote Sensing and Spatial Information Sciences, 2016, p. 97–102. doi:10.5194/isprs-annals-III-7-97-2016
[BibTeX] [PDF]
The detection of traces is a main task of forensic science. A potential method is hyperspectral imaging (HSI) from which we expect to capture more fluorescence effects than with common Forensic Light Sources (FLS). Specimen of blood, semen and saliva traces in several dilution steps are prepared on cardboard substrate. As our key result we successfully make latent traces visible up to highest available dilution (1:8000). We can attribute most of the detectability to interference of electromagnetic light with the water content of the traces in the Shortwave Infrared region of the spectrum. In a classification task we use several dimensionality reduction methods (PCA and LDA) in combination with a Maximum Likelihood (ML) classifier assuming normally distributed data. Random Forest builds a competitive approach. The classifiers retrieve the exact positions of labeled trace preparation up to highest dilution and determine posterior probabilities. By modeling the classification with a Markov Random Field we obtain smoothed results.
@InProceedings{schubert2016investigation, title = {{Investigation of Latent Traces Using Infrared Reflectance Hyperspectral Imaging}}, author = {Schubert, Till and Wenzel, Susanne and Roscher, Ribana and Stachniss, Cyrill}, booktitle = {ISPRS Annals of Photogrammetry, Remote Sensing and Spatial Information Sciences}, year = {2016}, pages = {97--102}, volume = {III-7}, abstract = {The detection of traces is a main task of forensic science. A potential method is hyperspectral imaging (HSI) from which we expect to capture more fluorescence effects than with common Forensic Light Sources (FLS). Specimen of blood, semen and saliva traces in several dilution steps are prepared on cardboard substrate. As our key result we successfully make latent traces visible up to highest available dilution (1:8000). We can attribute most of the detectability to interference of electromagnetic light with the water content of the traces in the Shortwave Infrared region of the spectrum. In a classification task we use several dimensionality reduction methods (PCA and LDA) in combination with a Maximum Likelihood (ML) classifier assuming normally distributed data. Random Forest builds a competitive approach. The classifiers retrieve the exact positions of labeled trace preparation up to highest dilution and determine posterior probabilities. By modeling the classification with a Markov Random Field we obtain smoothed results.}, doi = {10.5194/isprs-annals-III-7-97-2016}, url = {https://www.ipb.uni-bonn.de/pdfs/Schubert2016Investigation.pdf}, }
- C. Siedentop, V. Laukhart, B. Krastev, D. Kasper, A. Wenden, G. Breuel, and C. Stachniss, “Autonomous Parking Using Previous Paths,” in Advanced Microsystems for Automotive Applications 2015: Smart Systems for Green and Automated Driving. Lecture Notes in Mobility, T. Schulze, B. Müller, and G. Meyer, Eds., Springer, 2016, pp. 3-14. doi:10.1007/978-3-319-20855-8_1
[BibTeX]@InBook{siedentop16lnb, title = {Autonomous Parking Using Previous Paths}, author = {C. Siedentop and V. Laukhart and B. Krastev and D. Kasper and A. Wenden and G. Breuel and C. Stachniss}, editor = {T. Schulze and B. M{\"u}ller and G. Meyer}, pages = {3-14}, publisher = {Springer}, year = {2016}, booktitle = {Advanced Microsystems for Automotive Applications 2015: Smart Systems for Green and Automated Driving. Lecture Notes in Mobility}, doi = {10.1007/978-3-319-20855-8_1}, }
- C. Stachniss, “Springer Handbook of Photogrammetry.” Springer, 2016.
[BibTeX]@InBook{springerbook-photo-slamchapter, author = {C. Stachniss}, title = {Springer Handbook of Photogrammetry}, chapter = {Simultaneous Localization and Mapping}, publisher = {Springer}, note = {In German}, year = {2016}, }
- O. Vysotska and C. Stachniss, “Exploiting Building Information from Publicly Available Maps in Graph-Based SLAM,” in Proc. of the IEEE/RSJ Intl. Conf. on Intelligent Robots and Systems (IROS), 2016.
[BibTeX] [PDF] [Video]
[none]
@InProceedings{vysotska16iros, title = {Exploiting Building Information from Publicly Available Maps in Graph-Based SLAM}, author = {O. Vysotska and C. Stachniss}, booktitle = iros, year = {2016}, abstract = {[none]}, url = {https://www.ipb.uni-bonn.de/pdfs/vysotska16iros.pdf}, videourl = {https://www.youtube.com/watch?v=5RfRAEP-baM}, }
- O. Vysotska and C. Stachniss, “Lazy Data Association For Image Sequences Matching Under Substantial Appearance Changes,” IEEE Robotics and Automation Letters (RA-L), vol. 1, iss. 1, pp. 213-220, 2016. doi:10.1109/LRA.2015.2512936
[BibTeX] [PDF] [Code] [Video]
Localization is an essential capability for mobile robots and the ability to localize in changing environments is key to robust outdoor navigation. Robots operating over extended periods of time should be able to handle substantial appearance changes such as those occurring over seasons or under different weather conditions. In this letter, we investigate the problem of efficiently coping with seasonal appearance changes in online localization. We propose a lazy data association approach for matching streams of incoming images to a reference image sequence in an online fashion. We present a search heuristic to quickly find matches between the current image sequence and a database using a data association graph. Our experiments conducted under substantial seasonal changes suggest that our approach can efficiently match image sequences while requiring a comparably small number of image to image comparisons
@Article{vysotska16ral, title = {Lazy Data Association For Image Sequences Matching Under Substantial Appearance Changes}, author = {O. Vysotska and C. Stachniss}, journal = ral, year = {2016}, number = {1}, pages = {213-220}, volume = {1}, abstract = {Localization is an essential capability for mobile robots and the ability to localize in changing environments is key to robust outdoor navigation. Robots operating over extended periods of time should be able to handle substantial appearance changes such as those occurring over seasons or under different weather conditions. In this letter, we investigate the problem of efficiently coping with seasonal appearance changes in online localization. We propose a lazy data association approach for matching streams of incoming images to a reference image sequence in an online fashion. We present a search heuristic to quickly find matches between the current image sequence and a database using a data association graph. Our experiments conducted under substantial seasonal changes suggest that our approach can efficiently match image sequences while requiring a comparably small number of image to image comparisons}, doi = {10.1109/LRA.2015.2512936}, timestamp = {2016.04.18}, url = {https://www.ipb.uni-bonn.de/pdfs/vysotska16ral-icra.pdf}, codeurl = {https://github.com/Photogrammetry-Robotics-Bonn/online_place_recognition}, videourl = {https://www.youtube.com/watch?v=l-hNk7Z4lSk}, }
- C. Merfels, T. Riemenschneider, and C. Stachniss, “Pose fusion with biased and dependent data for automated driving,” in Proc. of the Positioning and Navigation for Intelligent Transportation Systems Conf. (POSNAV ITS), 2016.
[BibTeX] [PDF]@InProceedings{merfels2016posnav, author = {C. Merfels and T. Riemenschneider and C. Stachniss}, title = {Pose fusion with biased and dependent data for automated driving}, booktitle = {Proc. of the Positioning and Navigation for Intelligent Transportation Systems Conf. (POSNAV ITS)}, year = 2016, }
2015
- N. Abdo, C. Stachniss, L. Spinello, and W. Burgard, “Collaborative Filtering for Predicting User Preferences for Organizing Objects,” arXiv Preprint, vol. abs/1512.06362, 2015.
[BibTeX] [PDF]
[none]
@Article{abdo15arxiv, title = {Collaborative Filtering for Predicting User Preferences for Organizing Objects}, author = {N. Abdo and C. Stachniss and L. Spinello and W. Burgard}, journal = arxiv, year = {2015}, note = {arXiv:1512.06362 [cs.RO]}, volume = {abs/1512.06362}, abstract = {[none]}, timestamp = {2016.04.18}, url = {https://arxiv.org/abs/1512.06362}, }
- N. Abdo, C. Stachniss, L. Spinello, and W.Burgard, “Robot, Organize my Shelves! Tidying up Objects by Predicting User Preferences,” in Proc. of the IEEE Intl. Conf. on Robotics & Automation (ICRA), 2015, pp. 1557-1564. doi:10.1109/ICRA.2015.7139396
[BibTeX] [PDF]
As service robots become more and more capable of performing useful tasks for us, there is a growing need to teach robots how we expect them to carry out these tasks. However, learning our preferences is a nontrivial problem, as many of them stem from a variety of factors including personal taste, cultural background, or common sense. Obviously, such factors are hard to formulate or model a priori. In this paper, we present a solution for tidying up objects in containers, e.g., shelves or boxes, by following user preferences. We learn the user preferences using collaborative filtering based on crowdsourced and mined data. First, we predict pairwise object preferences of the user. Then, we subdivide the objects in containers by modeling a spectral clustering problem. Our solution is easy to update, does not require complex modeling, and improves with the amount of user data. We evaluate our approach using crowdsoucing data from over 1,200 users and demonstrate its effectiveness for two tidy-up scenarios. Additionally, we show that a real robot can reliably predict user preferences using our approach.
@InProceedings{abdo15icra, title = {Robot, Organize my Shelves! Tidying up Objects by Predicting User Preferences}, author = {N. Abdo and C. Stachniss and L. Spinello and W.Burgard}, booktitle = icra, year = {2015}, pages = {1557-1564}, abstract = {As service robots become more and more capable of performing useful tasks for us, there is a growing need to teach robots how we expect them to carry out these tasks. However, learning our preferences is a nontrivial problem, as many of them stem from a variety of factors including personal taste, cultural background, or common sense. Obviously, such factors are hard to formulate or model a priori. In this paper, we present a solution for tidying up objects in containers, e.g., shelves or boxes, by following user preferences. We learn the user preferences using collaborative filtering based on crowdsourced and mined data. First, we predict pairwise object preferences of the user. Then, we subdivide the objects in containers by modeling a spectral clustering problem. Our solution is easy to update, does not require complex modeling, and improves with the amount of user data. We evaluate our approach using crowdsoucing data from over 1,200 users and demonstrate its effectiveness for two tidy-up scenarios. Additionally, we show that a real robot can reliably predict user preferences using our approach.}, doi = {10.1109/ICRA.2015.7139396}, url = {https://www.ipb.uni-bonn.de/wp-content/papercite-data/pdf/abdo15icra.pdf}, }
- I. Bogoslavskyi, L. Spinello, W. Burgard, and C. Stachniss, “Where to Park%3F Minimizing the Expected Time to Find a Parking Space,” in Proc. of the IEEE Intl. Conf. on Robotics & Automation (ICRA), 2015, pp. 2147-2152. doi:10.1109/ICRA.2015.7139482
[BibTeX] [PDF]
Quickly finding a free parking spot that is close to a desired target location can be a difficult task. This holds for human drivers and autonomous cars alike. In this paper, we investigate the problem of predicting the occupancy of parking spaces and exploiting this information during route planning. We propose an MDP-based planner that considers route information as well as the occupancy probabilities of parking spaces to compute the path that minimizes the expected total time for finding an unoccupied parking space and for walking from the parking location to the target destination. We evaluated our system on real world data gathered over several days in a real parking lot. We furthermore compare our approach to three parking strategies and show that our method outperforms the alternative behaviors.
@InProceedings{bogoslavskyi15icra, title = {Where to Park? Minimizing the Expected Time to Find a Parking Space}, author = {I. Bogoslavskyi and L. Spinello and W. Burgard and C. Stachniss}, booktitle = icra, year = {2015}, pages = {2147-2152}, abstract = {Quickly finding a free parking spot that is close to a desired target location can be a difficult task. This holds for human drivers and autonomous cars alike. In this paper, we investigate the problem of predicting the occupancy of parking spaces and exploiting this information during route planning. We propose an MDP-based planner that considers route information as well as the occupancy probabilities of parking spaces to compute the path that minimizes the expected total time for finding an unoccupied parking space and for walking from the parking location to the target destination. We evaluated our system on real world data gathered over several days in a real parking lot. We furthermore compare our approach to three parking strategies and show that our method outperforms the alternative behaviors.}, doi = {10.1109/ICRA.2015.7139482}, timestamp = {2015.06.29}, url = {https://www.ipb.uni-bonn.de/pdfs/bogoslavskyi15icra.pdf}, }
- T. Naseer, M. Ruhnke, L. Spinello, C. Stachniss, and W. Burgard, “Robust Visual SLAM Across Seasons,” in Proc. of the IEEE/RSJ Intl. Conf. on Intelligent Robots and Systems (IROS), 2015, pp. 2529-2535. doi:10.1109/IROS.2015.7353721
[BibTeX] [PDF]
In this paper, we present an appearance-based visual SLAM approach that focuses on detecting loop closures across seasons. Given two image sequences, our method first extracts one descriptor per image for both sequences using a deep convolutional neural network. Then, we compute a similarity matrix by comparing each image of a query sequence with a database. Finally, based on the similarity matrix, we formulate a flow network problem and compute matching hypotheses between sequences. In this way, our approach can handle partially matching routes, loops in the trajectory and different speeds of the robot. With a matching hypothesis as loop closure information and the odometry information of the robot, we formulate a graph based SLAM problem and compute a joint maximum likelihood trajectory.
@InProceedings{naseer15iros, title = {Robust Visual SLAM Across Seasons}, author = {Naseer, Tayyab and Ruhnke, Michael and Spinello, Luciano and Stachniss, Cyrill and Burgard, Wolfram}, booktitle = iros, year = {2015}, pages = {2529 - 2535}, abstract = {In this paper, we present an appearance-based visual SLAM approach that focuses on detecting loop closures across seasons. Given two image sequences, our method first extracts one descriptor per image for both sequences using a deep convolutional neural network. Then, we compute a similarity matrix by comparing each image of a query sequence with a database. Finally, based on the similarity matrix, we formulate a flow network problem and compute matching hypotheses between sequences. In this way, our approach can handle partially matching routes, loops in the trajectory and different speeds of the robot. With a matching hypothesis as loop closure information and the odometry information of the robot, we formulate a graph based SLAM problem and compute a joint maximum likelihood trajectory.}, doi = {10.1109/IROS.2015.7353721}, timestamp = {2016.04.19}, url = {https://www.ipb.uni-bonn.de/pdfs/Naseer2015Robust.pdf}, }
- D. Perea-Ström, F. Nenci, and C. Stachniss, “Predictive Exploration Considering Previously Mapped Environments,” in Proc. of the IEEE Intl. Conf. on Robotics & Automation (ICRA), 2015, pp. 2761-2766. doi:10.1109/ICRA.2015.7139574
[BibTeX] [PDF]
The ability to explore an unknown environment is an important prerequisite for building truly autonomous robots. The central decision that a robot needs to make when exploring an unknown environment is to select the next view point(s) for gathering observations. In this paper, we consider the problem of how to select view points that support the underlying mapping process. We propose a novel approach that makes predictions about the structure of the environments in the unexplored areas by relying on maps acquired previously. Our approach seeks to find similarities between the current surroundings of the robot and previously acquired maps stored in a database in order to predict how the environment may expand in the unknown areas. This allows us to predict potential future loop closures early. This knowledge is used in the view point selection to actively close loops and in this way reduce the uncertainty in the robot’s belief. We implemented and tested the proposed approach. The experiments indicate that our method improves the ability of a robot to explore challenging environments and improves the quality of the resulting maps.
@InProceedings{perea15icra, title = {Predictive Exploration Considering Previously Mapped Environments}, author = {D. Perea-Str{\"o}m and F. Nenci and C. Stachniss}, booktitle = icra, year = {2015}, pages = {2761-2766}, abstract = {The ability to explore an unknown environment is an important prerequisite for building truly autonomous robots. The central decision that a robot needs to make when exploring an unknown environment is to select the next view point(s) for gathering observations. In this paper, we consider the problem of how to select view points that support the underlying mapping process. We propose a novel approach that makes predictions about the structure of the environments in the unexplored areas by relying on maps acquired previously. Our approach seeks to find similarities between the current surroundings of the robot and previously acquired maps stored in a database in order to predict how the environment may expand in the unknown areas. This allows us to predict potential future loop closures early. This knowledge is used in the view point selection to actively close loops and in this way reduce the uncertainty in the robot's belief. We implemented and tested the proposed approach. The experiments indicate that our method improves the ability of a robot to explore challenging environments and improves the quality of the resulting maps.}, doi = {10.1109/ICRA.2015.7139574}, url = {https://www.ipb.uni-bonn.de/wp-content/papercite-data/pdf/perea15icra.pdf}, }
- C. Siedentop, R. Heinze, D. Kasper, G. Breuel, and C. Stachniss, “Path-Planning for Autonomous Parking with Dubins Curves,” in Proc. of the Workshop Fahrerassistenzsysteme, 2015.
[BibTeX] [PDF]@InProceedings{siedentop15fas, title = {Path-Planning for Autonomous Parking with Dubins Curves}, author = {C. Siedentop and R. Heinze and D. Kasper and G. Breuel and C. Stachniss}, booktitle = {Proc. of the Workshop Fahrerassistenzsysteme}, year = {2015}, }
- O. Vysotska, T. Naseer, L. Spinello, W. Burgard, and C. Stachniss, “Efficient and Effective Matching of Image Sequences Under Substantial Appearance Changes Exploiting GPS Prior,” in Proc. of the IEEE Intl. Conf. on Robotics & Automation (ICRA), 2015, pp. 2774-2779. doi:10.1109/ICRA.2015.7139576
[BibTeX] [PDF] [Code]@InProceedings{vysotska15icra, title = {Efficient and Effective Matching of Image Sequences Under Substantial Appearance Changes Exploiting GPS Prior}, author = {O. Vysotska and T. Naseer and L. Spinello and W. Burgard and C. Stachniss}, booktitle = icra, year = {2015}, pages = {2774-2779}, doi = {10.1109/ICRA.2015.7139576}, timestamp = {2015.06.29}, url = {https://www.ipb.uni-bonn.de/pdfs/vysotska15icra.pdf}, codeurl = {https://github.com/ovysotska/image_sequence_matcher}, }
- O. Vysotska and C. Stachniss, “Lazy Sequences Matching Under Substantial Appearance Changes,” in Workshop on Visual Place Recognition in Changing Environments at the IEEE Proc. of the IEEE Intl. Conf. on Robotics & Automation (ICRA), 2015.
[BibTeX] [PDF]
[none]
@InProceedings{vysotska15icraws, title = {Lazy Sequences Matching Under Substantial Appearance Changes}, author = {O. Vysotska and C. Stachniss}, booktitle = {Workshop on Visual Place Recognition in Changing Environments at the IEEE } # icra, year = {2015}, abstract = {[none]}, timestamp = {2015.06.29}, url = {https://www.ipb.uni-bonn.de/pdfs/vysotska15icra-ws.pdf}, }
2014
- B. Frank, C. Stachniss, R. Schmedding, M. Teschner, and W. Burgard, “Learning object deformation models for robot motion planning,” Robotics and Autonomous Systems, p. -, 2014. doi:https://dx.doi.org/10.1016/j.robot.2014.04.005
[BibTeX] [PDF]
[none]
@Article{frank2014, title = {Learning object deformation models for robot motion planning }, author = {Barbara Frank and Cyrill Stachniss and R\"{u}diger Schmedding and Matthias Teschner and Wolfram Burgard}, journal = {Robotics and Autonomous Systems }, year = {2014}, pages = { - }, abstract = {[none]}, crossref = {mn}, doi = {https://dx.doi.org/10.1016/j.robot.2014.04.005}, issn = {0921-8890}, keywords = {Mobile robots}, url = {https://www.sciencedirect.com/science/article/pii/S0921889014000797}, }
- N. Abdo, L. Spinello, W. Burgard, and C. Stachniss, “Inferring What to Imitate in Manipulation Actions by Using a Recommender System,” in Proc. of the IEEE Intl. Conf. on Robotics & Automation (ICRA), Hong Kong, China, 2014.
[BibTeX] [PDF]@InProceedings{abdo2014icra, title = {Inferring What to Imitate in Manipulation Actions by Using a Recommender System}, author = {N. Abdo and L. Spinello and W. Burgard and C. Stachniss}, booktitle = icra, year = {2014}, address = {Hong Kong, China}, }
- P. Agarwal, W. Burgard, and C. Stachniss, “Helmert’s and Bowie’s Geodetic Mapping Methods and Their Relation to Graph-Based SLAM,” in Proc. of the IEEE Intl. Conf. on Robotics & Automation (ICRA), Hong Kong, China, 2014.
[BibTeX]@InProceedings{agarwal2014icra, title = {Helmert's and Bowie's Geodetic Mapping Methods and Their Relation to Graph-Based SLAM}, author = {P. Agarwal and W. Burgard and C. Stachniss}, booktitle = icra, year = {2014}, address = {Hong Kong, China}, timestamp = {2014.04.24}, }
- P. Agarwal, W. Burgard, and C. Stachniss, “A Survey of Geodetic Approaches to Mapping and the Relationship to Graph-Based SLAM,” IEEE Robotics and Automation Magazine, vol. 21, pp. 63-80, 2014. doi:10.1109/MRA.2014.2322282
[BibTeX] [PDF]
The ability to simultaneously localize a robot and build a map of the environment is central to most robotics applications, and the problem is often referred to as simultaneous localization and mapping (SLAM). Robotics researchers have proposed a large variety of solutions allowing robots to build maps and use them for navigation. In addition, the geodetic community has addressed large-scale map building for centuries, computing maps that span across continents. These large-scale mapping processes had to deal with several challenges that are similar to those of the robotics community. In this article, we explain key geodetic map building methods that we believe are relevant for robot mapping. We also aim at providing a geodetic perspective on current state-of-the-art SLAM methods and identifying similarities both in terms of challenges faced and the solutions proposed by both communities. The central goal of this article is to connect both fields and enable future synergies between them.
@Article{agarwal2014ram, title = {A Survey of Geodetic Approaches to Mapping and the Relationship to Graph-Based SLAM}, author = {Pratik Agarwal and Wolfram Burgard and Cyrill Stachniss}, journal = {IEEE Robotics and Automation Magazine}, year = {2014}, pages = {63 - 80}, volume = {21}, abstract = {The ability to simultaneously localize a robot and build a map of the environment is central to most robotics applications, and the problem is often referred to as simultaneous localization and mapping (SLAM). Robotics researchers have proposed a large variety of solutions allowing robots to build maps and use them for navigation. In addition, the geodetic community has addressed large-scale map building for centuries, computing maps that span across continents. These large-scale mapping processes had to deal with several challenges that are similar to those of the robotics community. In this article, we explain key geodetic map building methods that we believe are relevant for robot mapping. We also aim at providing a geodetic perspective on current state-of-the-art SLAM methods and identifying similarities both in terms of challenges faced and the solutions proposed by both communities. The central goal of this article is to connect both fields and enable future synergies between them.}, doi = {10.1109/MRA.2014.2322282}, timestamp = {2014.09.18}, }
- P. Agarwal, G. Grisetti, G. D. Tipaldi, L. Spinello, W. Burgard, and C. Stachniss, “Experimental Analysis of Dynamic Covariance Scaling for Robust Map Optimization Under Bad Initial Estimates,” in Proc. of the IEEE Intl. Conf. on Robotics & Automation (ICRA), Hong Kong, China, 2014.
[BibTeX]
[none]
@InProceedings{agarwal2014-dcs, title = {Experimental Analysis of Dynamic Covariance Scaling for Robust Map Optimization Under Bad Initial Estimates}, author = {P. Agarwal and G. Grisetti and G.D. Tipaldi and L. Spinello and W. Burgard and C. Stachniss}, booktitle = icra, year = {2014}, address = {Hong Kong, China}, abstract = {[none]}, timestamp = {2014.04.24}, }
- S. Ito, F. Endres, M. Kuderer, G. D. Tipaldi, C. Stachniss, and W. Burgard, “W-RGB-D: Floor-Plan-Based Indoor Global Localization Using a Depth Camera and WiFi,” in Proc. of the IEEE Intl. Conf. on Robotics & Automation (ICRA), Hong Kong, China, 2014.
[BibTeX] [PDF]
[none]
@InProceedings{ito2014, title = {W-RGB-D: Floor-Plan-Based Indoor Global Localization Using a Depth Camera and WiFi}, author = {S. Ito and F. Endres and M. Kuderer and G.D. Tipaldi and C. Stachniss and W. Burgard}, booktitle = icra, year = {2014}, address = {Hong Kong, China}, abstract = {[none]}, timestamp = {2014.04.24}, url = {https://www2.informatik.uni-freiburg.de/~tipaldi/papers/ito14icra.pdf}, }
- R. Kümmerle, M. Ruhnke, B. Steder, C. Stachniss, and W. Burgard, “Autonomous Robot Navigation in Highly Populated Pedestrian Zones,” Journal of Field Robotics, 2014. doi:10.1002/rob.21534
[BibTeX] [PDF]
[none]
@Article{kummerle14jfr, title = {Autonomous Robot Navigation in Highly Populated Pedestrian Zones}, author = {K{\"u}mmerle, Rainer and Ruhnke, Michael and Steder, Bastian and Stachniss,Cyrill and Burgard, Wolfram}, journal = jfr, year = {2014}, abstract = {[none]}, doi = {10.1002/rob.21534}, timestamp = {2015.01.22}, url = {https://ais.informatik.uni-freiburg.de/publications/papers/kuemmerle14jfr.pdf}, }
- M. Mazuran, G. D. Tipaldi, L. Spinello, W. Burgard, and C. Stachniss, “A Statistical Measure for Map Consistency in SLAM,” in Proc. of the IEEE Intl. Conf. on Robotics & Automation (ICRA), Hong Kong, China, 2014.
[BibTeX] [PDF]@InProceedings{mazuran2014icra, title = {A Statistical Measure for Map Consistency in SLAM}, author = {M. Mazuran and G.D. Tipaldi and L. Spinello and W. Burgard and C. Stachniss}, booktitle = icra, year = {2014}, address = {Hong Kong, China}, timestamp = {2014.04.24}, }
- T. Naseer, L. Spinello, W. Burgard, and Stachniss, “Robust Visual Robot Localization Across Seasons using Network Flows,” in Proc. of the National Conf. on Artificial Intellience (AAAI), 2014.
[BibTeX] [PDF]
[none]
@InProceedings{naseer2014aaai, title = {Robust Visual Robot Localization Across Seasons using Network Flows}, author = {Naseer, T. and Spinello, L. and Burgard, W. and Stachniss}, booktitle = aaai, year = {2014}, abstract = {[none]}, timestamp = {2014.05.12}, }
- F. Nenci, L. Spinello, and C. Stachniss, “Effective Compression of Range Data Streams for Remote Robot Operations using H.264,” in Proc. of the IEEE/RSJ Intl. Conf. on Intelligent Robots and Systems (IROS), 2014.
[BibTeX] [PDF]@InProceedings{nenci2014iros, title = {Effective Compression of Range Data Streams for Remote Robot Operations using H.264}, author = {Fabrizio Nenci and Luciano Spinello and Cyrill Stachniss}, booktitle = iros, year = {2014}, }
- S. Oßwald, H. Kretzschmar, W. Burgard, and C. Stachniss, “Learning to Give Route Directions from Human Demonstrations,” in Proc. of the IEEE Intl. Conf. on Robotics & Automation (ICRA), Hong Kong, China, 2014.
[BibTeX] [PDF]@InProceedings{osswald2014icra, title = {Learning to Give Route Directions from Human Demonstrations}, author = {S. O{\ss}wald and H. Kretzschmar and W. Burgard and C. Stachniss}, booktitle = icra, year = {2014}, address = {Hong Kong, China}, }
- C. Stachniss and W. Burgard, “Particle Filters for Robot Navigation,” Foundations and Trends in Robotics, vol. 3, iss. 4, pp. 211-282, 2014. doi:10.1561/2300000013
[BibTeX] [PDF]
[none]
@Article{stachniss2014, title = {Particle Filters for Robot Navigation}, author = {C. Stachniss and W. Burgard}, journal = fntr, year = {2014}, month = {2012, published 2014}, number = {4}, pages = {211-282}, volume = {3}, abstract = {[none]}, doi = {10.1561/2300000013}, timestamp = {2014.04.24}, url = {https://www.nowpublishers.com/articles/foundations-and-trends-in-robotics/ROB-013}, }
- O. Vysotska, B. Frank, I. Ulbert, O. Paul, P. Ruther, C. Stachniss, and W. Burgard, “Automatic Channel Selection and Neural Signal Estimation across Channels of Neural Probes,” in Proc. of the IEEE/RSJ Intl. Conf. on Intelligent Robots and Systems (IROS), Chicago, USA, 2014.
[BibTeX] [PDF]@InProceedings{vysotska2014iros, title = {Automatic Channel Selection and Neural Signal Estimation across Channels of Neural Probes}, author = {O. Vysotska and B. Frank and I. Ulbert and O. Paul and P. Ruther and C. Stachniss and W. Burgard}, booktitle = iros, year = {2014}, address = {Chicago, USA}, }
- V. A. Ziparo, G. Castelli, L. Van Gool, G. Grisetti, B. Leibe, M. Proesmans, and C. Stachniss, “The ROVINA Project. Robots for Exploration, Digital Preservation and Visualization of Archeological sites,” in Proc. of the 18th ICOMOS General Assembly and Scientific Symposium “Heritage and Landscape as Human Values”, 2014.
[BibTeX]
[none]
@InProceedings{ziparo14icomosga, title = {The ROVINA Project. Robots for Exploration, Digital Preservation and Visualization of Archeological sites}, author = {Ziparo, V.A. and Castelli, G. and Van Gool, L. and Grisetti, G. and Leibe, B. and Proesmans, M. and Stachniss, C.}, booktitle = {Proc. of the 18th ICOMOS General Assembly and Scientific Symposium ``Heritage and Landscape as Human Values"}, year = {2014}, abstract = {[none]}, timestamp = {2015.03.02}, }
2013
- N. Abdo, H. Kretzschmar, L. Spinello, and C. Stachniss, “Learning Manipulation Actions from a Few Demonstrations,” in Proc. of the IEEE Intl. Conf. on Robotics & Automation (ICRA), Karlsruhe, Germany, 2013.
[BibTeX] [PDF]
[none]
@InProceedings{abdo2013, title = {Learning Manipulation Actions from a Few Demonstrations}, author = {N. Abdo and H. Kretzschmar and L. Spinello and C. Stachniss}, booktitle = icra, year = {2013}, address = {Karlsruhe, Germany}, abstract = {[none]}, timestamp = {2014.04.24}, url = {https://www.ipb.uni-bonn.de/wp-content/papercite-data/pdf/abdo13icra.pdf}, }
- P. Agarwal, G. D. Tipaldi, L. Spinello, C. Stachniss, and W. Burgard, “Dynamic Covariance Scaling for Robust Robotic Mapping,” in ICRA Workshop on robust and Multimodal Inference in Factor Graphs, Karlsruhe, Germany, 2013.
[BibTeX] [PDF]
[none]
@InProceedings{agarwal2013, title = {Dynamic Covariance Scaling for Robust Robotic Mapping}, author = {P. Agarwal and G.D. Tipaldi and L. Spinello and C. Stachniss and W. Burgard}, booktitle = {ICRA Workshop on robust and Multimodal Inference in Factor Graphs}, year = {2013}, address = {Karlsruhe, Germany}, abstract = {[none]}, timestamp = {2014.04.24}, url = {https://www.ipb.uni-bonn.de/wp-content/papercite-data/pdf/agarwal13icraws.pdf}, }
- P. Agarwal, G. D. Tipaldi, L. Spinello, C. Stachniss, and W. Burgard, “Robust Map Optimization using Dynamic Covariance Scaling,” in Proc. of the IEEE Intl. Conf. on Robotics & Automation (ICRA), Karlsruhe, Germany, 2013.
[BibTeX] [PDF]
[none]
@InProceedings{agarwal2013a, title = {Robust Map Optimization using Dynamic Covariance Scaling}, author = {P. Agarwal and G.D. Tipaldi and L. Spinello and C. Stachniss and W. Burgard}, booktitle = icra, year = {2013}, address = {Karlsruhe, Germany}, abstract = {[none]}, timestamp = {2014.04.24}, url = {https://www.ipb.uni-bonn.de/wp-content/papercite-data/pdf/agarwal13icra.pdf}, }
- I. Bogoslavskyi, O. Vysotska, J. Serafin, G. Grisetti, and C. Stachniss, “Efficient Traversability Analysis for Mobile Robots using the Kinect Sensor,” in Proc. of the European Conf. on Mobile Robots (ECMR), Barcelona, Spain, 2013.
[BibTeX] [PDF]
[none]
@InProceedings{bogoslavskyi2013, title = {Efficient Traversability Analysis for Mobile Robots using the Kinect Sensor}, author = {I. Bogoslavskyi and O. Vysotska and J. Serafin and G. Grisetti and C. Stachniss}, booktitle = ecmr, year = {2013}, address = {Barcelona, Spain}, abstract = {[none]}, timestamp = {2014.04.24}, url = {https://www.ipb.uni-bonn.de/wp-content/papercite-data/pdf/bogoslavskyi13ecmr.pdf}, }
- W. Burgard and C. Stachniss, “Gestatten, Obelix!,” Forschung – Das Magazin der Deutschen Forschungsgemeinschaft, vol. 1, 2013.
[BibTeX] [PDF]
[none]
@Article{burgard2013, title = {Gestatten, Obelix!}, author = {W. Burgard and C. Stachniss}, journal = {Forschung -- Das Magazin der Deutschen Forschungsgemeinschaft}, year = {2013}, note = {In German, invited}, volume = {1}, abstract = {[none]}, timestamp = {2014.04.24}, url = {https://www.ipb.uni-bonn.de/wp-content/papercite-data/pdf/forschung_2013_01-pg4-9.pdf}, }
- A. Hornung, K. M. Wurm, M. Bennewitz, C. Stachniss, and W. Burgard, “OctoMap: An Efficient Probabilistic 3D Mapping Framework Based on Octrees,” Autonomous Robots, vol. 34, pp. 189-206, 2013.
[BibTeX] [PDF]
[none]
@Article{hornung2013, title = {{OctoMap}: An Efficient Probabilistic 3D Mapping Framework Based on Octrees}, author = {A. Hornung and K.M. Wurm and M. Bennewitz and C. Stachniss and W. Burgard}, journal = auro, year = {2013}, pages = {189-206}, volume = {34}, abstract = {[none]}, issue = {3}, timestamp = {2014.04.24}, url = {https://www.ipb.uni-bonn.de/wp-content/papercite-data/pdf/hornung13auro.pdf}, }
- R. Kümmerle, M. Ruhnke, B. Steder, C. Stachniss, and W. Burgard, “A Navigation System for Robots Operating in Crowded Urban Environments,” in Proc. of the IEEE Intl. Conf. on Robotics & Automation (ICRA), Karlsruhe, Germany, 2013.
[BibTeX] [PDF]
[none]
@InProceedings{kummerle2013, title = {A Navigation System for Robots Operating in Crowded Urban Environments}, author = {R. K\"ummerle and M. Ruhnke and B. Steder and C. Stachniss and W. Burgard}, booktitle = icra, year = {2013}, address = {Karlsruhe, Germany}, abstract = {[none]}, timestamp = {2014.04.24}, url = {https://www.ipb.uni-bonn.de/wp-content/papercite-data/pdf/kuemmerle13icra.pdf}, }
- D. Maier, C. Stachniss, and M. Bennewitz, “Vision-Based Humanoid Navigation Using Self-Supervised Obstacle Detection,” The Intl. Journal of Humanoid Robotics (IJHR), vol. 10, 2013.
[BibTeX] [PDF]
[none]
@Article{maier2013, title = {Vision-Based Humanoid Navigation Using Self-Supervised Obstacle Detection}, author = {D. Maier and C. Stachniss and M. Bennewitz}, journal = ijhr, year = {2013}, volume = {10}, abstract = {[none]}, issue = {2}, timestamp = {2014.04.24}, url = {https://www.ipb.uni-bonn.de/wp-content/papercite-data/pdf/maier13ijhr.pdf}, }
- K. M. Wurm, C. Dornhege, B. Nebel, W. Burgard, and C. Stachniss, “Coordinating Heterogeneous Teams of Robots using Temporal Symbolic Planning,” Autonomous Robots, vol. 34, 2013.
[BibTeX] [PDF]
[none]
@Article{wurm2013, title = {Coordinating Heterogeneous Teams of Robots using Temporal Symbolic Planning}, author = {K.M. Wurm and C. Dornhege and B. Nebel and W. Burgard and C. Stachniss}, journal = auro, year = {2013}, volume = {34}, abstract = {[none]}, issue = {4}, timestamp = {2014.04.24}, url = {https://www.ipb.uni-bonn.de/wp-content/papercite-data/pdf/wurm13auro.pdf}, }
- K. M. Wurm, H. Kretzschmar, R. Kümmerle, C. Stachniss, and W. Burgard, “Identifying Vegetation from Laser Data in Structured Outdoor Environments,” Robotics and Autonomous Systems, 2013.
[BibTeX] [PDF]
[none]
@Article{wurm2013a, title = {Identifying Vegetation from Laser Data in Structured Outdoor Environments}, author = {K.M. Wurm and H. Kretzschmar and R. K{\"u}mmerle and C. Stachniss and W. Burgard}, journal = jras, year = {2013}, note = {In press}, abstract = {[none]}, timestamp = {2014.04.24}, url = {https://www.ipb.uni-bonn.de/wp-content/papercite-data/pdf/wurm13ras.pdf}, }
2012
- N. Abdo, H. Kretzschmar, and C. Stachniss, “From Low-Level Trajectory Demonstrations to Symbolic Actions for Planning,” in Proc. of the ICAPS Workshop on Combining Task and Motion Planning for Real-World Applications (TAMPRA), 2012.
[BibTeX] [PDF]
[none]
@InProceedings{abdo2012, title = {From Low-Level Trajectory Demonstrations to Symbolic Actions for Planning}, author = {N. Abdo and H. Kretzschmar and C. Stachniss}, booktitle = {Proc. of the ICAPS Workshop on Combining Task and Motion Planning for Real-World Applications (TAMPRA)}, year = {2012}, abstract = {[none]}, timestamp = {2014.04.24}, url = {https://www.ipb.uni-bonn.de/wp-content/papercite-data/pdf/abdo12tampra.pdf}, }
- G. Grisetti, L. Iocchi, B. Leibe, V. A. Ziparo, and C. Stachniss, “Digitization of Inaccessible Archeological Sites with Autonomous Mobile Robots,” in Conf. on Robotics Innovation for Cultural Heritage, 2012.
[BibTeX]
[none]
@InProceedings{grisetti2012, title = {Digitization of Inaccessible Archeological Sites with Autonomous Mobile Robots}, author = {G. Grisetti and L. Iocchi and B. Leibe and V.A. Ziparo and C. Stachniss}, booktitle = {Conf. on Robotics Innovation for Cultural Heritage}, year = {2012}, abstract = {[none]}, notes = {Extended abstract}, timestamp = {2014.04.24}, }
- D. Joho, G. D. Tipaldi, N. Engelhard, C. Stachniss, and W. Burgard, “Nonparametric Bayesian Models for Unsupervised Scene Analysis and Reconstruction,” in Proc. of Robotics: Science and Systems (RSS), 2012.
[BibTeX] [PDF]
[none]
@InProceedings{joho2012, title = {Nonparametric {B}ayesian Models for Unsupervised Scene Analysis and Reconstruction}, author = {D. Joho and G.D. Tipaldi and N. Engelhard and C. Stachniss and W. Burgard}, booktitle = rss, year = {2012}, abstract = {[none]}, timestamp = {2014.04.24}, url = {https://www.ipb.uni-bonn.de/wp-content/papercite-data/pdf/joho12rss.pdf}, }
- H. Kretzschmar and C. Stachniss, “Information-Theoretic Pose Graph Compression for Laser-based SLAM,” The Intl. Journal of Robotics Research, vol. 31, p. 1219–1230, 2012.
[BibTeX] [PDF]
[none]
@Article{kretzschmar2012, title = {Information-Theoretic Pose Graph Compression for Laser-based {SLAM}}, author = {H. Kretzschmar and C. Stachniss}, journal = ijrr, year = {2012}, pages = {1219--1230}, volume = {31}, abstract = {[none]}, issue = {11}, timestamp = {2014.04.24}, url = {https://www.ipb.uni-bonn.de/wp-content/papercite-data/pdf/kretzschmar12ijrr.pdf}, }
- J. Roewekaemper, C. Sprunk, G. D. Tipaldi, C. Stachniss, P. Pfaff, and W. Burgard, “On the Position Accuracy of Mobile Robot Localization based on Particle Filters combined with Scan Matching,” in Proc. of the IEEE/RSJ Intl. Conf. on Intelligent Robots and Systems (IROS), 2012.
[BibTeX] [PDF]
[none]
@InProceedings{roewekaemper2012, title = {On the Position Accuracy of Mobile Robot Localization based on Particle Filters combined with Scan Matching}, author = {J. Roewekaemper and C. Sprunk and G.D. Tipaldi and C. Stachniss and P. Pfaff and W. Burgard}, booktitle = iros, year = {2012}, abstract = {[none]}, timestamp = {2014.04.24}, url = {https://ais.informatik.uni-freiburg.de/publications/papers/roewekaemper12iros.pdf}, }
- L. Spinello, C. Stachniss, and W. Burgard, “Scene in the Loop: Towards Adaptation-by-Tracking in RGB-D Data,” in Proc. of the RSS Workshop RGB-D: Advanced Reasoning with Depth Cameras, 2012.
[BibTeX] [PDF]
[none]
@InProceedings{spinello2012, title = {Scene in the Loop: Towards Adaptation-by-Tracking in RGB-D Data}, author = {L. Spinello and C. Stachniss and W. Burgard}, booktitle = {Proc. of the RSS Workshop RGB-D: Advanced Reasoning with Depth Cameras}, year = {2012}, abstract = {[none]}, timestamp = {2014.04.24}, url = {https://www.ipb.uni-bonn.de/wp-content/papercite-data/pdf/spinello12rssws.pdf}, }
2011
- S. Asadi, M. Reggente, C. Stachniss, C. Plagemann, and A. J. Lilienthal, “Intelligent Systems for Machine Olfaction: Tools and Methodologies,” , E. L. Hines and M. S. Leeson, Eds., IGI Global, 2011, pp. 153-179.
[BibTeX]
[none]
@InBook{asadi2011, title = {Intelligent Systems for Machine Olfaction: Tools and Methodologies}, author = {S. Asadi and M. Reggente and C. Stachniss and C. Plagemann and A.J. Lilienthal}, chapter = {Statistical Gas Distribution Modelling using Kernel Methods}, editor = {E.L. Hines and M.S. Leeson}, pages = {153-179}, publisher = {{IGI} {G}lobal}, year = {2011}, abstract = {[none]}, timestamp = {2014.04.24}, }
- J. Becker, C. Bersch, D. Pangercic, B. Pitzer, T. Rühr, B. Sankaran, J. Sturm, C. Stachniss, M. Beetz, and W. Burgard, “Mobile Manipulation of Kitchen Containers,” in Proc. of the IROS’11 Workshop on Results, Challenges and Lessons Learned in Advancing Robots with a Common Platform, San Francisco, CA, USA, 2011.
[BibTeX] [PDF]
[none]
@InProceedings{becker2011, title = {Mobile Manipulation of Kitchen Containers}, author = {J. Becker and C. Bersch and D. Pangercic and B. Pitzer and T. R\"uhr and B. Sankaran and J. Sturm and C. Stachniss and M. Beetz and W. Burgard}, booktitle = {Proc. of the IROS'11 Workshop on Results, Challenges and Lessons Learned in Advancing Robots with a Common Platform}, year = {2011}, address = {San Francisco, CA, USA}, abstract = {[none]}, timestamp = {2014.04.24}, url = {https://www.ipb.uni-bonn.de/wp-content/papercite-data/pdf/becker11irosws.pdf}, }
- M. Bennewitz, D. Maier, A. Hornung, and C. Stachniss, “Integrated Perception and Navigation in Complex Indoor Environments,” in Proc. of the IEEE-RAS Int. Conf. on Humanoid Robots (HUMANOIDS), 2011.
[BibTeX]
[none]
@InProceedings{bennewitz2011, title = {Integrated Perception and Navigation in Complex Indoor Environments}, author = {M. Bennewitz and D. Maier and A. Hornung and C. Stachniss}, booktitle = {Proc. of the IEEE-RAS Int. Conf. on Humanoid Robots (HUMANOIDS)}, year = {2011}, note = {Invited presentation at the workshop on Humanoid service robot navigation in crowded and dynamic environments}, abstract = {[none]}, timestamp = {2014.04.24}, }
- B. Frank, C. Stachniss, N. Abdo, and W. Burgard, “Using Gaussian Process Regression for Efficient Motion Planning in Environments with Deformable Objects,” in Proc. of the AAAI-11 Workshop on Automated Action Planning for Autonomous Mobile Robots (PAMR), San Francisco, CA, USA, 2011.
[BibTeX] [PDF]
[none]
@InProceedings{frank2011, title = {Using Gaussian Process Regression for Efficient Motion Planning in Environments with Deformable Objects}, author = {B. Frank and C. Stachniss and N. Abdo and W. Burgard}, booktitle = {Proc. of the AAAI-11 Workshop on Automated Action Planning for Autonomous Mobile Robots (PAMR)}, year = {2011}, address = {San Francisco, CA, USA}, abstract = {[none]}, timestamp = {2014.04.24}, url = {https://www.ipb.uni-bonn.de/wp-content/papercite-data/pdf/frank11pamr.pdf}, }
- B. Frank, C. Stachniss, N. Abdo, and W. Burgard, “Efficient Motion Planning for Manipulation Robots in Environments with Deformable Objects,” in Proc. of the IEEE/RSJ Intl. Conf. on Intelligent Robots and Systems (IROS), San Francisco, CA, USA, 2011.
[BibTeX] [PDF]
[none]
@InProceedings{frank2011a, title = {Efficient Motion Planning for Manipulation Robots in Environments with Deformable Objects}, author = {B. Frank and C. Stachniss and N. Abdo and W. Burgard}, booktitle = iros, year = {2011}, address = {San Francisco, CA, USA}, abstract = {[none]}, timestamp = {2014.04.24}, url = {https://www.ipb.uni-bonn.de/wp-content/papercite-data/pdf/frank11iros.pdf}, }
- R. Kümmerle, G. Grisetti, C. Stachniss, and W. Burgard, “Simultaneous Parameter Calibration, Localization, and Mapping for Robust Service Robotics,” in Proc. of the IEEE Workshop on Advanced Robotics and its Social Impacts, Half-Moon Bay, CA, USA, 2011.
[BibTeX] [PDF]
[none]
@InProceedings{kummerle2011, title = {Simultaneous Parameter Calibration, Localization, and Mapping for Robust Service Robotics}, author = {R. K\"ummerle and G. Grisetti and C. Stachniss and W. Burgard}, booktitle = {Proc. of the IEEE Workshop on Advanced Robotics and its Social Impacts}, year = {2011}, address = {Half-Moon Bay, CA, USA}, abstract = {[none]}, timestamp = {2014.04.24}, url = {https://www.ipb.uni-bonn.de/wp-content/papercite-data/pdf/kuemmerle11arso.pdf}, }
- H. Kretzschmar and C. Stachniss, “Pose Graph Compression for Laser-based SLAM,” in Proc. of the Intl. Symposium of Robotics Research (ISRR), Flagstaff, AZ, USA, 2011.
[BibTeX] [PDF]
[none]
@InProceedings{kretzschmar2011a, title = {Pose Graph Compression for Laser-based {SLAM}}, author = {H. Kretzschmar and C. Stachniss}, booktitle = isrr, year = {2011}, address = {Flagstaff, AZ, USA}, note = {Invited presentation}, abstract = {[none]}, timestamp = {2014.04.24}, url = {https://www.ipb.uni-bonn.de/wp-content/papercite-data/pdf/stachniss11isrr.pdf}, }
- H. Kretzschmar, C. Stachniss, and G. Grisetti, “Efficient Information-Theoretic Graph Pruning for Graph-Based SLAM with Laser Range Finders,” in Proc. of the IEEE/RSJ Intl. Conf. on Intelligent Robots and Systems (IROS), San Francisco, CA, USA, 2011.
[BibTeX] [PDF]
[none]
@InProceedings{kretzschmar2011, title = {Efficient Information-Theoretic Graph Pruning for Graph-Based {SLAM} with Laser Range Finders}, author = {H. Kretzschmar and C. Stachniss and G. Grisetti}, booktitle = iros, year = {2011}, address = {San Francisco, CA, USA}, abstract = {[none]}, timestamp = {2014.04.24}, url = {https://www.ipb.uni-bonn.de/wp-content/papercite-data/pdf/kretzschmar11iros.pdf}, }
- D. Maier, M. Bennewitz, and C. Stachniss, “Self-supervised Obstacle Detection for Humanoid Navigation Using Monocular Vision and Sparse Laser Data,” in Proc. of the IEEE Intl. Conf. on Robotics & Automation (ICRA), Shanghai, China, 2011.
[BibTeX] [PDF]
[none]
@InProceedings{maier2011, title = {Self-supervised Obstacle Detection for Humanoid Navigation Using Monocular Vision and Sparse Laser Data}, author = {D. Maier and M. Bennewitz and C. Stachniss}, booktitle = icra, year = {2011}, address = {Shanghai, China}, abstract = {[none]}, timestamp = {2014.04.24}, url = {https://www.ipb.uni-bonn.de/wp-content/papercite-data/pdf/maier11icra.pdf}, }
- J. Sturm, C. Stachniss, and W. Burgard, “A Probabilistic Framework for Learning Kinematic Models of Articulated Objects,” Journal on Artificial Intelligence Research, vol. 41, p. 477–526, 2011.
[BibTeX] [PDF]
[none]
@Article{sturm2011, title = {A Probabilistic Framework for Learning Kinematic Models of Articulated Objects}, author = {J. Sturm and C. Stachniss and W. Burgard}, journal = jair, year = {2011}, pages = {477--526}, volume = {41}, abstract = {[none]}, timestamp = {2014.04.24}, url = {https://www.ipb.uni-bonn.de/wp-content/papercite-data/pdf/sturm11jair.pdf}, }
- K. M. Wurm, D. Hennes, D. Holz, R. B. Rusu, C. Stachniss, K. Konolige, and W. Burgard, “Hierarchies of Octrees for Efficient 3D Mapping,” in Proc. of the IEEE/RSJ Intl. Conf. on Intelligent Robots and Systems (IROS), San Francisco, CA, USA, 2011.
[BibTeX] [PDF]
[none]
@InProceedings{wurm2011, title = {Hierarchies of Octrees for Efficient 3D Mapping}, author = {K.M. Wurm and D. Hennes and D. Holz and R.B. Rusu and C. Stachniss and K. Konolige and W. Burgard}, booktitle = iros, year = {2011}, address = {San Francisco, CA, USA}, abstract = {[none]}, timestamp = {2014.04.24}, url = {https://www.ipb.uni-bonn.de/wp-content/papercite-data/pdf/wurm11iros.pdf}, }
- J. Ziegler, H. Kretzschmar, C. Stachniss, G. Grisetti, and W. Burgard, “Accurate Human Motion Capture in Large Areas by Combining IMU- and Laser-based People Tracking,” in Proc. of the IEEE/RSJ Intl. Conf. on Intelligent Robots and Systems (IROS), San Francisco, CA, USA, 2011.
[BibTeX] [PDF]
[none]
@InProceedings{ziegler2011, title = {Accurate Human Motion Capture in Large Areas by Combining IMU- and Laser-based People Tracking}, author = {J. Ziegler and H. Kretzschmar and C. Stachniss and G. Grisetti and W. Burgard}, booktitle = iros, year = {2011}, address = {San Francisco, CA, USA}, abstract = {[none]}, timestamp = {2014.04.24}, url = {https://www.ipb.uni-bonn.de/wp-content/papercite-data/pdf/ziegler11iros.pdf}, }
2010
- W. Burgard, K. M. Wurm, M. Bennewitz, C. Stachniss, A. Hornung, R. B. Rusu, and K. Konolige, “Modeling the World Around Us: An Efficient 3D Representation for Personal Robotics,” in Workshop on Defining and Solving Realistic Perception Problems in Personal Robotics at the IEEE/RSJ Int.Conf.on Intelligent Robots and Systems, Taipei, Taiwan, 2010.
[BibTeX]
[none]
@InProceedings{burgard2010, title = {Modeling the World Around Us: An Efficient 3D Representation for Personal Robotics}, author = {Burgard, W. and Wurm, K.M. and Bennewitz, M. and Stachniss, C. and Hornung, A. and Rusu, R.B. and Konolige, K.}, booktitle = {Workshop on Defining and Solving Realistic Perception Problems in Personal Robotics at the IEEE/RSJ Int.Conf.on Intelligent Robots and Systems}, year = {2010}, address = {Taipei, Taiwan}, abstract = {[none]}, timestamp = {2014.04.24}, }
- B. Frank, R. Schmedding, C. Stachniss, M. Teschner, and W. Burgard, “Learning Deformable Object Models for Mobile Robot Path Planning using Depth Cameras and a Manipulation Robot,” in Proc. of the Workshop RGB-D: Advanced Reasoning with Depth Cameras at Robotics: Science and Systems (RSS), Zaragoza, Spain, 2010.
[BibTeX] [PDF]
[none]
@InProceedings{frank2010, title = {Learning Deformable Object Models for Mobile Robot Path Planning using Depth Cameras and a Manipulation Robot}, author = {B. Frank and R. Schmedding and C. Stachniss and M. Teschner and W. Burgard}, booktitle = {Proc. of the Workshop RGB-D: Advanced Reasoning with Depth Cameras at Robotics: Science and Systems (RSS)}, year = {2010}, address = {Zaragoza, Spain}, abstract = {[none]}, timestamp = {2014.04.24}, url = {https://www.ipb.uni-bonn.de/wp-content/papercite-data/pdf/frank10rssws.pdf}, }
- B. Frank, R. Schmedding, C. Stachniss, M. Teschner, and W. Burgard, “Learning the Elasticity Parameters of Deformable Objects with a Manipulation Robot,” in Proc. of the IEEE/RSJ Intl. Conf. on Intelligent Robots and Systems (IROS), Taipei, Taiwan, 2010.
[BibTeX] [PDF]
[none]
@InProceedings{frank2010a, title = {Learning the Elasticity Parameters of Deformable Objects with a Manipulation Robot}, author = {B. Frank and R. Schmedding and C. Stachniss and M. Teschner and W. Burgard}, booktitle = iros, year = {2010}, address = {Taipei, Taiwan}, abstract = {[none]}, timestamp = {2014.04.24}, url = {https://www.ipb.uni-bonn.de/wp-content/papercite-data/pdf/frank10iros.pdf}, }
- G. Grisetti, R. Kümmerle, C. Stachniss, and W. Burgard, “A Tutorial on Graph-based SLAM,” IEEE Transactions on Intelligent Transportation Systems Magazine, vol. 2, p. 31–43, 2010.
[BibTeX] [PDF]
[none]
@Article{grisetti2010a, title = {A Tutorial on Graph-based {SLAM}}, author = {G. Grisetti and R. K{\"u}mmerle and C. Stachniss and W. Burgard}, journal = {IEEE Transactions on Intelligent Transportation Systems Magazine}, year = {2010}, pages = {31--43}, volume = {2}, abstract = {[none]}, issue = {4}, timestamp = {2014.04.24}, url = {https://www.ipb.uni-bonn.de/wp-content/papercite-data/pdf/grisetti10titsmag.pdf}, }
- G. Grisetti, R. Kümmerle, C. Stachniss, U. Frese, and C. Hertzberg, “Hierarchical Optimization on Manifolds for Online 2D and 3D Mapping,” in Proc. of the IEEE Intl. Conf. on Robotics & Automation (ICRA), Anchorage, Alaska, 2010.
[BibTeX] [PDF]
[none]
@InProceedings{grisetti2010, title = {Hierarchical Optimization on Manifolds for Online 2D and 3D Mapping}, author = {G. Grisetti and R. K{\"u}mmerle and C. Stachniss and U. Frese and C. Hertzberg}, booktitle = icra, year = {2010}, address = {Anchorage, Alaska}, abstract = {[none]}, timestamp = {2014.04.24}, url = {https://www.ipb.uni-bonn.de/wp-content/papercite-data/pdf/grisetti10icra.pdf}, }
- A. Hornung, M.Bennewitz, C. Stachniss, H. Strasdat, S. Oßwald, and W. Burgard, “Learning Adaptive Navigation Strategies for Resource-Constrained Systems,” in Proc. of the Int. Workshop on Evolutionary and Reinforcement Learning for Autonomous Robot Systems, Lisbon, Portugal, 2010.
[BibTeX] [PDF]
[none]
@InProceedings{hornung2010, title = {Learning Adaptive Navigation Strategies for Resource-Constrained Systems}, author = {A. Hornung and M.Bennewitz and C. Stachniss and H. Strasdat and S. O{\ss}wald and W. Burgard}, booktitle = {Proc. of the Int. Workshop on Evolutionary and Reinforcement Learning for Autonomous Robot Systems}, year = {2010}, address = {Lisbon, Portugal}, abstract = {[none]}, timestamp = {2014.04.24}, url = {https://www.ipb.uni-bonn.de/wp-content/papercite-data/pdf/hornung10erlars.pdf}, }
- M. Karg, K. M. Wurm, C. Stachniss, K. Dietmayer, and W. Burgard, “Consistent Mapping of Multistory Buildings by Introducing Global Constraints to Graph-based SLAM,” in Proc. of the IEEE Intl. Conf. on Robotics & Automation (ICRA), Anchorage, Alaska, 2010.
[BibTeX] [PDF]
[none]
@InProceedings{karg2010, title = {Consistent Mapping of Multistory Buildings by Introducing Global Constraints to Graph-based {SLAM}}, author = {M. Karg and K.M. Wurm and C. Stachniss and K. Dietmayer and W. Burgard}, booktitle = icra, year = {2010}, address = {Anchorage, Alaska}, abstract = {[none]}, timestamp = {2014.04.24}, url = {https://www.ipb.uni-bonn.de/wp-content/papercite-data/pdf/karg10icra.pdf}, }
- H. Kretzschmar, G. Grisetti, and C. Stachniss, “Lifelong Map Learning for Graph-based SLAM in Static Environments,” KI – Künstliche Intelligenz, vol. 24, p. 199–206, 2010.
[BibTeX]
[none]
@Article{kretzschmar2010, title = {Lifelong Map Learning for Graph-based {SLAM} in Static Environments}, author = {H. Kretzschmar and G. Grisetti and C. Stachniss}, journal = {{KI} -- {K}\"unstliche {I}ntelligenz}, year = {2010}, pages = {199--206}, volume = {24}, abstract = {[none]}, issue = {3}, timestamp = {2014.04.24}, }
- J. Müller, C. Stachniss, K. O. Arras, and W. Burgard, “Socially Inspired Motion Planning for Mobile Robots in Populated Environments,” in Cognitive Systems, Springer, 2010.
[BibTeX]
[none]
@InCollection{muller2010, title = {Socially Inspired Motion Planning for Mobile Robots in Populated Environments}, author = {M\"{u}ller, J. and Stachniss, C. and Arras, K.O. and Burgard, W.}, booktitle = {Cognitive Systems}, publisher = springer, year = {2010}, note = {In press}, series = {Cognitive Systems Monographs}, abstract = {[none]}, timestamp = {2014.04.24}, }
- C. Plagemann, C. Stachniss, J. Hess, F. Endres, and N. Franklin, “A Nonparametric Learning Approach to Range Sensing from Omnidirectional Vision,” Robotics and Autonomous Systems, vol. 58, p. 762–772, 2010.
[BibTeX]
[none]
@Article{plagemann2010, title = {A Nonparametric Learning Approach to Range Sensing from Omnidirectional Vision}, author = {C. Plagemann and C. Stachniss and J. Hess and F. Endres and N. Franklin}, journal = jras, year = {2010}, pages = {762--772}, volume = {58}, abstract = {[none]}, issue = {6}, timestamp = {2014.04.24}, }
- J. Sturm, A. Jain, C. Stachniss, C. C. Kemp, and W. Burgard, “Robustly Operating Articulated Objects based on Experience,” in Proc. of the IEEE/RSJ Intl. Conf. on Intelligent Robots and Systems (IROS), Taipei, Taiwan, 2010.
[BibTeX] [PDF]
[none]
@InProceedings{sturm2010b, title = {Robustly Operating Articulated Objects based on Experience}, author = {J. Sturm and A. Jain and C. Stachniss and C.C. Kemp and W. Burgard}, booktitle = iros, year = {2010}, address = {Taipei, Taiwan}, abstract = {[none]}, timestamp = {2014.04.24}, url = {https://www.ipb.uni-bonn.de/wp-content/papercite-data/pdf/sturm10iros.pdf}, }
- J. Sturm, K. Konolige, C. Stachniss, and W. Burgard, “Vision-based Detection for Learning Articulation Models of Cabinet Doors and Drawers in Household Environments,” in Proc. of the IEEE Intl. Conf. on Robotics & Automation (ICRA), Anchorage, Alaska, 2010.
[BibTeX] [PDF]
[none]
@InProceedings{sturm2010, title = {Vision-based Detection for Learning Articulation Models of Cabinet Doors and Drawers in Household Environments}, author = {J. Sturm and K. Konolige and C. Stachniss and W. Burgard}, booktitle = icra, year = {2010}, address = {Anchorage, Alaska}, abstract = {[none]}, timestamp = {2014.04.24}, url = {https://www.ipb.uni-bonn.de/wp-content/papercite-data/pdf/sturm10icra.pdf}, }
- J. Sturm, K. Konolige, C. Stachniss, and W. Burgard, “3D Pose Estimation, Tracking and Model Learning of Articulated Objects from Dense Depth Video using Projected Texture Stereo,” in Proc. of the Workshop RGB-D: Advanced Reasoning with Depth Cameras at Robotics: Science and Systems (RSS), Zaragoza, Spain, 2010.
[BibTeX] [PDF]
[none]
@InProceedings{sturm2010a, title = {3D Pose Estimation, Tracking and Model Learning of Articulated Objects from Dense Depth Video using Projected Texture Stereo}, author = {J. Sturm and K. Konolige and C. Stachniss and W. Burgard}, booktitle = {Proc. of the Workshop RGB-D: Advanced Reasoning with Depth Cameras at Robotics: Science and Systems (RSS)}, year = {2010}, address = {Zaragoza, Spain}, abstract = {[none]}, timestamp = {2014.04.24}, url = {https://www.ipb.uni-bonn.de/wp-content/papercite-data/pdf/sturm10rssws.pdf}, }
- K. M. Wurm, C. Dornhege, P. Eyerich, C. Stachniss, B. Nebel, and W. Burgard, “Coordinated Exploration with Marsupial Teams of Robots using Temporal Symbolic Planning,” in Proc. of the IEEE/RSJ Intl. Conf. on Intelligent Robots and Systems (IROS), Taipei, Taiwan, 2010.
[BibTeX] [PDF]
[none]
@InProceedings{wurm2010a, title = {Coordinated Exploration with Marsupial Teams of Robots using Temporal Symbolic Planning}, author = {K.M. Wurm and C. Dornhege and P. Eyerich and C. Stachniss and B. Nebel and W. Burgard}, booktitle = iros, year = {2010}, address = {Taipei, Taiwan}, abstract = {[none]}, timestamp = {2014.04.24}, url = {https://www.ipb.uni-bonn.de/wp-content/papercite-data/pdf/wurm10iros.pdf}, }
- K. M. Wurm, A. Hornung, M. Bennewitz, C. Stachniss, and W. Burgard, “OctoMap: A Probabilistic, Flexible, and Compact 3D Map Representation for Robotic Systems,” in Proc. of the ICRA 2010 Workshop on Best Practice in 3D Perception and Modeling for Mobile Manipulation, Anchorage, AK, USA, 2010.
[BibTeX] [PDF]
[none]
@InProceedings{wurm2010, title = {{OctoMap}: A Probabilistic, Flexible, and Compact {3D} Map Representation for Robotic Systems}, author = {K.M. Wurm and A. Hornung and M. Bennewitz and C. Stachniss and W. Burgard}, booktitle = {Proc. of the ICRA 2010 Workshop on Best Practice in 3D Perception and Modeling for Mobile Manipulation}, year = {2010}, address = {Anchorage, AK, USA}, abstract = {[none]}, timestamp = {2014.04.24}, url = {https://www.ipb.uni-bonn.de/wp-content/papercite-data/pdf/wurm10icraws.pdf}, }
- K. M. Wurm, C. Stachniss, and G. Grisetti, “Bridging the Gap Between Feature- and Grid-based SLAM,” Robotics and Autonomous Systems, vol. 58, iss. 2, pp. 140-148, 2010. doi:10.1016/j.robot.2009.09.009
[BibTeX] [PDF]
[none]
@Article{wurm2010b, title = {Bridging the Gap Between Feature- and Grid-based SLAM}, author = {Wurm, K.M. and Stachniss, C. and Grisetti, G.}, journal = jras, year = {2010}, number = {2}, pages = {140 - 148}, volume = {58}, abstract = {[none]}, doi = {10.1016/j.robot.2009.09.009}, issn = {0921-8890}, timestamp = {2014.04.24}, url = {https://ais.informatik.uni-freiburg.de/publications/papers/wurm10ras.pdf}, }
2009
- M. Bennewitz, C. Stachniss, S. Behnke, and W. Burgard, “Utilizing Reflection Properties of Surfaces to Improve Mobile Robot Localization,” in Proc. of the IEEE Intl. Conf. on Robotics & Automation (ICRA), Kobe, Japan, 2009.
[BibTeX]
[none]
@InProceedings{bennewitz2009, title = {Utilizing Reflection Properties of Surfaces to Improve Mobile Robot Localization}, author = {M. Bennewitz and Stachniss, C. and Behnke, S. and Burgard, W.}, booktitle = icra, year = {2009}, address = {Kobe, Japan}, abstract = {[none]}, timestamp = {2014.04.24}, }
- W. Burgard, C. Stachniss, G. Grisetti, B. Steder, R. Kümmerle, C. Dornhege, M. Ruhnke, A. Kleiner, and J. D. Tardós, “A Comparison of SLAM Algorithms Based on a Graph of Relations,” in Proc. of the IEEE/RSJ Intl. Conf. on Intelligent Robots and Systems (IROS), 2009.
[BibTeX] [PDF]
[none]
@InProceedings{burgard2009, title = {A Comparison of {SLAM} Algorithms Based on a Graph of Relations}, author = {W. Burgard and C. Stachniss and G. Grisetti and B. Steder and R. K\"ummerle and C. Dornhege and M. Ruhnke and A. Kleiner and J.D. Tard\'os}, booktitle = iros, year = {2009}, abstract = {[none]}, timestamp = {2014.04.24}, url = {https://www.ipb.uni-bonn.de/wp-content/papercite-data/pdf/burgard09iros.pdf}, }
- F. Endres, J. Hess, N. Franklin, C. Plagemann, C. Stachniss, and W. Burgard, “Estimating Range Information from Monocular Vision,” in Workshop Regression in Robotics – Approaches and Applications at Robotics: Science and Systems (RSS), Seattle, WA, USA, 2009.
[BibTeX]
[none]
@InProceedings{endres2009, title = {Estimating Range Information from Monocular Vision}, author = {Endres, F. and Hess, J. and Franklin, N. and Plagemann, C. and Stachniss, C. and Burgard, W.}, booktitle = {Workshop Regression in Robotics - Approaches and Applications at Robotics: Science and Systems (RSS)}, year = {2009}, address = {Seattle, WA, USA}, abstract = {[none]}, timestamp = {2014.04.24}, }
- F. Endres, C. Plagemann, C. Stachniss, and W. Burgard, “Scene Analysis using Latent Dirichlet Allocation,” in Proc. of Robotics: Science and Systems (RSS), Seattle, WA, USA, 2009.
[BibTeX] [PDF]
[none]
@InProceedings{endres2009a, title = {Scene Analysis using Latent Dirichlet Allocation}, author = {F. Endres and C. Plagemann and Stachniss, C. and Burgard, W.}, booktitle = rss, year = {2009}, address = {Seattle, WA, USA}, abstract = {[none]}, timestamp = {2014.04.24}, url = {https://www.ipb.uni-bonn.de/wp-content/papercite-data/pdf/endres09rss-draft.pdf}, }
- C. Eppner, J. Sturm, M. Bennewitz, C. Stachniss, and W. Burgard, “Imitation Learning with Generalized Task Descriptions,” in Proc. of the IEEE Intl. Conf. on Robotics & Automation (ICRA), Kobe, Japan, 2009.
[BibTeX]
[none]
@InProceedings{eppner2009, title = {Imitation Learning with Generalized Task Descriptions}, author = {C. Eppner and J. Sturm and M. Bennewitz and Stachniss, C. and Burgard, W.}, booktitle = icra, year = {2009}, address = {Kobe, Japan}, abstract = {[none]}, timestamp = {2014.04.24}, }
- B. Frank, C. Stachniss, R. Schmedding, W. Burgard, and M. Teschner, “Real-world Robot Navigation amongst Deformable Obstacles,” in Proc. of the IEEE Intl. Conf. on Robotics & Automation (ICRA), Kobe, Japan, 2009.
[BibTeX]
[none]
@InProceedings{frank2009, title = {Real-world Robot Navigation amongst Deformable Obstacles}, author = {B. Frank and C. Stachniss and R. Schmedding and W. Burgard and M. Teschner}, booktitle = icra, year = {2009}, address = {Kobe, Japan}, abstract = {[none]}, timestamp = {2014.04.24}, }
- G. Grisetti, C. Stachniss, and W. Burgard, “Non-linear Constraint Network Optimization for Efficient Map Learning,” IEEE Transactions on Intelligent Transportation Systems, vol. 10, iss. 3, p. 428–439, 2009.
[BibTeX] [PDF]
[none]
@Article{grisetti2009, title = {Non-linear Constraint Network Optimization for Efficient Map Learning}, author = {Grisetti, G. and Stachniss, C. and Burgard, W.}, journal = ieeeits, year = {2009}, number = {3}, pages = {428--439}, volume = {10}, abstract = {[none]}, timestamp = {2014.04.24}, url = {https://www.ipb.uni-bonn.de/wp-content/papercite-data/pdf/grisetti09its.pdf}, }
- R. Kuemmerle, B. Steder, C. Dornhege, M. Ruhnke, G. Grisetti, C. Stachniss, and A. Kleiner, “On measuring the accuracy of SLAM algorithms,” Autonomous Robots, vol. 27, p. 387ff, 2009.
[BibTeX] [PDF]
[none]
@Article{kuemmerle2009, title = {On measuring the accuracy of {SLAM} algorithms}, author = {R. Kuemmerle and B. Steder and C. Dornhege and M. Ruhnke and G. Grisetti and C. Stachniss and A. Kleiner}, journal = auro, year = {2009}, pages = {387ff}, volume = {27}, abstract = {[none]}, issue = {4}, timestamp = {2014.04.24}, url = {https://www.ipb.uni-bonn.de/wp-content/papercite-data/pdf/kuemmerle09auro.pdf}, }
- A. Schneider, S. J. C. Stachniss, M. Reisert, H. Burkhardt, and W. Burgard, “Object Identification with Tactile Sensors Using Bag-of-Features,” in Proc. of the IEEE/RSJ Intl. Conf. on Intelligent Robots and Systems (IROS), 2009.
[BibTeX] [PDF]
[none]
@InProceedings{schneider2009, title = {Object Identification with Tactile Sensors Using Bag-of-Features}, author = {A. Schneider and J. Sturm C. Stachniss and M. Reisert and H. Burkhardt and W. Burgard}, booktitle = iros, year = {2009}, abstract = {[none]}, timestamp = {2014.04.24}, url = {https://www.ipb.uni-bonn.de/wp-content/papercite-data/pdf/wurm09iros.pdf}, }
- C. Stachniss, “Spatial Modeling and Robot Navigation,” Habilitation PhD Thesis, 2009.
[BibTeX] [PDF]
[none]
@PhDThesis{stachniss2009, title = {Spatial Modeling and Robot Navigation}, author = {C. Stachniss}, school = {University of Freiburg, Department of Computer Science}, year = {2009}, type = {Habilitation}, abstract = {[none]}, timestamp = {2014.04.24}, url = {https://www.ipb.uni-bonn.de/wp-content/papercite-data/pdf/stachniss-habil.pdf}, }
- C. Stachniss, Robotic Mapping and Exploration, Springer, 2009, vol. 55.
[BibTeX]
[none]
@Book{stachniss2009a, title = {Robotic Mapping and Exploration}, author = {C. Stachniss}, publisher = {Springer}, year = {2009}, series = springerstaradvanced, volume = {55}, abstract = {[none]}, isbn = {978-3-642-01096-5}, timestamp = {2014.04.24}, }
- C. Stachniss, O. Martinez Mozos, and W. Burgard, “Efficient Exploration of Unknown Indoor Environments using a Team of Mobile Robots,” Annals of Mathematics and Artificial Intelligence, vol. 52, p. 205ff, 2009.
[BibTeX]
[none]
@Article{stachniss2009b, title = {Efficient Exploration of Unknown Indoor Environments using a Team of Mobile Robots}, author = {Stachniss, C. and Martinez Mozos, O. and Burgard, W.}, journal = {Annals of Mathematics and Artificial Intelligence}, year = {2009}, pages = {205ff}, volume = {52}, abstract = {[none]}, issue = {2}, timestamp = {2014.04.24}, }
- C. Stachniss, C. Plagemann, and A. J. Lilienthal, “Gas Distribution Modeling using Sparse Gaussian Process Mixtures,” Autonomous Robots, vol. 26, p. 187ff, 2009.
[BibTeX]
[none]
@Article{stachniss2009c, title = {Gas Distribution Modeling using Sparse Gaussian Process Mixtures}, author = {Stachniss, C. and Plagemann, C. and Lilienthal, A.J.}, journal = auro, year = {2009}, pages = {187ff}, volume = {26}, abstract = {[none]}, issue = {2}, timestamp = {2014.04.24}, }
- H. Strasdat, C. Stachniss, and W. Burgard, “Which Landmark is Useful? Learning Selection Policies for Navigation in Unknown Environments,” in Proc. of the IEEE Intl. Conf. on Robotics & Automation (ICRA), Kobe, Japan, 2009.
[BibTeX]
[none]
@InProceedings{strasdat2009, title = {Which Landmark is Useful? Learning Selection Policies for Navigation in Unknown Environments}, author = {H. Strasdat and Stachniss, C. and Burgard, W.}, booktitle = icra, year = {2009}, address = {Kobe, Japan}, abstract = {[none]}, timestamp = {2014.04.24}, }
- J. Sturm, V. Predeap, C. Stachniss, C. Plagemann, K. Konolige, and W. Burgard, “Learning Kinematic Models for Articulated Objects,” in Proc. of the Intl. Conf. on Artificial Intelligence (IJCAI), Pasadena, CA, USA, 2009.
[BibTeX]
[none]
@InProceedings{sturm2009a, title = {Learning Kinematic Models for Articulated Objects}, author = {J. Sturm and V. Predeap and Stachniss, C. and C. Plagemann and K. Konolige and Burgard, W.}, booktitle = ijcai, year = {2009}, address = {Pasadena, CA, USA}, abstract = {[none]}, timestamp = {2014.04.24}, }
- J. Sturm, C. Stachniss, V. Predeap, C. Plagemann, K. Konolige, and W. Burgard, “Learning Kinematic Models for Articulated Objects,” in Online Proc. of the Learning Workshop (Snowbird), Clearwater, FL, USA, 2009.
[BibTeX]
[none]
@InProceedings{sturm2009, title = {Learning Kinematic Models for Articulated Objects}, author = {J. Sturm and Stachniss, C. and V. Predeap and C. Plagemann and K. Konolige and Burgard, W.}, booktitle = {Online Proc. of the Learning Workshop (Snowbird)}, year = {2009}, address = {Clearwater, FL, USA}, abstract = {[none]}, timestamp = {2014.04.24}, }
- J. Sturm, C. Stachniss, V. Predeap, C. Plagemann, K. Konolige, and W. Burgard, “Towards Understanding Articulated Objects,” in Workshop Integrating Mobility and Manipulation at Robotics: Science and Systems (RSS), Seattle, WA, USA, 2009.
[BibTeX]
[none]
@InProceedings{sturm2009b, title = {Towards Understanding Articulated Objects}, author = {J. Sturm and Stachniss, C. and V. Predeap and C. Plagemann and K. Konolige and Burgard, W.}, booktitle = {Workshop Integrating Mobility and Manipulation at Robotics: Science and Systems (RSS)}, year = {2009}, address = {Seattle, WA, USA}, abstract = {[none]}, timestamp = {2014.04.24}, }
- K. M. Wurm, R. Kuemmerle, C. Stachniss, and W. Burgard, “Improving Robot Navigation in Structured Outdoor Environments by Identifying Vegetation from Laser Data,” in Proc. of the IEEE/RSJ Intl. Conf. on Intelligent Robots and Systems (IROS), 2009.
[BibTeX] [PDF]
[none]
@InProceedings{wurm2009, title = {Improving Robot Navigation in Structured Outdoor Environments by Identifying Vegetation from Laser Data}, author = {K.M. Wurm and R. Kuemmerle and Stachniss, C. and Burgard, W.}, booktitle = iros, year = {2009}, abstract = {[none]}, timestamp = {2014.04.24}, url = {https://www.ipb.uni-bonn.de/wp-content/papercite-data/pdf/wurm09iros.pdf}, }
2008
- B. Frank, M. Becker, C. Stachniss, M. Teschner, and W. Burgard, “Learning Cost Functions for Mobile Robot Navigation in Environments with Deformable Objects,” in Workshop on Path Planning on Cost Maps at the IEEE Int. Conf. on Robotics & Automation (ICRA), Pasadena, CA, USA, 2008.
[BibTeX] [PDF]
[none]
@InProceedings{frank2008, title = {Learning Cost Functions for Mobile Robot Navigation in Environments with Deformable Objects}, author = {Frank, B. and Becker, M. and Stachniss, C. and Teschner, M. and Burgard, W.}, booktitle = icrawsplanning, year = {2008}, address = {Pasadena, CA, USA}, abstract = {[none]}, timestamp = {2014.04.24}, url = {https://www.ipb.uni-bonn.de/wp-content/papercite-data/pdf/frank08icraws.pdf}, }
- B. Frank, M. Becker, C. Stachniss, M. Teschner, and W. Burgard, “Efficient Path Planning for Mobile Robots in Environments with Deformable Objects,” in Proc. of the IEEE Intl. Conf. on Robotics & Automation (ICRA), Pasadena, CA, USA, 2008.
[BibTeX] [PDF]
[none]
@InProceedings{frank2008a, title = {Efficient Path Planning for Mobile Robots in Environments with Deformable Objects}, author = {Frank, B. and Becker, M. and Stachniss, C. and Teschner, M. and Burgard, W.}, booktitle = icra, year = {2008}, address = {Pasadena, CA, USA}, abstract = {[none]}, timestamp = {2014.04.24}, url = {https://www.ipb.uni-bonn.de/wp-content/papercite-data/pdf/frank08icra.pdf}, }
- G. Grisetti, D. Lordi Rizzini, C. Stachniss, E. Olson, and W. Burgard, “Online Constraint Network Optimization for Efficient Maximum Likelihood Map Learning,” in Proc. of the IEEE Intl. Conf. on Robotics & Automation (ICRA), Pasadena, CA, USA, 2008.
[BibTeX] [PDF]
[none]
@InProceedings{grisetti2008, title = {Online Constraint Network Optimization for Efficient Maximum Likelihood Map Learning}, author = {Grisetti, G. and Lordi Rizzini, D. and Stachniss, C. and Olson, E. and Burgard, W.}, booktitle = icra, year = {2008}, address = {Pasadena, CA, USA}, abstract = {[none]}, timestamp = {2014.04.24}, url = {https://www.ipb.uni-bonn.de/wp-content/papercite-data/pdf/grisetti08icra.pdf}, }
- H. Kretzschmar, C. Stachniss, C. Plagemann, and W. Burgard, “Estimating Landmark Locations from Geo-Referenced Photographs,” in Proc. of the IEEE/RSJ Intl. Conf. on Intelligent Robots and Systems (IROS), Nice, France, 2008.
[BibTeX] [PDF]
[none]
@InProceedings{kretzschmar2008, title = {Estimating Landmark Locations from Geo-Referenced Photographs}, author = {Kretzschmar, H. and Stachniss, C. and Plagemann, C. and W. Burgard}, booktitle = iros, year = {2008}, address = {Nice, France}, abstract = {[none]}, timestamp = {2014.04.24}, url = {https://www.ipb.uni-bonn.de/wp-content/papercite-data/pdf/kretzschmar08iros.pdf}, }
- J. Müller, C. Stachniss, K. O. Arras, and W. Burgard, “Socially Inspired Motion Planning for Mobile Robots in Populated Environments,” in Intl. Conf. on Cognitive Systems (CogSys), Baden Baden, Germany, 2008.
[BibTeX]
[none]
@InProceedings{muller2008, title = {Socially Inspired Motion Planning for Mobile Robots in Populated Environments}, author = {M\"uller, J. and Stachniss, C. and Arras, K.O. and Burgard, W.}, booktitle = cogsys, year = {2008}, address = {Baden Baden, Germany}, abstract = {[none]}, timestamp = {2014.04.24}, }
- P. Pfaff, C. Stachniss, C. Plagemann, and W. Burgard, “Efficiently Learning High-dimensional Observation Models for Monte-Carlo Localization using Gaussian Mixtures,” in Proc. of the IEEE/RSJ Intl. Conf. on Intelligent Robots and Systems (IROS), Nice, France, 2008.
[BibTeX] [PDF]
[none]
@InProceedings{pfaff2008, title = {Efficiently Learning High-dimensional Observation Models for Monte-Carlo Localization using Gaussian Mixtures}, author = {Pfaff, P. and Stachniss, C. and Plagemann, C. and W. Burgard}, booktitle = iros, year = {2008}, address = {Nice, France}, abstract = {[none]}, timestamp = {2014.04.24}, url = {https://www.ipb.uni-bonn.de/wp-content/papercite-data/pdf/pfaff08iros.pdf}, }
- C. Plagemann, F. Endres, J. Hess, C. Stachniss, and W. Burgard, “Monocular Range Sensing: A Non-Parametric Learning Approach,” in Proc. of the IEEE Intl. Conf. on Robotics & Automation (ICRA), Pasadena, CA, USA, 2008.
[BibTeX] [PDF]
[none]
@InProceedings{plagemann2008, title = {Monocular Range Sensing: A Non-Parametric Learning Approach}, author = {Plagemann, C. and Endres, F. and Hess, J. and Stachniss, C. and Burgard, W.}, booktitle = icra, year = {2008}, address = {Pasadena, CA, USA}, abstract = {[none]}, timestamp = {2014.04.24}, url = {https://www.ipb.uni-bonn.de/wp-content/papercite-data/pdf/plagemann08icra.pdf}, }
- C. Stachniss, M. Bennewitz, G. Grisetti, S. Behnke, and W. Burgard, “How to Learn Accurate Grid Maps with a Humanoid,” in Proc. of the IEEE Intl. Conf. on Robotics & Automation (ICRA), Pasadena, CA, USA, 2008.
[BibTeX] [PDF]
[none]
@InProceedings{stachniss2008, title = {How to Learn Accurate Grid Maps with a Humanoid}, author = {Stachniss, C. and Bennewitz, M. and Grisetti, G. and Behnke, S. and Burgard, W.}, booktitle = icra, year = {2008}, address = {Pasadena, CA, USA}, abstract = {[none]}, timestamp = {2014.04.24}, url = {https://www.ipb.uni-bonn.de/wp-content/papercite-data/pdf/stachniss08icra.pdf}, }
- C. Stachniss, C. Plagemann, A. Lilienthal, and W. Burgard, “Gas Distribution Modeling using Sparse Gaussian Process Mixture Models,” in Proc. of Robotics: Science and Systems (RSS), Zurich, Switzerland, 2008.
[BibTeX] [PDF]
[none]
@InProceedings{stachniss2008a, title = {Gas Distribution Modeling using Sparse Gaussian Process Mixture Models}, author = {Stachniss, C. and Plagemann, C. and Lilienthal, A. and Burgard, W.}, booktitle = rss, year = {2008}, address = {Zurich, Switzerland}, note = {To appear}, abstract = {[none]}, timestamp = {2014.04.24}, url = {https://www.ipb.uni-bonn.de/wp-content/papercite-data/pdf/stachniss08rss.pdf}, }
- B. Steder, G. Grisetti, C. Stachniss, and W. Burgard, “Learning Visual Maps using Cameras and Inertial Sensors,” in Workshop on Robotic Perception, International Conf. on Computer Vision Theory and Applications, Funchal, Madeira, Portugal, 2008.
[BibTeX]
[none]
@InProceedings{steder2008, title = {Learning Visual Maps using Cameras and Inertial Sensors}, author = {Steder, B. and Grisetti, G. and Stachniss, C. and Burgard, W.}, booktitle = {Workshop on Robotic Perception, International Conf. on Computer Vision Theory and Applications}, year = {2008}, address = {Funchal, Madeira, Portugal}, note = {To appear}, abstract = {[none]}, timestamp = {2014.04.24}, }
- K. M. Wurm, C. Stachniss, and W. Burgard, “Coordinated Multi-Robot Exploration using a Segmentation of the Environment,” in Proc. of the IEEE/RSJ Intl. Conf. on Intelligent Robots and Systems (IROS), Nice, France, 2008.
[BibTeX] [PDF]
[none]
@InProceedings{wurm2008, title = {Coordinated Multi-Robot Exploration using a Segmentation of the Environment}, author = {K.M. Wurm and Stachniss, C. and W. Burgard}, booktitle = iros, year = {2008}, address = {Nice, France}, abstract = {[none]}, timestamp = {2014.04.24}, url = {https://www.ipb.uni-bonn.de/wp-content/papercite-data/pdf/wurm08iros.pdf}, }
2007
- W. Burgard, C. Stachniss, and D. Haehnel, “Mobile Robot Map Learning from Range Data in Dynamic Environments,” in Autonomous Navigation in Dynamic Environments, C. Laugier and R. Chatila, Eds., Springer, 2007, vol. 35.
[BibTeX]
[none]
@InCollection{burgard2007, title = {Mobile Robot Map Learning from Range Data in Dynamic Environments}, author = {Burgard, W. and Stachniss, C. and Haehnel, D.}, booktitle = {Autonomous Navigation in Dynamic Environments}, publisher = springer, year = {2007}, editor = {Laugier, C. and Chatila, R.}, series = springerstaradvanced, volume = {35}, abstract = {[none]}, timestamp = {2014.04.24}, }
- G. Grisetti, S. Grzonka, C. Stachniss, P. Pfaff, and W. Burgard, “Efficient Estimation of Accurate Maximum Likelihood Maps in 3D,” in Proc. of the IEEE/RSJ Intl. Conf. on Intelligent Robots and Systems (IROS), San Diego, CA, USA, 2007.
[BibTeX] [PDF]
[none]
@InProceedings{grisetti2007c, title = {Efficient Estimation of Accurate Maximum Likelihood Maps in 3D}, author = {Grisetti, G. and Grzonka, S. and Stachniss, C. and Pfaff, P. and Burgard, W.}, booktitle = iros, year = {2007}, address = {San Diego, CA, USA}, abstract = {[none]}, timestamp = {2014.04.24}, url = {https://www.ipb.uni-bonn.de/wp-content/papercite-data/pdf/grisetti07iros.pdf}, }
- G. Grisetti, C. Stachniss, and W. Burgard, “Improved Techniques for Grid Mapping with Rao-Blackwellized Particle Filters,” IEEE Transactions on Robotics, vol. 23, iss. 1, p. 34–46, 2007.
[BibTeX] [PDF]
[none]
@Article{grisetti2007a, title = {Improved Techniques for Grid Mapping with Rao-Blackwellized Particle Filters}, author = {Grisetti, G. and Stachniss, C. and Burgard, W.}, journal = ieeetransrob, year = {2007}, number = {1}, pages = {34--46}, volume = {23}, abstract = {[none]}, timestamp = {2014.04.24}, url = {https://www.ipb.uni-bonn.de/wp-content/papercite-data/pdf/grisetti07tro.pdf}, }
- G. Grisetti, C. Stachniss, S. Grzonka, and W. Burgard, “A Tree Parameterization for Efficiently Computing Maximum Likelihood Maps using Gradient Descent,” in Proc. of Robotics: Science and Systems (RSS), Atlanta, GA, USA, 2007.
[BibTeX] [PDF]
[none]
@InProceedings{grisetti2007b, title = {A Tree Parameterization for Efficiently Computing Maximum Likelihood Maps using Gradient Descent}, author = {Grisetti, G. and Stachniss, C. and Grzonka, S. and Burgard, W.}, booktitle = rss, year = {2007}, address = {Atlanta, GA, USA}, abstract = {[none]}, timestamp = {2014.04.24}, url = {https://www.ipb.uni-bonn.de/wp-content/papercite-data/pdf/grisetti07rss.pdf}, }
- G. Grisetti, G. D. Tipaldi, C. Stachniss, W. Burgard, and D. Nardi, “Fast and Accurate SLAM with Rao-Blackwellized Particle Filters,” Robotics and Autonomous Systems, vol. 55, iss. 1, p. 30–38, 2007.
[BibTeX] [PDF]
[none]
@Article{grisetti2007, title = {Fast and Accurate {SLAM} with Rao-Blackwellized Particle Filters}, author = {Grisetti, G. and Tipaldi, G.D. and Stachniss, C. and Burgard, W. and Nardi, D.}, journal = jras, year = {2007}, number = {1}, pages = {30--38}, volume = {55}, abstract = {[none]}, timestamp = {2014.04.24}, url = {https://www.ipb.uni-bonn.de/wp-content/papercite-data/pdf/grisetti07jras.pdf}, }
- D. Joho, C. Stachniss, P. Pfaff, and W. Burgard, “Autonomous Exploration for 3D Map Learning,” in Autonome Mobile Systeme, Kaiserslautern, Germany, 2007.
[BibTeX] [PDF]
[none]
@InProceedings{joho2007, title = {Autonomous Exploration for 3D Map Learning}, author = {Joho, D. and Stachniss, C. and Pfaff, P. and Burgard, W.}, booktitle = ams, year = {2007}, address = {Kaiserslautern, Germany}, abstract = {[none]}, timestamp = {2014.04.24}, url = {https://www.ipb.uni-bonn.de/wp-content/papercite-data/pdf/joho07ams.pdf}, }
- O. Martínez-Mozos, C. Stachniss, A. Rottmann, and W. Burgard, “Using AdaBoost for Place Labelling and Topological Map Building,” in Robotics Research, S. Thrun, R. Brooks, and H. Durrant-Whyte, Eds., Springer, 2007, vol. 28.
[BibTeX] [PDF]
[none]
@InCollection{martinez-mozos2007, title = {Using AdaBoost for Place Labelling and Topological Map Building}, author = {Mart\'{i}nez-Mozos, O. and Stachniss, C. and Rottmann, A. and Burgard, W.}, booktitle = {Robotics Research}, publisher = springer, year = {2007}, editor = {Thrun, S. and Brooks, R. and Durrant-Whyte, H.}, series = springerstaradvanced, volume = {28}, abstract = {[none]}, isbn = {978-3-540-48110-2}, timestamp = {2014.04.24}, url = {https://www.ipb.uni-bonn.de/wp-content/papercite-data/pdf/martinez07springer.pdf}, }
- P. Pfaff, R. Kuemmerle, D. Joho, C. Stachniss, R. Triebel, and Burgard, “Navigation in Combined Outdoor and Indoor Environments using Multi-Level Surface Maps,” in Workshop on Safe Navigation in Open and Dynamic Environments at the IEEE/RSJ Int. Conf. on Intelligent Robots and Systems, San Diego, CA, USA, 2007.
[BibTeX] [PDF]
[none]
@InProceedings{pfaff2007a, title = {Navigation in Combined Outdoor and Indoor Environments using Multi-Level Surface Maps}, author = {Pfaff, P. and Kuemmerle, R. and Joho, D. and Stachniss, C. and Triebel, R. and Burgard}, booktitle = iroswsnav, year = {2007}, address = {San Diego, CA, USA}, abstract = {[none]}, timestamp = {2014.04.24}, url = {https://www.ipb.uni-bonn.de/wp-content/papercite-data/pdf/pfaff07irosws.pdf}, }
- P. Pfaff, R. Triebel, C. Stachniss, P. Lamon, W. Burgard, and R. Siegwart, “Towards Mapping of Cities,” in Proc. of the IEEE Intl. Conf. on Robotics & Automation (ICRA), Rome, Italy, 2007.
[BibTeX] [PDF]
[none]
@InProceedings{pfaff2007, title = {Towards Mapping of Cities}, author = {Pfaff, P. and Triebel, R. and Stachniss, C. and Lamon, P. and Burgard, W. and Siegwart, R.}, booktitle = icra, year = {2007}, address = {Rome, Italy}, abstract = {[none]}, timestamp = {2014.04.24}, url = {https://www.ipb.uni-bonn.de/wp-content/papercite-data/pdf/pfaff07icra.pdf}, }
- C. Stachniss, G. Grisetti, W. Burgard, and N. Roy, “Evaluation of Gaussian Proposal Distributions for Mapping with Rao-Blackwellized Particle Filters,” in Proc. of the IEEE/RSJ Intl. Conf. on Intelligent Robots and Systems (IROS), San Diego, CA, USA, 2007.
[BibTeX] [PDF]
[none]
@InProceedings{stachniss2007a, title = {Evaluation of Gaussian Proposal Distributions for Mapping with Rao-Blackwellized Particle Filters}, author = {Stachniss, C. and Grisetti, G. and Burgard, W. and Roy, N.}, booktitle = iros, year = {2007}, address = {San Diego, CA, USA}, abstract = {[none]}, timestamp = {2014.04.24}, url = {https://www.ipb.uni-bonn.de/wp-content/papercite-data/pdf/stachniss07iros.pdf}, }
- C. Stachniss, G. Grisetti, O. Martínez-Mozos, and W. Burgard, “Efficiently Learning Metric and Topological Maps with Autonomous Service Robots,” it – Information Technology, vol. 49, iss. 4, p. 232–238, 2007.
[BibTeX]
[none]
@Article{stachniss2007, title = {Efficiently Learning Metric and Topological Maps with Autonomous Service Robots}, author = {Stachniss, C. and Grisetti, G. and Mart\'{i}nez-Mozos, O. and Burgard, W.}, journal = {it -- Information Technology}, year = {2007}, number = {4}, pages = {232--238}, volume = {49}, abstract = {[none]}, editor = {Buss, M. and Lawitzki, G.}, timestamp = {2014.04.24}, }
- B. Steder, G. Grisetti, S. Grzonka, C. Stachniss, A. Rottmann, and W. Burgard, “Learning Maps in 3D using Attitude and Noisy Vision Sensors,” in Proc. of the IEEE/RSJ Intl. Conf. on Intelligent Robots and Systems (IROS), San Diego, CA, USA, 2007.
[BibTeX] [PDF]
[none]
@InProceedings{steder2007, title = {Learning Maps in 3D using Attitude and Noisy Vision Sensors}, author = {Steder, B. and Grisetti, G. and Grzonka, S. and Stachniss, C. and Rottmann, A. and Burgard, W.}, booktitle = iros, year = {2007}, address = {San Diego, CA, USA}, abstract = {[none]}, timestamp = {2014.04.24}, url = {https://www.ipb.uni-bonn.de/wp-content/papercite-data/pdf/steder07iros.pdf}, }
- B. Steder, A. Rottmann, G. Grisetti, C. Stachniss, and W. Burgard, “Autonomous Navigation for Small Flying Vehicles,” in Workshop on Micro Aerial Vehicles at the IEEE/RSJ Int. Conf. on Intelligent Robots and Systems, San Diego, CA, USA, 2007.
[BibTeX] [PDF]
[none]
@InProceedings{steder, title = {Autonomous Navigation for Small Flying Vehicles}, author = {Steder, B. and Rottmann, A. and Grisetti, G. and Stachniss, C. and Burgard, W.}, booktitle = iroswsfly, year = {2007}, address = {San Diego, CA, USA}, abstract = {[none]}, timestamp = {2014.04.24}, url = {https://www.informatik.uni-freiburg.de/~steder/publications/steder07irosws.pdf}, }
- H. Strasdat, C. Stachniss, M. Bennewitz, and W. Burgard, “Visual Bearing-Only Simultaneous Localization and Mapping with Improved Feature Matching,” in Autonome Mobile Systeme, Kaiserslautern, Germany, 2007.
[BibTeX] [PDF]
[none]
@InProceedings{strasdat2007, title = {Visual Bearing-Only Simultaneous Localization and Mapping with Improved Feature Matching}, author = {Strasdat, H. and Stachniss, C. and Bennewitz, M. and Burgard, W.}, booktitle = ams, year = {2007}, address = {Kaiserslautern, Germany}, abstract = {[none]}, timestamp = {2014.04.24}, url = {https://www.ipb.uni-bonn.de/wp-content/papercite-data/pdf/strasdat07ams.pdf}, }
- K. M. Wurm, C. Stachniss, G. Grisetti, and W. Burgard, “Improved Simultaneous Localization and Mapping using a Dual Representation of the Environment,” in Proc. of the European Conf. on Mobile Robots (ECMR), Freiburg, Germany, 2007.
[BibTeX] [PDF]
[none]
@InProceedings{wurm2007, title = {Improved Simultaneous Localization and Mapping using a Dual Representation of the Environment}, author = {Wurm, K.M. and Stachniss, C. and Grisetti, G. and Burgard, W.}, booktitle = ecmr, year = {2007}, address = {Freiburg, Germany}, abstract = {[none]}, timestamp = {2014.04.24}, url = {https://www.ipb.uni-bonn.de/wp-content/papercite-data/pdf/wurm07ecmr.pdf}, }
2006
- M. Bennewitz, C. Stachniss, W. Burgard, and S. Behnke, “Metric Localization with Scale-Invariant Visual Features using a Single Perspective Camera,” in European Robotics Symposium 2006, 2006, p. 143–157.
[BibTeX] [PDF]
[none]
@InProceedings{bennewitz2006, title = {Metric Localization with Scale-Invariant Visual Features using a Single Perspective Camera}, author = {Bennewitz, M. and Stachniss, C. and Burgard, W. and Behnke, S.}, booktitle = {European Robotics Symposium 2006}, year = {2006}, editor = {H.I. Christiensen}, pages = {143--157}, publisher = {Springer-Verlag Berlin Heidelberg, Germany}, series = springerstaradvanced, volume = {22}, abstract = {[none]}, isbn = {3-540-32688-X}, timestamp = {2014.04.24}, url = {https://www.ipb.uni-bonn.de/wp-content/papercite-data/pdf/bennewitz06euros.pdf}, }
- A. Gil, O. Reinoso, O. Martínez-Mozos, C. Stachniss, and W. Burgard, “Improving Data Association in Vision-based SLAM,” in Proc. of the IEEE/RSJ Intl. Conf. on Intelligent Robots and Systems (IROS), Beijing, China, 2006.
[BibTeX]
[none]
@InProceedings{gil2006, title = {Improving Data Association in Vision-based {SLAM}}, author = {Gil, A. and Reinoso, O. and Mart\'{i}nez-Mozos, O. and Stachniss, C. and Burgard, W.}, booktitle = iros, year = {2006}, address = {Beijing, China}, abstract = {[none]}, timestamp = {2014.04.24}, }
- G. Grisetti, G. D. Tipaldi, C. Stachniss, W. Burgard, and D. Nardi, “Speeding-Up Rao-Blackwellized SLAM,” in Proc. of the IEEE Intl. Conf. on Robotics & Automation (ICRA), Orlando, FL, USA, 2006, p. 442–447.
[BibTeX] [PDF]
[none]
@InProceedings{grisetti2006, title = {Speeding-Up Rao-Blackwellized {SLAM}}, author = {Grisetti, G. and Tipaldi, G.D. and Stachniss, C. and Burgard, W. and Nardi, D.}, booktitle = icra, year = {2006}, address = {Orlando, FL, USA}, pages = {442--447}, abstract = {[none]}, timestamp = {2014.04.24}, url = {https://www.ipb.uni-bonn.de/wp-content/papercite-data/pdf/grisetti06icra.pdf}, }
- P. Lamon, C. Stachniss, R. Triebel, P. Pfaff, C. Plagemann, G. Grisetti, S. Kolski, W. Burgard, and R. Siegwart, “Mapping with an Autonomous Car,” in Workshop on Safe Navigation in Open and Dynamic Environments at the IEEE/RSJ Int. Conf. on Intelligent Robots and Systems, Beijing, China, 2006.
[BibTeX] [PDF]
[none]
@InProceedings{lamon2006, title = {Mapping with an Autonomous Car}, author = {Lamon, P. and Stachniss, C. and Triebel, R. and Pfaff, P. and Plagemann, C. and Grisetti, G. and Kolski, S. and Burgard, W. and Siegwart, R.}, booktitle = iroswsnav, year = {2006}, address = {Beijing, China}, abstract = {[none]}, timestamp = {2014.04.24}, url = {https://www.ipb.uni-bonn.de/wp-content/papercite-data/pdf/lamon06iros.pdf}, }
- D. Meier, C. Stachniss, and W. Burgard, “Cooperative Exploration With Multiple Robots Using Low Bandwidth Communication,” in Informationsfusion in der Mess- und Sensortechnik, 2006, p. 145–157.
[BibTeX] [PDF]
[none]
@InProceedings{meier2006, title = {Cooperative Exploration With Multiple Robots Using Low Bandwidth Communication}, author = {Meier, D. and Stachniss, C. and Burgard, W.}, booktitle = {Informationsfusion in der Mess- und Sensortechnik}, year = {2006}, editor = {Beyerer, J. and Puente Le\'{o}n, F. and Sommer, K.-D.}, pages = {145--157}, abstract = {[none]}, isbn = {3-86644-053-7}, timestamp = {2014.04.24}, url = {https://www.ipb.uni-bonn.de/wp-content/papercite-data/pdf/meier06sensor.pdf}, }
- C. Plagemann, C. Stachniss, and W. Burgard, “Efficient Failure Detection for Mobile Robots using Mixed-Abstraction Particle Filters,” in European Robotics Symposium 2006, 2006, p. 93–107.
[BibTeX] [PDF]
[none]
@InProceedings{plagemann2006, title = {Efficient Failure Detection for Mobile Robots using Mixed-Abstraction Particle Filters}, author = {Plagemann, C. and Stachniss, C. and Burgard, W.}, booktitle = {European Robotics Symposium 2006}, year = {2006}, editor = {H.I. Christiensen}, pages = {93--107}, publisher = {Springer-Verlag Berlin Heidelberg, Germany}, series = springerstaradvanced, volume = {22}, abstract = {[none]}, isbn = {3-540-32688-X}, timestamp = {2014.04.24}, url = {https://www.ipb.uni-bonn.de/wp-content/papercite-data/pdf/plagemann06euros.pdf}, }
- D. Sonntag, S. Stachniss-Carp, C. Stachniss, and V. Stachniss, “Determination of Root Canal Curvatures before and after Canal Preparation (Part II): A Method based on Numeric Calculus,” Aust Endod J, vol. 32, p. 16–25, 2006.
[BibTeX] [PDF]
[none]
@Article{sonntag2006, title = {Determination of Root Canal Curvatures before and after Canal Preparation (Part II): A Method based on Numeric Calculus}, author = {Sonntag, D. and Stachniss-Carp, S. and Stachniss, C. and Stachniss, V.}, journal = {Aust Endod J}, year = {2006}, pages = {16--25}, volume = {32}, abstract = {[none]}, timestamp = {2014.04.24}, url = {https://www.ipb.uni-bonn.de/wp-content/papercite-data/pdf/sonntag06endod.pdf}, }
- C. Stachniss, “Exploration and Mapping with Mobile Robots,” PhD Thesis, 2006.
[BibTeX] [PDF]
[none]
@PhDThesis{stachniss2006a, title = {Exploration and Mapping with Mobile Robots}, author = {Stachniss, C.}, school = {University of Freiburg, Department of Computer Science}, year = {2006}, abstract = {[none]}, timestamp = {2014.04.24}, url = {https://www.ipb.uni-bonn.de/wp-content/papercite-data/pdf/stachniss06phd.pdf}, }
- C. Stachniss, O. Martínez-Mozos, and W. Burgard, “Speeding-Up Multi-Robot Exploration by Considering Semantic Place Information,” in Proc. of the IEEE Intl. Conf. on Robotics & Automation (ICRA), Orlando, FL, USA, 2006, p. 1692–1697.
[BibTeX] [PDF]
[none]
@InProceedings{stachniss2006, title = {Speeding-Up Multi-Robot Exploration by Considering Semantic Place Information}, author = {Stachniss, C. and Mart\'{i}nez-Mozos, O. and Burgard, W.}, booktitle = icra, year = {2006}, address = {Orlando, FL, USA}, pages = {1692--1697}, abstract = {[none]}, timestamp = {2014.04.24}, url = {https://www.ipb.uni-bonn.de/wp-content/papercite-data/pdf/stachniss06icra.pdf}, }
2005
- W. Burgard, M. Moors, C. Stachniss, and F. Schneider, “Coordinated Multi-Robot Exploration,” IEEE Transactions on Robotics, vol. 21, iss. 3, p. 376–378, 2005.
[BibTeX] [PDF]
[none]
@Article{burgard2005a, title = {Coordinated Multi-Robot Exploration}, author = {W. Burgard and M. Moors and C. Stachniss and F. Schneider}, journal = ieeetransrob, year = {2005}, number = {3}, pages = {376--378}, volume = {21}, abstract = {[none]}, timestamp = {2014.04.24}, url = {https://www.ipb.uni-bonn.de/wp-content/papercite-data/pdf/burgard05tro.pdf}, }
- W. Burgard, C. Stachniss, and G. Grisetti, “Information Gain-based Exploration Using Rao-Blackwellized Particle Filters,” in Proc. of the Learning Workshop (Snowbird), Snowbird, UT, USA, 2005.
[BibTeX] [PDF]
[none]
@InProceedings{burgard2005, title = {Information Gain-based Exploration Using Rao-Blackwellized Particle Filters}, author = {Burgard, W. and Stachniss, C. and Grisetti, G.}, booktitle = {Proc. of the Learning Workshop (Snowbird)}, year = {2005}, address = {Snowbird, UT, USA}, abstract = {[none]}, timestamp = {2014.04.24}, url = {https://www.ipb.uni-bonn.de/wp-content/papercite-data/pdf/burgard05snowbird.pdf}, }
- G. Grisetti, C. Stachniss, and W. Burgard, “Improving Grid-based SLAM with Rao-Blackwellized Particle Filters by Adaptive Proposals and Selective Resampling,” in Proc. of the IEEE Intl. Conf. on Robotics & Automation (ICRA), Barcelona, Spain, 2005, p. 2443–2448.
[BibTeX] [PDF]
[none]
@InProceedings{grisetti2005, title = {Improving Grid-based {SLAM} with Rao-Blackwellized Particle Filters by Adaptive Proposals and Selective Resampling}, author = {Grisetti, G. and Stachniss, C. and Burgard, W.}, booktitle = icra, year = {2005}, address = {Barcelona, Spain}, pages = {2443--2448}, abstract = {[none]}, timestamp = {2014.04.24}, url = {https://www.ipb.uni-bonn.de/wp-content/papercite-data/pdf/grisetti05icra.pdf}, }
- O. Martínez-Mozos, C. Stachniss, and W. Burgard, “Supervised Learning of Places from Range Data using Adaboost,” in Proc. of the IEEE Intl. Conf. on Robotics & Automation (ICRA), Barcelona, Spain, 2005, p. 1742–1747.
[BibTeX] [PDF]
[none]
@InProceedings{martinez-mozos2005, title = {Supervised Learning of Places from Range Data using Adaboost}, author = {Mart\'{i}nez-Mozos, O. and Stachniss, C. and W. Burgard}, booktitle = icra, year = {2005}, address = {Barcelona, Spain}, pages = {1742--1747}, abstract = {[none]}, timestamp = {2014.04.24}, url = {https://www.ipb.uni-bonn.de/wp-content/papercite-data/pdf/martinez05icra.pdf}, }
- D. Meier, C. Stachniss, and W. Burgard, “Coordinating Multiple Robots During Exploration Under Communication With Limited Bandwidth,” in Proc. of the European Conf. on Mobile Robots (ECMR), Ancona, Italy, 2005, p. 26–31.
[BibTeX] [PDF]
[none]
@InProceedings{meier2005, title = {Coordinating Multiple Robots During Exploration Under Communication With Limited Bandwidth}, author = {Meier, D. and Stachniss, C. and Burgard, W.}, booktitle = ecmr, year = {2005}, address = {Ancona, Italy}, pages = {26--31}, abstract = {[none]}, timestamp = {2014.04.24}, url = {https://www.ipb.uni-bonn.de/wp-content/papercite-data/pdf/meier05ecmr.pdf}, }
- A. Rottmann, O. Martínez-Mozos, C. Stachniss, and W. Burgard, “Place Classification of Indoor Environments with Mobile Robots using Boosting,” in Proc. of the National Conf. on Artificial Intellience (AAAI), Pittsburgh, PA, USA, 2005, p. 1306–1311.
[BibTeX] [PDF]
[none]
@InProceedings{rottmann2005, title = {Place Classification of Indoor Environments with Mobile Robots using Boosting}, author = {Rottmann, A. and Mart\'{i}nez-Mozos, O. and Stachniss, C. and Burgard, W.}, booktitle = aaai, year = {2005}, address = {Pittsburgh, PA, USA}, pages = {1306--1311}, abstract = {[none]}, timestamp = {2014.04.24}, url = {https://www.ipb.uni-bonn.de/wp-content/papercite-data/pdf/rottmann05aaai.pdf}, }
- C. Stachniss and W. Burgard, “Mobile Robot Mapping and Localization in Non-Static Environments,” in Proc. of the National Conf. on Artificial Intellience (AAAI), Pittsburgh, PA, USA, 2005, p. 1324–1329.
[BibTeX] [PDF]
[none]
@InProceedings{stachniss2005, title = {Mobile Robot Mapping and Localization in Non-Static Environments}, author = {Stachniss, C. and Burgard, W.}, booktitle = aaai, year = {2005}, address = {Pittsburgh, PA, USA}, pages = {1324--1329}, abstract = {[none]}, timestamp = {2014.04.24}, url = {https://www.ipb.uni-bonn.de/wp-content/papercite-data/pdf/stachniss05aaai.pdf}, }
- C. Stachniss, G. Grisetti, and W. Burgard, “Information Gain-based Exploration Using Rao-Blackwellized Particle Filters,” in Proc. of Robotics: Science and Systems (RSS), Cambridge, MA, USA, 2005, p. 65–72.
[BibTeX] [PDF]
[none]
@InProceedings{stachniss2005a, title = {Information Gain-based Exploration Using Rao-Blackwellized Particle Filters}, author = {Stachniss, C. and Grisetti, G. and Burgard, W.}, booktitle = rss, year = {2005}, address = {Cambridge, MA, USA}, pages = {65--72}, abstract = {[none]}, timestamp = {2014.04.24}, url = {https://www.ipb.uni-bonn.de/wp-content/papercite-data/pdf/stachniss05rss.pdf}, }
- C. Stachniss, G. Grisetti, and W. Burgard, “Recovering Particle Diversity in a Rao-Blackwellized Particle Filter for SLAM after Actively Closing Loops,” in Proc. of the IEEE Intl. Conf. on Robotics & Automation (ICRA), Barcelona, Spain, 2005, p. 667–672.
[BibTeX] [PDF]
[none]
@InProceedings{stachniss2005d, title = {Recovering Particle Diversity in a Rao-Blackwellized Particle Filter for {SLAM} after Actively Closing Loops}, author = {Stachniss, C. and Grisetti, G. and Burgard, W.}, booktitle = icra, year = {2005}, address = {Barcelona, Spain}, pages = {667--672}, abstract = {[none]}, timestamp = {2014.04.24}, url = {https://www.ipb.uni-bonn.de/wp-content/papercite-data/pdf/stachniss05icra.pdf}, }
- C. Stachniss, D. Hähnel, W. Burgard, and G. Grisetti, “On Actively Closing Loops in Grid-based FastSLAM,” Advanced Robotics, vol. 19, iss. 10, p. 1059–1080, 2005.
[BibTeX] [PDF]
[none]
@Article{stachniss2005c, title = {On Actively Closing Loops in Grid-based {FastSLAM}}, author = {Stachniss, C. and H\"{a}hnel, D. and Burgard, W. and Grisetti, G.}, journal = advancedrobotics, year = {2005}, number = {10}, pages = {1059--1080}, volume = {19}, abstract = {[none]}, timestamp = {2014.04.24}, url = {https://www.ipb.uni-bonn.de/wp-content/papercite-data/pdf/stachniss05ar.pdf}, }
- C. Stachniss, O. Martínez-Mozos, A. Rottmann, and W. Burgard, “Semantic Labeling of Places,” in Proc. of the Intl. Symposium of Robotics Research (ISRR), San Francisco, CA, USA, 2005.
[BibTeX] [PDF]
[none]
@InProceedings{stachniss2005b, title = {Semantic Labeling of Places}, author = {Stachniss, C. and Mart\'{i}nez-Mozos, O. and Rottmann, A. and Burgard, W.}, booktitle = isrr, year = {2005}, address = {San Francisco, CA, USA}, abstract = {[none]}, timestamp = {2014.04.24}, url = {https://www.ipb.uni-bonn.de/wp-content/papercite-data/pdf/stachniss05isrr.pdf}, }
- P. Trahanias, W. Burgard, A. Argyros, D. Hähnel, H. Baltzakis, P. Pfaff, and C. Stachniss, “TOURBOT and WebFAIR: Web-Operated Mobile Robots for Tele-Presence in Populated Exhibitions,” IEEE Robotics & Automation Magazine, vol. 12, iss. 2, p. 77–89, 2005.
[BibTeX] [PDF]
[none]
@Article{trahanias2005, title = {{TOURBOT} and {WebFAIR}: Web-Operated Mobile Robots for Tele-Presence in Populated Exhibitions}, author = {Trahanias, P. and Burgard, W. and Argyros, A. and H\"{a}hnel, D. and Baltzakis, H. and Pfaff, P. and Stachniss, C.}, journal = {IEEE Robotics \& Automation Magazine}, year = {2005}, number = {2}, pages = {77--89}, volume = {12}, abstract = {[none]}, timestamp = {2014.04.24}, url = {https://ieeexplore.ieee.org/iel5/100/31383/01458329.pdf?arnumber=1458329}, }
2004
- C. Stachniss, G. Grisetti, D. Hähnel, and W. Burgard, “Improved Rao-Blackwellized Mapping by Adaptive Sampling and Active Loop-Closure,” in Proc. of the Workshop on Self-Organization of AdaptiVE behavior (SOAVE), Ilmenau, Germany, 2004, p. 1–15.
[BibTeX] [PDF]
[none]
@InProceedings{stachniss2004a, title = {Improved Rao-Blackwellized Mapping by Adaptive Sampling and Active Loop-Closure}, author = {Stachniss, C. and Grisetti, G. and H\"{a}hnel, D. and Burgard, W.}, booktitle = soave, year = {2004}, address = {Ilmenau, Germany}, pages = {1--15}, abstract = {[none]}, timestamp = {2014.04.24}, url = {https://www.ipb.uni-bonn.de/wp-content/papercite-data/pdf/stachniss04soave.pdf}, }
- C. Stachniss, D. Hähnel, and W. Burgard, “Exploration with Active Loop-Closing for FastSLAM,” in Proc. of the IEEE/RSJ Intl. Conf. on Intelligent Robots and Systems (IROS), Sendai, Japan, 2004, p. 1505–1510.
[BibTeX] [PDF]
[none]
@InProceedings{stachniss2004, title = {Exploration with Active Loop-Closing for {FastSLAM}}, author = {Stachniss, C. and H\"{a}hnel, D. and Burgard, W.}, booktitle = iros, year = {2004}, address = {Sendai, Japan}, pages = {1505--1510}, abstract = {[none]}, timestamp = {2014.04.24}, url = {https://www.ipb.uni-bonn.de/wp-content/papercite-data/pdf/stachniss04iros.pdf}, }
2003
- C. Stachniss and W. Burgard, “Exploring Unknown Environments with Mobile Robots using Coverage Maps,” in Proc. of the Intl. Conf. on Artificial Intelligence (IJCAI), Acapulco, Mexico, 2003, p. 1127–1132.
[BibTeX] [PDF]
[none]
@InProceedings{stachniss2003, title = {Exploring Unknown Environments with Mobile Robots using Coverage Maps}, author = {Stachniss, C. and Burgard, W.}, booktitle = ijcai, year = {2003}, address = {Acapulco, Mexico}, pages = {1127--1132}, abstract = {[none]}, timestamp = {2014.04.24}, url = {https://www.ipb.uni-bonn.de/wp-content/papercite-data/pdf/stachniss03ijcai.pdf}, }
- C. Stachniss and W. Burgard, “Using Coverage Maps to Represent the Environment of Mobile Robots,” in Proc. of the European Conf. on Mobile Robots (ECMR), Radziejowice, Poland, 2003, p. 59–64.
[BibTeX] [PDF]
[none]
@InProceedings{stachniss2003a, title = {Using Coverage Maps to Represent the Environment of Mobile Robots}, author = {Stachniss, C. and Burgard, W.}, booktitle = ecmr, year = {2003}, address = {Radziejowice, Poland}, pages = {59--64}, abstract = {[none]}, timestamp = {2014.04.24}, url = {https://www.ipb.uni-bonn.de/wp-content/papercite-data/pdf/stachniss03ecmr.pdf}, }
- C. Stachniss and W. Burgard, “Mapping and Exploration with Mobile Robots using Coverage Maps,” in Proc. of the IEEE/RSJ Intl. Conf. on Intelligent Robots and Systems (IROS), Las Vegas, NV, USA, 2003, p. 476–481.
[BibTeX] [PDF]
[none]
@InProceedings{stachniss2003b, title = {Mapping and Exploration with Mobile Robots using Coverage Maps}, author = {Stachniss, C. and Burgard, W.}, booktitle = iros, year = {2003}, address = {Las Vegas, NV, USA}, pages = {476--481}, abstract = {[none]}, timestamp = {2014.04.24}, url = {https://www.ipb.uni-bonn.de/wp-content/papercite-data/pdf/stachniss03iros.pdf}, }
- C. Stachniss, D. Hähnel, and W. Burgard, “Grid-based FastSLAM and Exploration with Active Loop Closing,” in Online Proc. of the Dagstuhl Seminar on Robot Navigation (Dagstuhl Seminar 03501), Dagstuhl, Germany, 2003.
[BibTeX]
[none]
@InProceedings{stachniss2003c, title = {Grid-based {FastSLAM} and Exploration with Active Loop Closing}, author = {Stachniss, C. and H\"{a}hnel, D. and Burgard, W.}, booktitle = {Online Proc. of the Dagstuhl Seminar on Robot Navigation (Dagstuhl Seminar 03501)}, year = {2003}, address = {Dagstuhl, Germany}, abstract = {[none]}, timestamp = {2014.04.24}, }
2002
- C. Stachniss, “Zielgerichtete Kollisionsvermeidung für mobile Roboter in dynamischen Umgebungen,” Master Thesis, 2002.
[BibTeX] [PDF]
[none]
@MastersThesis{stachniss2002, title = {{Z}ielgerichtete {K}ollisionsvermeidung f{\"u}r mobile {R}oboter in dynamischen {U}mgebungen}, author = {Stachniss, C.}, school = {University of Freiburg, Department of Computer Science}, year = {2002}, note = {In German}, abstract = {[none]}, timestamp = {2014.04.24}, url = {https://www.ipb.uni-bonn.de/wp-content/papercite-data/pdf/stachniss02diplom.pdf}, }
- C. Stachniss and W. Burgard, “An Integrated Approach to Goal-directed Obstacle Avoidance under Dynamic Constraints for Dynamic Environments,” in Proc. of the IEEE/RSJ Intl. Conf. on Intelligent Robots and Systems (IROS), Lausanne, Switzerland, 2002, p. 508–513.
[BibTeX] [PDF]
[none]
@InProceedings{stachniss2002a, title = {An Integrated Approach to Goal-directed Obstacle Avoidance under Dynamic Constraints for Dynamic Environments}, author = {Stachniss, C. and Burgard, W.}, booktitle = iros, year = {2002}, address = {Lausanne, Switzerland}, pages = {508--513}, abstract = {[none]}, timestamp = {2014.04.24}, url = {https://www.ipb.uni-bonn.de/wp-content/papercite-data/pdf/stachniss02iros.pdf}, }