Andres Milioto
PhD Student (Graduated 2021) Contact:Email: amilioto@nulluni-bonn.de
Tel: +49 – 228 – 73 –
Office: Nussallee 15,
Address:
University of Bonn
Photogrammetry, IGG
Nussallee 15
53115 Bonn
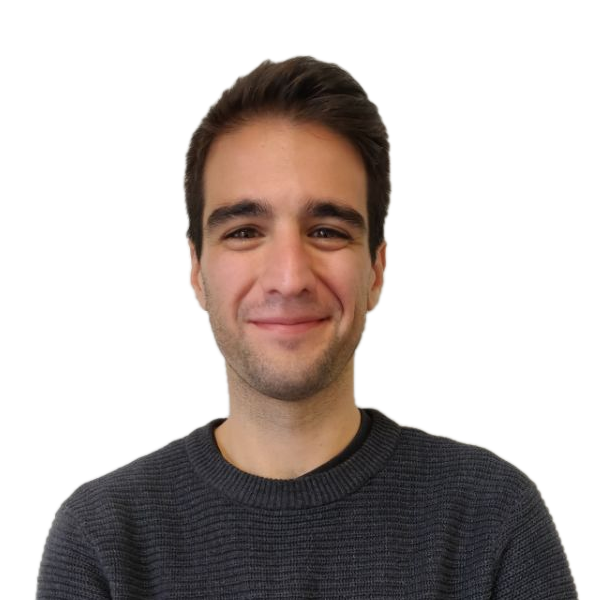
Links
Google Scholar | LinkedIn | Research Gate | Github
Research Interests
- Deep Learning for Robotics
- Computer Vision for Robotics
- Artificial Intelligence
- Real-time stuff
Projects
- Flourish – Developing computer vision algorithms to detect crops and weeds in the context of autonomous precision agriculture robots. Focusing on novel machine learning algorithms to aid autonomy of agriculture robotics solutions.
- Bonnet – an open-source training and deployment framework for semantic segmentation in robotics.
StarForkWatch - Bonnetal – Another open-source training and deployment framework for perception in robotics.
StarForkWatch - SemanticKitti: A Dataset for Semantic Segmentation of Point Cloud Sequences: .
- Lidar-Bonnetal – And open-source library for training and deploying semantic and instance segmentation using LiDAR point clouds from rotating sensor.
StarForkWatch - Alfred: Leading development effort of autonomous multi-sensor robotic platform based on Clearpath Husky A200.
- goPro-meta – Software for extracting meta-data from goPro Hero5 cameras, such as GPS information, for each frame.
StarForkWatch
Short CV
Andres Milioto is a Research Assistant and Ph.D. Student at the University of Bonn since February 2017. He received his Electrical Engineering Degree from Universidad Nacional de Rosario, Argentina in June 2016, where he was best of his class. During this time, he was involved in several robotics projects for private companies, including the construction of a large-scale iron pellet stacker and software development for robotics arms in welding applications, in Argentina, Mexico, and Italy. The 2 years preceding his Ph.D. he worked for iRobot (USA) on Software development and Hardware integration, developing behaviors and communication protocols for state-of-the-art, SLAM-enabled, consumer robots.Teaching
Awards
- Finalist “Best Systems Paper” RSS 2020.
- Winner “Best Demo” Award (Bonnet), Workshop on Multimodal Robot Perception, ICRA 2018, Brisbane, Australia.
- Finalist “IEEE ICRA Best Paper Award in Service Robotics” ICRA 2018, Brisbane, Australia.
- Best in 2015/2016 class, Electrical Engineering, Universidad Nacional de Rosario, Argentina.
Publications
2021
- J. Behley, M. Garbade, A. Milioto, J. Quenzel, S. Behnke, J. Gall, and C. Stachniss, “Towards 3D LiDAR-based semantic scene understanding of 3D point cloud sequences: The SemanticKITTI Dataset,” Intl. Journal of Robotics Research (IJRR), vol. 40, iss. 8-9, pp. 959-967, 2021. doi:10.1177/02783649211006735
[BibTeX] [PDF]@article{behley2021ijrr, author = {J. Behley and M. Garbade and A. Milioto and J. Quenzel and S. Behnke and J. Gall and C. Stachniss}, title = {Towards 3D LiDAR-based semantic scene understanding of 3D point cloud sequences: The SemanticKITTI Dataset}, journal = ijrr, volume = {40}, number = {8-9}, pages = {959-967}, year = {2021}, doi = {10.1177/02783649211006735}, url = {https://www.ipb.uni-bonn.de/pdfs/behley2021ijrr.pdf} }
- A. Pretto, S. Aravecchia, W. Burgard, N. Chebrolu, C. Dornhege, T. Falck, F. Fleckenstein, A. Fontenla, M. Imperoli, R. Khanna, F. Liebisch, P. Lottes, A. Milioto, D. Nardi, S. Nardi, J. Pfeifer, M. Popovic, C. Potena, C. Pradalier, E. Rothacker-Feder, I. Sa, A. Schaefer, R. Siegwart, C. Stachniss, A. Walter, V. Winterhalter, X. Wu, and J. Nieto, “Building an Aerial-Ground Robotics Systemfor Precision Farming: An Adaptable Solution,” IEEE Robotics & Automation Magazine, vol. 28, iss. 3, 2021.
[BibTeX] [PDF]@article{pretto2021ram, title = {{Building an Aerial-Ground Robotics Systemfor Precision Farming: An Adaptable Solution}}, author = {A. Pretto and S. Aravecchia and W. Burgard and N. Chebrolu and C. Dornhege and T. Falck and F. Fleckenstein and A. Fontenla and M. Imperoli and R. Khanna and F. Liebisch and P. Lottes and A. Milioto and D. Nardi and S. Nardi and J. Pfeifer and M. Popovic and C. Potena and C. Pradalier and E. Rothacker-Feder and I. Sa and A. Schaefer and R. Siegwart and C. Stachniss and A. Walter and V. Winterhalter and X. Wu and J. Nieto}, journal = ram, volume = 28, number = 3, year = {2021}, url = {https://www.ipb.uni-bonn.de/pdfs/pretto2021ram.pdf} }
- X. Chen, T. Läbe, A. Milioto, T. Röhling, J. Behley, and C. Stachniss, “OverlapNet: A Siamese Network for Computing LiDAR Scan Similarity with Applications to Loop Closing and Localization,” Autonomous Robots, vol. 46, p. 61–81, 2021. doi:10.1007/s10514-021-09999-0
[BibTeX] [PDF] [Code]@article{chen2021auro, author = {X. Chen and T. L\"abe and A. Milioto and T. R\"ohling and J. Behley and C. Stachniss}, title = {{OverlapNet: A Siamese Network for Computing LiDAR Scan Similarity with Applications to Loop Closing and Localization}}, journal = {Autonomous Robots}, year = {2021}, doi = {10.1007/s10514-021-09999-0}, issn = {1573-7527}, volume = 46, pages = {61--81}, codeurl = {https://github.com/PRBonn/OverlapNet}, url = {https://www.ipb.uni-bonn.de/pdfs/chen2021auro.pdf} }
- P. Rottmann, T. Posewsky, A. Milioto, C. Stachniss, and J. Behley, “Improving Monocular Depth Estimation by Semantic Pre-training,” in Proc. of the IEEE/RSJ Intl. Conf. on Intelligent Robots and Systems (IROS), 2021.
[BibTeX] [PDF]@inproceedings{rottmann2021iros, title = {{Improving Monocular Depth Estimation by Semantic Pre-training}}, author = {P. Rottmann and T. Posewsky and A. Milioto and C. Stachniss and J. Behley}, booktitle = iros, year = {2021}, url = {https://www.ipb.uni-bonn.de/pdfs/rottmann2021iros.pdf} }
- J. Behley, A. Milioto, and C. Stachniss, “A Benchmark for LiDAR-based Panoptic Segmentation based on KITTI,” in Proc. of the IEEE Intl. Conf. on Robotics & Automation (ICRA), 2021.
[BibTeX] [PDF]@inproceedings{behley2021icra, author = {J. Behley and A. Milioto and C. Stachniss}, title = {{A Benchmark for LiDAR-based Panoptic Segmentation based on KITTI}}, booktitle = icra, year = 2021 }
- J. Weyler, A. Milioto, T. Falck, J. Behley, and C. Stachniss, “Joint Plant Instance Detection and Leaf Count Estimation for In-Field Plant Phenotyping,” IEEE Robotics and Automation Letters (RA-L), vol. 6, pp. 3599-3606, 2021. doi:10.1109/LRA.2021.3060712
[BibTeX] [PDF] [Video]@article{weyler2021ral, author = {J. Weyler and A. Milioto and T. Falck and J. Behley and C. Stachniss}, title = {{Joint Plant Instance Detection and Leaf Count Estimation for In-Field Plant Phenotyping}}, journal = ral, volume = 6, issue = 2, pages = {3599-3606}, doi = {10.1109/LRA.2021.3060712}, year = 2021, videourl = {https://youtu.be/Is18Rey625I} }
- L. Wiesmann, A. Milioto, X. Chen, C. Stachniss, and J. Behley, “Deep Compression for Dense Point Cloud Maps,” IEEE Robotics and Automation Letters (RA-L), vol. 6, pp. 2060-2067, 2021. doi:10.1109/LRA.2021.3059633
[BibTeX] [PDF] [Code] [Video]@article{wiesmann2021ral, author = {L. Wiesmann and A. Milioto and X. Chen and C. Stachniss and J. Behley}, title = {{Deep Compression for Dense Point Cloud Maps}}, journal = ral, volume = 6, issue = 2, pages = {2060-2067}, doi = {10.1109/LRA.2021.3059633}, year = 2021, url = {https://www.ipb.uni-bonn.de/pdfs/wiesmann2021ral.pdf}, codeurl = {https://github.com/PRBonn/deep-point-map-compression}, videourl = {https://youtu.be/fLl9lTlZrI0} }
2020
- A. Milioto, J. Behley, C. McCool, and C. Stachniss, “LiDAR Panoptic Segmentation for Autonomous Driving,” in Proc. of the IEEE/RSJ Intl. Conf. on Intelligent Robots and Systems (IROS), 2020.
[BibTeX] [PDF] [Video]@inproceedings{milioto2020iros, author = {A. Milioto and J. Behley and C. McCool and C. Stachniss}, title = {{LiDAR Panoptic Segmentation for Autonomous Driving}}, booktitle = iros, year = {2020}, videourl = {https://www.youtube.com/watch?v=C9CTQSosr9I} }
- F. Langer, A. Milioto, A. Haag, J. Behley, and C. Stachniss, “Domain Transfer for Semantic Segmentation of LiDAR Data using Deep Neural Networks,” in Proc. of the IEEE/RSJ Intl. Conf. on Intelligent Robots and Systems (IROS), 2020.
[BibTeX] [PDF] [Code] [Video]@inproceedings{langer2020iros, author = {F. Langer and A. Milioto and A. Haag and J. Behley and C. Stachniss}, title = {{Domain Transfer for Semantic Segmentation of LiDAR Data using Deep Neural Networks}}, booktitle = iros, year = {2020}, url = {https://www.ipb.uni-bonn.de/pdfs/langer2020iros.pdf}, videourl = {https://youtu.be/6FNGF4hKBD0}, codeurl = {https://github.com/PRBonn/lidar_transfer} }
- X. Chen, T. Läbe, A. Milioto, T. Röhling, O. Vysotska, A. Haag, J. Behley, and C. Stachniss, “OverlapNet: Loop Closing for LiDAR-based SLAM,” in Proc. of Robotics: Science and Systems (RSS), 2020.
[BibTeX] [PDF] [Code] [Video]@inproceedings{chen2020rss, author = {X. Chen and T. L\"abe and A. Milioto and T. R\"ohling and O. Vysotska and A. Haag and J. Behley and C. Stachniss}, title = {{OverlapNet: Loop Closing for LiDAR-based SLAM}}, booktitle = rss, year = {2020}, codeurl = {https://github.com/PRBonn/OverlapNet/}, videourl = {https://youtu.be/YTfliBco6aw} }
- J. Behley, A. Milioto, and C. Stachniss, “A Benchmark for LiDAR-based Panoptic Segmentation based on KITTI,” arXiv Preprint, 2020.
[BibTeX] [PDF]Panoptic segmentation is the recently introduced task that tackles semantic segmentation and instance segmentation jointly. In this paper, we present an extension of SemanticKITTI, which is a large-scale dataset providing dense point-wise semantic labels for all sequences of the KITTI Odometry Benchmark, for training and evaluation of laser-based panoptic segmentation. We provide the data and discuss the processing steps needed to enrich a given semantic annotation with temporally consistent instance information, i.e., instance information that supplements the semantic labels and identifies the same instance over sequences of LiDAR point clouds. Additionally, we present two strong baselines that combine state-of-the-art LiDAR-based semantic segmentation approaches with a state-of-the-art detector enriching the segmentation with instance information and that allow other researchers to compare their approaches against. We hope that our extension of SemanticKITTI with strong baselines enables the creation of novel algorithms for LiDAR-based panoptic segmentation as much as it has for the original semantic segmentation and semantic scene completion tasks. Data, code, and an online evaluation using a hidden test set will be published on https://semantic-kitti.org.
@article{behley2020arxiv, author = {J. Behley and A. Milioto and C. Stachniss}, title = {{A Benchmark for LiDAR-based Panoptic Segmentation based on KITTI}}, journal = arxiv, year = 2020, eprint = {2003.02371v1}, url = {https://arxiv.org/pdf/2003.02371v1}, keywords = {cs.CV}, abstract = {Panoptic segmentation is the recently introduced task that tackles semantic segmentation and instance segmentation jointly. In this paper, we present an extension of SemanticKITTI, which is a large-scale dataset providing dense point-wise semantic labels for all sequences of the KITTI Odometry Benchmark, for training and evaluation of laser-based panoptic segmentation. We provide the data and discuss the processing steps needed to enrich a given semantic annotation with temporally consistent instance information, i.e., instance information that supplements the semantic labels and identifies the same instance over sequences of LiDAR point clouds. Additionally, we present two strong baselines that combine state-of-the-art LiDAR-based semantic segmentation approaches with a state-of-the-art detector enriching the segmentation with instance information and that allow other researchers to compare their approaches against. We hope that our extension of SemanticKITTI with strong baselines enables the creation of novel algorithms for LiDAR-based panoptic segmentation as much as it has for the original semantic segmentation and semantic scene completion tasks. Data, code, and an online evaluation using a hidden test set will be published on https://semantic-kitti.org.} }
- P. Lottes, J. Behley, N. Chebrolu, A. Milioto, and C. Stachniss, “Robust joint stem detection and crop-weed classification using image sequences for plant-specific treatment in precision farming,” Journal of Field Robotics (JFR), vol. 37, pp. 20-34, 2020. doi:10.1002/rob.21901
[BibTeX] [PDF]@article{lottes2020jfr, title = {Robust joint stem detection and crop-weed classification using image sequences for plant-specific treatment in precision farming}, author = {Lottes, P. and Behley, J. and Chebrolu, N. and Milioto, A. and Stachniss, C.}, journal = jfr, volume = {37}, numer = {1}, pages = {20-34}, year = {2020}, doi = {10.1002/rob.21901}, url = {https://www.ipb.uni-bonn.de/pdfs/lottes2019jfr.pdf} }
- R. Sheikh, A. Milioto, P. Lottes, C. Stachniss, M. Bennewitz, and T. Schultz, “Gradient and Log-based Active Learning for Semantic Segmentation of Crop and Weed for Agricultural Robots,” in Proc. of the IEEE Intl. Conf. on Robotics & Automation (ICRA), 2020.
[BibTeX] [PDF] [Video]@inproceedings{sheikh2020icra, title = {Gradient and Log-based Active Learning for Semantic Segmentation of Crop and Weed for Agricultural Robots}, author = {R. Sheikh and A. Milioto and P. Lottes and C. Stachniss and M. Bennewitz and T. Schultz}, booktitle = icra, year = {2020}, url = {https://www.ipb.uni-bonn.de/pdfs/sheikh2020icra.pdf}, videourl = {https://www.youtube.com/watch?v=NySa59gxFAg} }
- P. Regier, A. Milioto, C. Stachniss, and M. Bennewitz, “Classifying Obstacles and Exploiting Class Information for Humanoid Navigation Through Cluttered Environments,” The Intl. Journal of Humanoid Robotics (IJHR), vol. 17, iss. 02, p. 2050013, 2020. doi:10.1142/S0219843620500139
[BibTeX] [PDF]Humanoid robots are often supposed to share their workspace with humans and thus have to deal with objects used by humans in their everyday life. In this article, we present our novel approach to humanoid navigation through cluttered environments, which exploits knowledge about different obstacle classes to decide how to deal with obstacles and select appropriate robot actions. To classify objects from RGB images and decide whether an obstacle can be overcome by the robot with a corresponding action, e.g., by pushing or carrying it aside or stepping over or onto it, we train and exploit a convolutional neural network (CNN). Based on associated action costs, we compute a cost grid containing newly observed objects in addition to static obstacles on which a 2D path can be efficiently planned. This path encodes the necessary actions that need to be carried out by the robot to reach the goal. We implemented our framework in the Robot Operating System (ROS) and tested it in various scenarios with a Nao robot as well as in simulation with the REEM-C robot. As the experiments demonstrate, using our CNN, the robot can robustly classify the observed obstacles into the different classes and decide on suitable actions to find efficient solution paths. Our system finds paths also through regions where traditional motion planning methods are not able to calculate a solution or require substantially more time.
@article{regier2020ijhr, author = {Regier, P. and Milioto, A. and Stachniss, C. and Bennewitz, M.}, title = {{Classifying Obstacles and Exploiting Class Information for Humanoid Navigation Through Cluttered Environments}}, journal = ijhr, volume = {17}, number = {02}, pages = {2050013}, year = {2020}, doi = {10.1142/S0219843620500139}, abstract = {Humanoid robots are often supposed to share their workspace with humans and thus have to deal with objects used by humans in their everyday life. In this article, we present our novel approach to humanoid navigation through cluttered environments, which exploits knowledge about different obstacle classes to decide how to deal with obstacles and select appropriate robot actions. To classify objects from RGB images and decide whether an obstacle can be overcome by the robot with a corresponding action, e.g., by pushing or carrying it aside or stepping over or onto it, we train and exploit a convolutional neural network (CNN). Based on associated action costs, we compute a cost grid containing newly observed objects in addition to static obstacles on which a 2D path can be efficiently planned. This path encodes the necessary actions that need to be carried out by the robot to reach the goal. We implemented our framework in the Robot Operating System (ROS) and tested it in various scenarios with a Nao robot as well as in simulation with the REEM-C robot. As the experiments demonstrate, using our CNN, the robot can robustly classify the observed obstacles into the different classes and decide on suitable actions to find efficient solution paths. Our system finds paths also through regions where traditional motion planning methods are not able to calculate a solution or require substantially more time. } }
2019
- J. Behley, M. Garbade, A. Milioto, J. Quenzel, S. Behnke, C. Stachniss, and J. Gall, “SemanticKITTI: A Dataset for Semantic Scene Understanding of LiDAR Sequences,” in Proc. of the IEEE/CVF Intl. Conf. on Computer Vision (ICCV), 2019.
[BibTeX] [PDF] [Video]@inproceedings{behley2019iccv, author = {J. Behley and M. Garbade and A. Milioto and J. Quenzel and S. Behnke and C. Stachniss and J. Gall}, title = {{SemanticKITTI: A Dataset for Semantic Scene Understanding of LiDAR Sequences}}, booktitle = iccv, year = {2019}, videourl = {https://www.ipb.uni-bonn.de/html/projects/semantic_kitti/videos/teaser.mp4} }
- A. Pretto, S. Aravecchia, W. Burgard, N. Chebrolu, C. Dornhege, T. Falck, F. Fleckenstein, A. Fontenla, M. Imperoli, R. Khanna, F. Liebisch, P. Lottes, A. Milioto, D. Nardi, S. Nardi, J. Pfeifer, M. Popović, C. Potena, C. Pradalier, E. Rothacker-Feder, I. Sa, A. Schaefer, R. Siegwart, C. Stachniss, A. Walter, W. Winterhalter, X. Wu, and J. Nieto, “Building an Aerial-Ground Robotics System for Precision Farming,” arXiv Preprint, 2019.
[BibTeX] [PDF]@article{pretto2019arxiv, author = {A. Pretto and S. Aravecchia and W. Burgard and N. Chebrolu and C. Dornhege and T. Falck and F. Fleckenstein and A. Fontenla and M. Imperoli and R. Khanna and F. Liebisch and P. Lottes and A. Milioto and D. Nardi and S. Nardi and J. Pfeifer and M. Popović and C. Potena and C. Pradalier and E. Rothacker-Feder and I. Sa and A. Schaefer and R. Siegwart and C. Stachniss and A. Walter and W. Winterhalter and X. Wu and J. Nieto}, title = {{Building an Aerial-Ground Robotics System for Precision Farming}}, journal = arxiv, year = 2019, eprint = {1911.03098v1}, url = {https://arxiv.org/pdf/1911.03098v1}, keywords = {cs.RO} }
- X. Chen, A. Milioto, E. Palazzolo, P. Giguère, J. Behley, and C. Stachniss, “SuMa++: Efficient LiDAR-based Semantic SLAM,” in Proc. of the IEEE/RSJ Intl. Conf. on Intelligent Robots and Systems (IROS), 2019.
[BibTeX] [PDF] [Code] [Video]@inproceedings{chen2019iros, author = {X. Chen and A. Milioto and E. Palazzolo and P. Giguère and J. Behley and C. Stachniss}, title = {{SuMa++: Efficient LiDAR-based Semantic SLAM}}, booktitle = iros, year = 2019, codeurl = {https://github.com/PRBonn/semantic_suma/}, videourl = {https://youtu.be/uo3ZuLuFAzk} }
- A. Milioto, I. Vizzo, J. Behley, and C. Stachniss, “RangeNet++: Fast and Accurate LiDAR Semantic Segmentation,” in Proc. of the IEEE/RSJ Intl. Conf. on Intelligent Robots and Systems (IROS), 2019.
[BibTeX] [PDF] [Code] [Video]@inproceedings{milioto2019iros, author = {A. Milioto and I. Vizzo and J. Behley and C. Stachniss}, title = {{RangeNet++: Fast and Accurate LiDAR Semantic Segmentation}}, booktitle = iros, year = 2019, codeurl = {https://github.com/PRBonn/lidar-bonnetal}, videourl = {https://youtu.be/wuokg7MFZyU} }
- L. Zabawa, A. Kicherer, L. Klingbeil, A. Milioto, R. Topfer, H. Kuhlmann, and R. Roscher, “Detection of Single Grapevine Berries in Images Using Fully Convolutional Neural Networks,” in The IEEE Conf. on Computer Vision and Pattern Recognition (CVPR) Workshops, 2019.
[BibTeX] [PDF]@inproceedings{zabawa2019cvpr-workshop, author = {L. Zabawa and A. Kicherer and L. Klingbeil and A. Milioto and R. Topfer and H. Kuhlmann and R. Roscher}, title = {{Detection of Single Grapevine Berries in Images Using Fully Convolutional Neural Networks}}, booktitle = {The IEEE Conf. on Computer Vision and Pattern Recognition (CVPR) Workshops}, month = {June}, year = {2019} }
- A. Milioto and C. Stachniss, “Bonnet: An Open-Source Training and Deployment Framework for Semantic Segmentation in Robotics using CNNs,” in Proc. of the IEEE Intl. Conf. on Robotics & Automation (ICRA), 2019.
[BibTeX] [PDF] [Code] [Video]@inproceedings{milioto2019icra, author = {A. Milioto and C. Stachniss}, title = {{Bonnet: An Open-Source Training and Deployment Framework for Semantic Segmentation in Robotics using CNNs}}, booktitle = icra, year = 2019, codeurl = {https://github.com/Photogrammetry-Robotics-Bonn/bonnet}, videourl = {https://www.youtube.com/watch?v=tfeFHCq6YJs} }
- A. Milioto, L. Mandtler, and C. Stachniss, “Fast Instance and Semantic Segmentation Exploiting Local Connectivity, Metric Learning, and One-Shot Detection for Robotics ,” in Proc. of the IEEE Intl. Conf. on Robotics & Automation (ICRA), 2019.
[BibTeX] [PDF]@inproceedings{milioto2019icra-fiass, author = {A. Milioto and L. Mandtler and C. Stachniss}, title = {{Fast Instance and Semantic Segmentation Exploiting Local Connectivity, Metric Learning, and One-Shot Detection for Robotics }}, booktitle = icra, year = 2019 }
2018
- P. Lottes, J. Behley, A. Milioto, and C. Stachniss, “Fully Convolutional Networks with Sequential Information for Robust Crop and Weed Detection in Precision Farming,” IEEE Robotics and Automation Letters (RA-L), vol. 3, pp. 3097-3104, 2018. doi:10.1109/LRA.2018.2846289
[BibTeX] [PDF] [Video]@article{lottes2018ral, author = {P. Lottes and J. Behley and A. Milioto and C. Stachniss}, title = {Fully Convolutional Networks with Sequential Information for Robust Crop and Weed Detection in Precision Farming}, journal = ral, year = {2018}, volume = {3}, issue = {4}, pages = {3097-3104}, doi = {10.1109/LRA.2018.2846289}, url = {https://www.ipb.uni-bonn.de/pdfs/lottes2018ral.pdf}, videourl = {https://www.youtube.com/watch?v=vTepw9HRLh8} }
- P. Regier, A. Milioto, P. Karkowski, C. Stachniss, and M. Bennewitz, “Classifying Obstacles and Exploiting Knowledge about Classes for Efficient Humanoid Navigation,” in Proc. of the IEEE-RAS Int. Conf. on Humanoid Robots (HUMANOIDS), 2018.
[BibTeX] [PDF]@inproceedings{regier2018humanoids, author = {P. Regier and A. Milioto and P. Karkowski and C. Stachniss and M. Bennewitz}, title = {{Classifying Obstacles and Exploiting Knowledge about Classes for Efficient Humanoid Navigation}}, booktitle = {Proc. of the IEEE-RAS Int. Conf. on Humanoid Robots (HUMANOIDS)}, year = 2018 }
- P. Lottes, J. Behley, N. Chebrolu, A. Milioto, and C. Stachniss, “Joint Stem Detection and Crop-Weed Classification for Plant-specific Treatment in Precision Farming,” in Proc. of the IEEE/RSJ Intl. Conf. on Intelligent Robots and Systems (IROS), 2018.
[BibTeX] [PDF] [Video]Applying agrochemicals is the default procedure for conventional weed control in crop production, but has negative impacts on the environment. Robots have the potential to treat every plant in the field individually and thus can reduce the required use of such chemicals. To achieve that, robots need the ability to identify crops and weeds in the field and must additionally select effective treatments. While certain types of weed can be treated mechanically, other types need to be treated by (selective) spraying. In this paper, we present an approach that provides the necessary information for effective plant-specific treatment. It outputs the stem location for weeds, which allows for mechanical treatments, and the covered area of the weed for selective spraying. Our approach uses an end-to- end trainable fully convolutional network that simultaneously estimates stem positions as well as the covered area of crops and weeds. It jointly learns the class-wise stem detection and the pixel-wise semantic segmentation. Experimental evaluations on different real-world datasets show that our approach is able to reliably solve this problem. Compared to state-of-the-art approaches, our approach not only substantially improves the stem detection accuracy, i.e., distinguishing crop and weed stems, but also provides an improvement in the semantic segmentation performance.
@inproceedings{lottes2018iros, author = {P. Lottes and J. Behley and N. Chebrolu and A. Milioto and C. Stachniss}, title = {Joint Stem Detection and Crop-Weed Classification for Plant-specific Treatment in Precision Farming}, booktitle = iros, year = 2018, url = {https://www.ipb.uni-bonn.de/pdfs/lottes18iros.pdf}, videourl = {https://www.youtube.com/watch?v=C9mjZxE_Sxg}, abstract = {Applying agrochemicals is the default procedure for conventional weed control in crop production, but has negative impacts on the environment. Robots have the potential to treat every plant in the field individually and thus can reduce the required use of such chemicals. To achieve that, robots need the ability to identify crops and weeds in the field and must additionally select effective treatments. While certain types of weed can be treated mechanically, other types need to be treated by (selective) spraying. In this paper, we present an approach that provides the necessary information for effective plant-specific treatment. It outputs the stem location for weeds, which allows for mechanical treatments, and the covered area of the weed for selective spraying. Our approach uses an end-to- end trainable fully convolutional network that simultaneously estimates stem positions as well as the covered area of crops and weeds. It jointly learns the class-wise stem detection and the pixel-wise semantic segmentation. Experimental evaluations on different real-world datasets show that our approach is able to reliably solve this problem. Compared to state-of-the-art approaches, our approach not only substantially improves the stem detection accuracy, i.e., distinguishing crop and weed stems, but also provides an improvement in the semantic segmentation performance.} }
- A. Milioto, P. Lottes, and C. Stachniss, “Real-time Semantic Segmentation of Crop and Weed for Precision Agriculture Robots Leveraging Background Knowledge in CNNs,” in Proc. of the IEEE Intl. Conf. on Robotics & Automation (ICRA), 2018.
[BibTeX] [PDF] [Video]Precision farming robots, which target to reduce the amount of herbicides that need to be brought out in the fields, must have the ability to identify crops and weeds in real time to trigger weeding actions. In this paper, we address the problem of CNN-based semantic segmentation of crop fields separating sugar beet plants, weeds, and background solely based on RGB data. We propose a CNN that exploits existing vegetation indexes and provides a classification in real time. Furthermore, it can be effectively re-trained to so far unseen fields with a comparably small amount of training data. We implemented and thoroughly evaluated our system on a real agricultural robot operating in different fields in Germany and Switzerland. The results show that our system generalizes well, can operate at around 20Hz, and is suitable for online operation in the fields.
@inproceedings{milioto2018icra, author = {A. Milioto and P. Lottes and C. Stachniss}, title = {Real-time Semantic Segmentation of Crop and Weed for Precision Agriculture Robots Leveraging Background Knowledge in CNNs}, year = {2018}, booktitle = icra, abstract = {Precision farming robots, which target to reduce the amount of herbicides that need to be brought out in the fields, must have the ability to identify crops and weeds in real time to trigger weeding actions. In this paper, we address the problem of CNN-based semantic segmentation of crop fields separating sugar beet plants, weeds, and background solely based on RGB data. We propose a CNN that exploits existing vegetation indexes and provides a classification in real time. Furthermore, it can be effectively re-trained to so far unseen fields with a comparably small amount of training data. We implemented and thoroughly evaluated our system on a real agricultural robot operating in different fields in Germany and Switzerland. The results show that our system generalizes well, can operate at around 20Hz, and is suitable for online operation in the fields.}, url = {https://arxiv.org/abs/1709.06764}, videourl = {https://youtu.be/DXcTkJmdWFQ} }
- A. Milioto and C. Stachniss, “Bonnet: An Open-Source Training and Deployment Framework for Semantic Segmentation in Robotics using CNNs,” ICRA Worshop on Perception, Inference, and Learning for Joint Semantic, Geometric, and Physical Understanding, 2018.
[BibTeX] [PDF] [Code] [Video]@article{milioto2018icraws, author = {A. Milioto and C. Stachniss}, title = {{Bonnet: An Open-Source Training and Deployment Framework for Semantic Segmentation in Robotics using CNNs}}, journal = {ICRA Worshop on Perception, Inference, and Learning for Joint Semantic, Geometric, and Physical Understanding}, eprint = {1802.08960}, primaryclass = {cs.RO}, keywords = {Computer Science - Robotics, Computer Science - Computer Vision and Pattern Recognition}, year = 2018, month = may, url = {https://arxiv.org/abs/1802.08960}, codeurl = {https://github.com/Photogrammetry-Robotics-Bonn/bonnet}, videourl = {https://www.youtube.com/watch?v=tfeFHCq6YJs} }
- F. Langer, L. Mandtler, A. Milioto, E. Palazzolo, and C. Stachniss, “Geometrical Stem Detection from Image Data for Precision Agriculture,” arXiv Preprint, 2018.
[BibTeX] [PDF]@article{langer2018arxiv, author = {F. Langer and L. Mandtler and A. Milioto and E. Palazzolo and C. Stachniss}, title = {{Geometrical Stem Detection from Image Data for Precision Agriculture}}, journal = arxiv, year = 2018, eprint = {1812.05415v1}, url = {https://arxiv.org/pdf/1812.05415v1}, keywords = {cs.RO} }
- K. Franz, R. Roscher, A. Milioto, S. Wenzel, and J. Kusche, “Ocean Eddy Identification and Tracking using Neural Networks,” in IEEE International Geoscience and Remote Sensing Symposium (IGARSS), 2018.
[BibTeX] [PDF]@inproceedings{franz2018ocean, author = {Franz, K. and Roscher, R. and Milioto, A. and Wenzel, S. and Kusche, J.}, title = {Ocean Eddy Identification and Tracking using Neural Networks}, booktitle = {IEEE International Geoscience and Remote Sensing Symposium (IGARSS)}, year = {2018}, note = {accepted}, url = {https://arxiv.org/abs/arXiv:1803.07436} }
2017
- A. Milioto, P. Lottes, and C. Stachniss, “Real-time Blob-wise Sugar Beets vs Weeds Classification for Monitoring Fields using Convolutional Neural Networks,” in Proc. of the Intl. Conf. on Unmanned Aerial Vehicles in Geomatics, 2017.
[BibTeX] [PDF]UAVs are becoming an important tool for field monitoring and precision farming. A prerequisite for observing and analyzing fields is the ability to identify crops and weeds from image data. In this paper, we address the problem of detecting the sugar beet plants and weeds in the field based solely on image data. We propose a system that combines vegetation detection and deep learning to obtain a high-quality classification of the vegetation in the field into value crops and weeds. We implemented and thoroughly evaluated our system on image data collected from different sugar beet fields and illustrate that our approach allows for accurately identifying the weeds on the field.
@inproceedings{milioto2017uavg, title = {Real-time Blob-wise Sugar Beets vs Weeds Classification for Monitoring Fields using Convolutional Neural Networks}, author = {A. Milioto and P. Lottes and C. Stachniss}, booktitle = uavg, year = {2017}, abstract = {UAVs are becoming an important tool for field monitoring and precision farming. A prerequisite for observing and analyzing fields is the ability to identify crops and weeds from image data. In this paper, we address the problem of detecting the sugar beet plants and weeds in the field based solely on image data. We propose a system that combines vegetation detection and deep learning to obtain a high-quality classification of the vegetation in the field into value crops and weeds. We implemented and thoroughly evaluated our system on image data collected from different sugar beet fields and illustrate that our approach allows for accurately identifying the weeds on the field.}, url = {https://www.ipb.uni-bonn.de/pdfs/milioto17uavg.pdf} }