Benedikt Mersch
PhD Student Contact:Email: mersch@nulligg.uni-bonn.de
Tel: +49 – 228 – 73 – 27 11
Office: Nussallee 15, 1. OG, room 1.012
Address:
University of Bonn
Photogrammetry, IGG
Nussallee 15
53115 Bonn
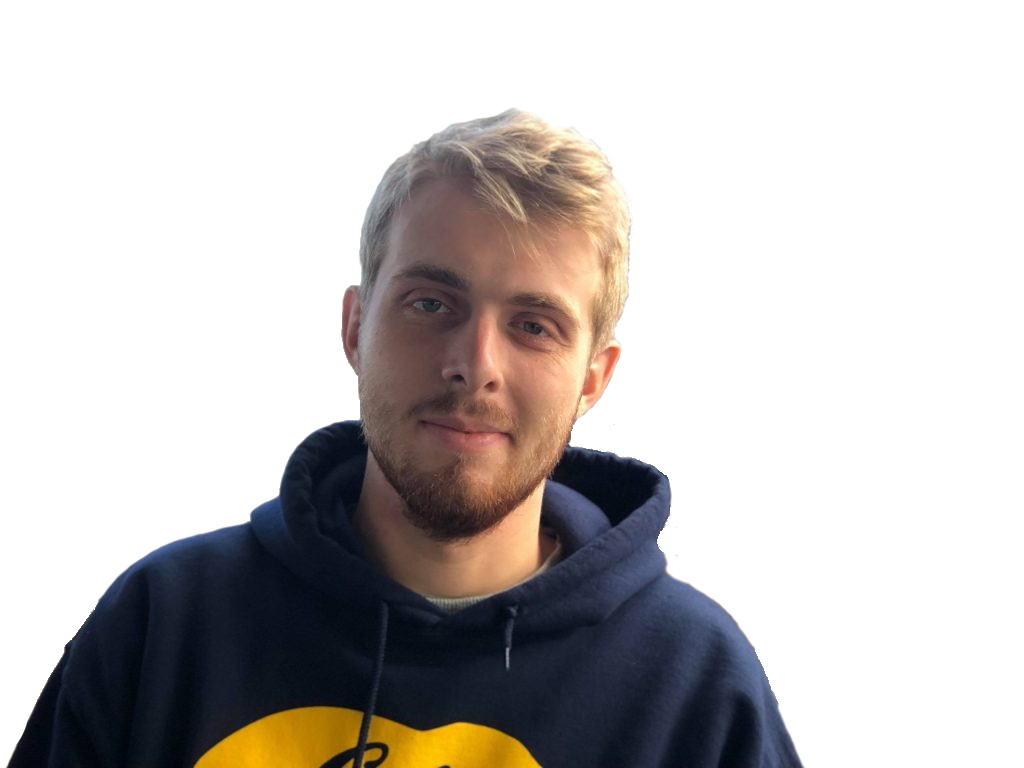
Profiles: Google Scholar | Github
Research Interests
- SLAM
- LiDAR Moving Object Segmentation
- Behavior Estimation
Short CV
Benedikt Mersch is a Ph.D. student at the Photogrammetry & Robotics Lab at the University of Bonn since July 2020. He received his master’s degree in Electrical Engineering at the Hamburg University of Technology in December 2019.
Teaching
- Techniques for Self-Driving Cars, 2024/2025
- Techniques for Self-Driving Cars, 2023/2024
- Techniques for Self-Driving Cars, 2022/2023
- Techniques for Self-Driving Cars, 2021/2022
- Mobile Sensing and Robotics Project, 2020/2021
- Techniques for Self-Driving Cars, 2020/2021
Code releases
- KISS-SLAM: A LiDAR SLAM pipeline that just works
StarForkWatch - Kinematic-ICP: A LiDAR odometry pipeline for wheeled mobile robots
StarForkWatch - MapMOS: Map-based LiDAR moving object segmentation
StarForkWatch - KISS-ICP: A LiDAR odometry pipeline that just works
StarForkWatch - 4DMOS: A sparse CNN on 4D point clouds for LiDAR moving object segmentation
StarForkWatch - point-cloud-prediction: Self-supervised point cloud prediction
StarForkWatch - LiDAR-MOS – Moving object segmentation in 3D LiDAR data
StarForkWatch
Publications
2025
- T. Guadagnino, B. Mersch, S. Gupta, I. Vizzo, G. Grisetti, and C. Stachniss, “KISS-SLAM: A Simple, Robust, and Accurate 3D LiDAR SLAM System With Enhanced Generalization Capabilities,” in Proc. of the IEEE/RSJ Intl. Conf. on Intelligent Robots and Systems (IROS), 2025.
[BibTeX] [PDF] [Code]@inproceedings{guadagnino2025iros, author = {T. Guadagnino and B. Mersch and S. Gupta and I. Vizzo and G. Grisetti and C. Stachniss}, title = {{KISS-SLAM: A Simple, Robust, and Accurate 3D LiDAR SLAM System With Enhanced Generalization Capabilities}}, booktitle = iros, year = 2025, url = {https://arxiv.org/pdf/2503.12660}, codeurl = {https://github.com/PRBonn/kiss-slam}, }
- B. Mersch, “Spatio-Temporal Perception for Mobile Robots in Dynamic Environments,” PhD Thesis, 2025.
[BibTeX] [PDF]@phdthesis{mersch2025phd, author = {Benedikt Mersch}, title = {{Spatio-Temporal Perception for Mobile Robots in Dynamic Environments}}, school = {University of Bonn}, year = 2025, url = {https://hdl.handle.net/20.500.11811/13012}, }
- T. Guadagnino, B. Mersch, I. Vizzo, S. Gupta, M. V. R. Malladi, L. Lobefaro, G. Doisy, and C. Stachniss, “Kinematic-ICP: Enhancing LiDAR Odometry with Kinematic Constraints for Wheeled Mobile Robots Moving on Planar Surfaces,” in Proc. of the IEEE Intl. Conf. on Robotics & Automation (ICRA), 2025.
[BibTeX] [PDF] [Code] [Video]@inproceedings{guadagnino2025icra, author = {Guadagnino, T. and Mersch, B. and Vizzo, I. and Gupta, S. and Malladi, M.V.R. and Lobefaro, L. and Doisy, G. and Stachniss, C.}, title = {{Kinematic-ICP: Enhancing LiDAR Odometry with Kinematic Constraints for Wheeled Mobile Robots Moving on Planar Surfaces}}, booktitle = icra, year = 2025, url = {https://www.ipb.uni-bonn.de/wp-content/papercite-data/pdf/guadagnino2025icra.pdf}, codeurl = {https://github.com/PRBonn/kinematic-icp}, videourl = {https://youtu.be/4nD5UtkMb1s} }
2024
- H. Lim, S. Jang, B. Mersch, J. Behley, H. Myung, and C. Stachniss, “HeLiMOS: A Dataset for Moving Object Segmentation in 3D Point Clouds From Heterogeneous LiDAR Sensors,” in Proc. of the IEEE/RSJ Intl. Conf. on Intelligent Robots and Systems (IROS), 2024. doi:10.1109/IROS58592.2024.10801938
[BibTeX] [PDF]@inproceedings{lim2024iros, author = {H. Lim and S. Jang and B. Mersch and J. Behley and H. Myung and C. Stachniss}, title = {{HeLiMOS: A Dataset for Moving Object Segmentation in 3D Point Clouds From Heterogeneous LiDAR Sensors}}, booktitle = iros, year = 2024, doi = {10.1109/IROS58592.2024.10801938} }
- L. Nunes, R. Marcuzzi, B. Mersch, J. Behley, and C. Stachniss, “Scaling Diffusion Models to Real-World 3D LiDAR Scene Completion,” in Proc. of the IEEE/CVF Conf. on Computer Vision and Pattern Recognition (CVPR), 2024. doi:10.1109/CVPR52733.2024.01399
[BibTeX] [PDF] [Code] [Video]@inproceedings{nunes2024cvpr, author = {L. Nunes and R. Marcuzzi and B. Mersch and J. Behley and C. Stachniss}, title = {{Scaling Diffusion Models to Real-World 3D LiDAR Scene Completion}}, booktitle = cvpr, year = 2024, doi = {10.1109/CVPR52733.2024.01399}, codeurl = {https://github.com/PRBonn/LiDiff}, videourl = {https://youtu.be/XWu8svlMKUo} }
- I. Hroob, B. Mersch, C. Stachniss, and M. Hanheide, “Generalizable Stable Points Segmentation for 3D LiDAR Scan-to-Map Long-Term Localization,” IEEE Robotics and Automation Letters (RA-L), vol. 9, iss. 4, p. 3546–3553, 2024. doi:10.1109/LRA.2024.3368236
[BibTeX] [PDF] [Code] [Video]@article{hroob2024ral, author = {I. Hroob and B. Mersch and C. Stachniss and M. Hanheide}, title = {{Generalizable Stable Points Segmentation for 3D LiDAR Scan-to-Map Long-Term Localization}}, journal = ral, volume = {9}, number = {4}, pages = {3546--3553}, year = 2024, doi = {10.1109/LRA.2024.3368236}, videourl = {https://youtu.be/aRLStFQEXbc}, codeurl = {https://github.com/ibrahimhroob/SPS} }
- S. Gupta, T. Guadagnino, B. Mersch, I. Vizzo, and C. Stachniss, “Effectively Detecting Loop Closures using Point Cloud Density Maps,” in Proc. of the IEEE Intl. Conf. on Robotics & Automation (ICRA), 2024. doi:10.1109/ICRA57147.2024.10610962
[BibTeX] [PDF] [Code] [Video]@inproceedings{gupta2024icra, author = {S. Gupta and T. Guadagnino and B. Mersch and I. Vizzo and C. Stachniss}, title = {{Effectively Detecting Loop Closures using Point Cloud Density Maps}}, booktitle = icra, year = 2024, doi = {10.1109/ICRA57147.2024.10610962}, codeurl = {https://github.com/PRBonn/MapClosures}, videourl = {https://youtu.be/BpwR_aLXrNo} }
- M. Zeller, V. S. Sandhu, B. Mersch, J. Behley, M. Heidingsfeld, and C. Stachniss, “Radar Instance Transformer: Reliable Moving Instance Segmentation in Sparse Radar Point Clouds,” IEEE Trans. on Robotics (TRO), vol. 40, pp. 2357-2372, 2024. doi:10.1109/TRO.2023.3338972
[BibTeX] [PDF] [Video]@article{zeller2024tro, author = {M. Zeller and Sandhu, V.S. and B. Mersch and J. Behley and M. Heidingsfeld and C. Stachniss}, title = {{Radar Instance Transformer: Reliable Moving Instance Segmentation in Sparse Radar Point Clouds}}, journal = tro, year = {2024}, volume = {40}, doi = {10.1109/TRO.2023.3338972}, pages = {2357-2372}, videourl = {https://www.youtube.com/watch?v=v-iXbJEcqPM} }
2023
- I. Vizzo, B. Mersch, L. Nunes, L. Wiesmann, T. Guadagnino, and C. Stachniss, “Toward Reproducible Version-Controlled Perception Platforms: Embracing Simplicity in Autonomous Vehicle Dataset Acquisition,” in Proc. of the Intl. Conf. on Intelligent Transportation Systems Workshops, 2023.
[BibTeX] [PDF] [Code]@inproceedings{vizzo2023itcsws, author = {I. Vizzo and B. Mersch and L. Nunes and L. Wiesmann and T. Guadagnino and C. Stachniss}, title = {{Toward Reproducible Version-Controlled Perception Platforms: Embracing Simplicity in Autonomous Vehicle Dataset Acquisition}}, booktitle = {Proc. of the Intl. Conf. on Intelligent Transportation Systems Workshops}, year = 2023, codeurl = {https://github.com/ipb-car/meta-workspace}, note = {accepted} }
- B. Mersch, T. Guadagnino, X. Chen, I. Vizzo, J. Behley, and C. Stachniss, “Building Volumetric Beliefs for Dynamic Environments Exploiting Map-Based Moving Object Segmentation,” IEEE Robotics and Automation Letters (RA-L), vol. 8, iss. 8, pp. 5180-5187, 2023. doi:10.1109/LRA.2023.3292583
[BibTeX] [PDF] [Code] [Video]@article{mersch2023ral, author = {B. Mersch and T. Guadagnino and X. Chen and I. Vizzo and J. Behley and C. Stachniss}, title = {{Building Volumetric Beliefs for Dynamic Environments Exploiting Map-Based Moving Object Segmentation}}, journal = ral, volume = {8}, number = {8}, pages = {5180-5187}, year = 2023, issn = {2377-3766}, doi = {10.1109/LRA.2023.3292583}, videourl = {https://youtu.be/aeXhvkwtDbI}, codeurl = {https://github.com/PRBonn/MapMOS} }
- H. Lim, L. Nunes, B. Mersch, X. Chen, J. Behley, H. Myung, and C. Stachniss, “ERASOR2: Instance-Aware Robust 3D Mapping of the Static World in Dynamic Scenes,” in Proc. of Robotics: Science and Systems (RSS), 2023.
[BibTeX] [PDF]@inproceedings{lim2023rss, author = {H. Lim and L. Nunes and B. Mersch and X. Chen and J. Behley and H. Myung and C. Stachniss}, title = {{ERASOR2: Instance-Aware Robust 3D Mapping of the Static World in Dynamic Scenes}}, booktitle = rss, year = 2023 }
- M. Zeller, V. S. Sandhu, B. Mersch, J. Behley, M. Heidingsfeld, and C. Stachniss, “Radar Velocity Transformer: Single-scan Moving Object Segmentation in Noisy Radar Point Clouds,” in Proc. of the IEEE Intl. Conf. on Robotics & Automation (ICRA), 2023.
[BibTeX] [PDF] [Video]@inproceedings{zeller2023icra, author = {M. Zeller and V.S. Sandhu and B. Mersch and J. Behley and M. Heidingsfeld and C. Stachniss}, title = {{Radar Velocity Transformer: Single-scan Moving Object Segmentation in Noisy Radar Point Clouds}}, booktitle = icra, year = 2023, videourl = {https://youtu.be/dTDgzWIBgpE} }
- I. Vizzo, T. Guadagnino, B. Mersch, L. Wiesmann, J. Behley, and C. Stachniss, “KISS-ICP: In Defense of Point-to-Point ICP – Simple, Accurate, and Robust Registration If Done the Right Way,” IEEE Robotics and Automation Letters (RA-L), vol. 8, iss. 2, pp. 1-8, 2023. doi:10.1109/LRA.2023.3236571
[BibTeX] [PDF] [Code] [Video]@article{vizzo2023ral, author = {Vizzo, Ignacio and Guadagnino, Tiziano and Mersch, Benedikt and Wiesmann, Louis and Behley, Jens and Stachniss, Cyrill}, title = {{KISS-ICP: In Defense of Point-to-Point ICP -- Simple, Accurate, and Robust Registration If Done the Right Way}}, journal = ral, pages = {1-8}, doi = {10.1109/LRA.2023.3236571}, volume = {8}, number = {2}, year = {2023}, codeurl = {https://github.com/PRBonn/kiss-icp}, videourl = {https://youtu.be/h71aGiD-uxU} }
2022
- I. Vizzo, B. Mersch, R. Marcuzzi, L. Wiesmann, J. Behley, and C. Stachniss, “Make it Dense: Self-Supervised Geometric Scan Completion of Sparse 3D LiDAR Scans in Large Outdoor Environments,” IEEE Robotics and Automation Letters (RA-L), vol. 7, iss. 3, pp. 8534-8541, 2022. doi:10.1109/LRA.2022.3187255
[BibTeX] [PDF] [Code] [Video]@article{vizzo2022ral, author = {I. Vizzo and B. Mersch and R. Marcuzzi and L. Wiesmann and J. Behley and C. Stachniss}, title = {Make it Dense: Self-Supervised Geometric Scan Completion of Sparse 3D LiDAR Scans in Large Outdoor Environments}, journal = ral, url = {https://www.ipb.uni-bonn.de/wp-content/papercite-data/pdf/vizzo2022ral-iros.pdf}, codeurl = {https://github.com/PRBonn/make_it_dense}, year = {2022}, volume = {7}, number = {3}, pages = {8534-8541}, doi = {10.1109/LRA.2022.3187255}, videourl = {https://youtu.be/NVjURcArHn8} }
- B. Mersch, X. Chen, I. Vizzo, L. Nunes, J. Behley, and C. Stachniss, “Receding Moving Object Segmentation in 3D LiDAR Data Using Sparse 4D Convolutions,” IEEE Robotics and Automation Letters (RA-L), vol. 7, iss. 3, p. 7503–7510, 2022. doi:10.1109/LRA.2022.3183245
[BibTeX] [PDF] [Code] [Video]@article{mersch2022ral, author = {B. Mersch and X. Chen and I. Vizzo and L. Nunes and J. Behley and C. Stachniss}, title = {{Receding Moving Object Segmentation in 3D LiDAR Data Using Sparse 4D Convolutions}}, journal = ral, year = 2022, url = {https://www.ipb.uni-bonn.de/wp-content/papercite-data/pdf/mersch2022ral.pdf}, volume = {7}, number = {3}, pages = {7503--7510}, doi = {10.1109/LRA.2022.3183245}, codeurl = {https://github.com/PRBonn/4DMOS}, videourl = {https://youtu.be/5aWew6caPNQ} }
- X. Chen, B. Mersch, L. Nunes, R. Marcuzzi, I. Vizzo, J. Behley, and C. Stachniss, “Automatic Labeling to Generate Training Data for Online LiDAR-Based Moving Object Segmentation,” IEEE Robotics and Automation Letters (RA-L), vol. 7, iss. 3, pp. 6107-6114, 2022. doi:10.1109/LRA.2022.3166544
[BibTeX] [PDF] [Code] [Video]@article{chen2022ral, author = {X. Chen and B. Mersch and L. Nunes and R. Marcuzzi and I. Vizzo and J. Behley and C. Stachniss}, title = {{Automatic Labeling to Generate Training Data for Online LiDAR-Based Moving Object Segmentation}}, journal = ral, year = 2022, volume = 7, number = 3, pages = {6107-6114}, url = {https://arxiv.org/pdf/2201.04501}, issn = {2377-3766}, doi = {10.1109/LRA.2022.3166544}, codeurl = {https://github.com/PRBonn/auto-mos}, videourl = {https://youtu.be/3V5RA1udL4c} }
2021
- B. Mersch, X. Chen, J. Behley, and C. Stachniss, “Self-supervised Point Cloud Prediction Using 3D Spatio-temporal Convolutional Networks,” in Proc. of the Conf. on Robot Learning (CoRL), 2021.
[BibTeX] [PDF] [Code] [Video]@inproceedings{mersch2021corl, author = {B. Mersch and X. Chen and J. Behley and C. Stachniss}, title = {{Self-supervised Point Cloud Prediction Using 3D Spatio-temporal Convolutional Networks}}, booktitle = corl, year = {2021}, url = {https://www.ipb.uni-bonn.de/pdfs/mersch2021corl.pdf}, codeurl = {https://github.com/PRBonn/point-cloud-prediction}, videourl = {https://youtu.be/-pSZpPgFAso} }
- B. Mersch, T. Höllen, K. Zhao, C. Stachniss, and R. Roscher, “Maneuver-based Trajectory Prediction for Self-driving Cars Using Spatio-temporal Convolutional Networks,” in Proc. of the IEEE/RSJ Intl. Conf. on Intelligent Robots and Systems (IROS), 2021.
[BibTeX] [PDF] [Video]@inproceedings{mersch2021iros, title = {{Maneuver-based Trajectory Prediction for Self-driving Cars Using Spatio-temporal Convolutional Networks}}, author = {B. Mersch and T. H\"ollen and K. Zhao and C. Stachniss and R. Roscher}, booktitle = iros, year = {2021}, videourl = {https://youtu.be/5RRGWUn4qAw}, url = {https://www.ipb.uni-bonn.de/pdfs/mersch2021iros.pdf} }
- X. Chen, S. Li, B. Mersch, L. Wiesmann, J. Gall, J. Behley, and C. Stachniss, “Moving Object Segmentation in 3D LiDAR Data: A Learning-based Approach Exploiting Sequential Data,” IEEE Robotics and Automation Letters (RA-L), vol. 6, pp. 6529-6536, 2021. doi:10.1109/LRA.2021.3093567
[BibTeX] [PDF] [Code] [Video]@article{chen2021ral, title = {{Moving Object Segmentation in 3D LiDAR Data: A Learning-based Approach Exploiting Sequential Data}}, author = {X. Chen and S. Li and B. Mersch and L. Wiesmann and J. Gall and J. Behley and C. Stachniss}, year = {2021}, volume = 6, issue = 4, pages = {6529-6536}, journal = ral, url = {https://www.ipb.uni-bonn.de/pdfs/chen2021ral-iros.pdf}, codeurl = {https://github.com/PRBonn/LiDAR-MOS}, videourl = {https://youtu.be/NHvsYhk4dhw}, doi = {10.1109/LRA.2021.3093567}, issn = {2377-3766} }