Our Cluster of Excellence proposal “PhenoRob – Robotics and Phenotyping for Sustainable Crop Production” has been accepted today.
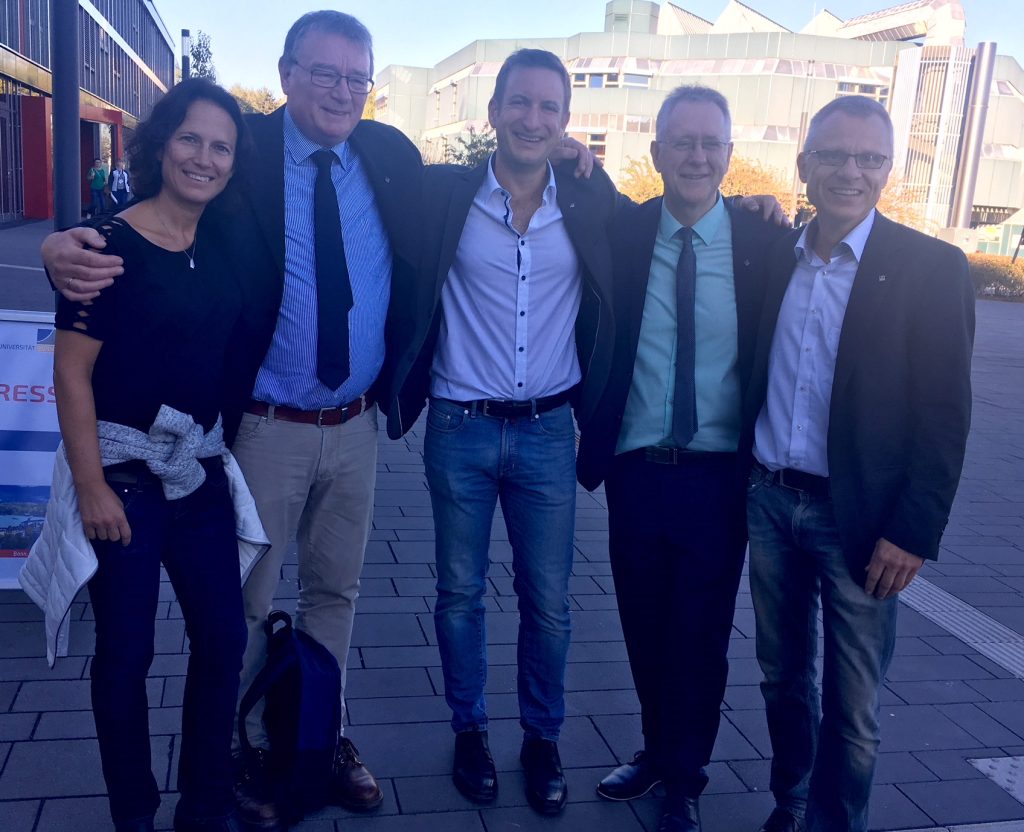
One of the greatest challenges for humanity is to produce sufficient food, feed, fiber, and fuel for an ever-growing world population while simultaneously reducing the environmental footprint of agricultural production. x arable land is limited, and the input of agro-chemicals needs to be reduced to curb environmental pollution and halt the decline in biodiversity. Climate change poses additional constraints on crop farming. Achieving sustainable crop production with limited resources is, thus, a task of immense proportions.
Our main hypothesis is that a major shift toward sustainable crop production can be achieved via two approaches: (1) multi-scale monitoring of plants and their environment using autonomous robots with automated and individualized intervention and big data analytics combined with machine learning to improve our understanding of the relation between input and output parameters of crop production, and (2) assessing, modeling, and optimizing the implications of the developed technical innovations in a systemic manner.
To realize our vision, we will take a technology-driven approach to address the challenging scientific objectives. We foresee novel ways of growing crops and managing fields, and aim at reducing the environmental footprint of crop production, maintaining the quality of soil and arable land, and analyzing the best routes to improve the adoption of technology.
The novel approach of PhenoRob is characterized by the integration of robotics, digitalization, and machine learning on one hand, and modern phenotyping, modeling, and crop production on the other. First, we will systematically monitor all essential aspects of crop production using sensor networks as well as ground and aerial robots. This is expected to provide detailed spatially and temporally aligned information at the level of individual plants, nutrient and disease status, soil information as well as ecosystem parameters, such as vegetation diversity. This will enable a more targeted management of inputs (genetic resources, crop protection, fertilization) for optimizing outputs (yield, growth, environmental impact). Second, we will develop novel technologies to enable real-time control of weeds and selective spraying and fertilization of individual plants in field stands. This will help reduce the environmental footprint by reducing chemical input. Third, machine learning applied to crop data will improve our understanding and modeling of plant growth and resource efficiencies and will further assist in the identification of correlations. Furthermore, we will develop integrated multi-scale models for the soil-crop-atmosphere system. These technologies and the gained knowledge will change crop production on all levels. Fourth, in addition to the impact on management decisions at the farm level, we will investigate the requirements for technology adoption as well as socioeconomic and environmental impact of the innovations resulting from upscaling.