
RIG – Robotics Institute Germany (BMBF)
The Robotics Institute Germany (RIG) is an initiative by the Federal Ministry of Education and Research (BMBF) aimed at connecting leading robotics labs across Germany to enhance their international visibility, attract talent, and accelerate progress in AI-powered robotics. RIG consists of 14 universities and research institutions that conduct internationally leading research in the field of AI-powered robotics, training future talents and transforming research into real-world impact. In addition, 20 further well-known universities and research networks support the initiative as associated partners.
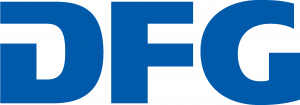
AID4Crops – Project 2: Exploiting Repeated Data Acquisitions for Improved Long-term Monitoring Capabilities (DFG, KI-FOR)
In the agricultural domain, fields as well as orchards are monitored repeatedly to assess the status quo and to trigger management decisions. To assess growth stages or compute phenotypic parameters of plants, knowledge about the plant geometry and further semantic information is key. Thus, estimating 3D geometric and semantic models of plants plays a key role in the automated status quo assessment. Most sensor-based monitoring systems, however, assume that the sensor platform is observing a new scene whenever starting the data acquisition process. Few approaches take prior maps from previous data into account to extend models or automatically track changes such as growth over time. Often, the fact that the same scene/objects are re-observed, potentially after undergoing some changes, is not exploited to its full extent. This project aims at addressing this challenge and will answer the following three coupled aspects: “How to build accurate plant models and exploit the fact that the same, but growing and changing objects, are being monitored repeatedly to (i) improve and achieve consistent modeling in the spatial and temporal dimensions (4D), (ii) estimate semantic information more precisely and consistently over time, and (iii) improve the involved learning approaches in a self-supervised or unsupervised way by exploiting prior knowledge about the scene?” This project will develop new approaches and will extend current systems for robot mapping/SLAM, filtering approaches for dealing with change, as well as contrastive learning in combination with deep neural networks to tackle the three research questions.
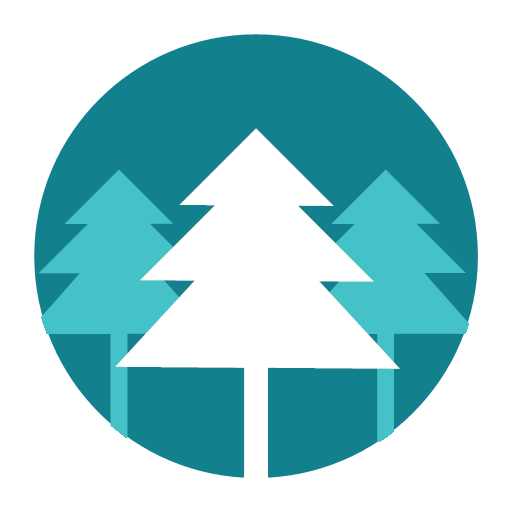
DigiForest – Digital Analytics and Robotics for Sustainable Forestry (EU, Horizon Europe)
What if we could create a revolution in spatial data acquisition, organization and analysis and give forestry operators and enterprises up-to-date, tangible information about the status of their forests down to the individual tree? We believe this would improve their oversight by allowing more accurate growth modelling of forest stands and precise predictions of timber yields. It would remove the uncertainty of when thinning operations are needed or where there are trees which are ready for harvest. It could also enable operators to automatically plan where their staff or equipment should be deployed. With capable (semi-)autonomous harvesting, operators eventually automating the full process.
It could also better quantify a forest’s carbon sequestration – with low uncertainty per-tree carbon estimates. Precise measures of crown volume and tree diameters would improve the granularity of carbon credit schemes. This could inform national governments and policy makers when deciding policy on initiatives such as carbon offsets and carbon farming.
In DIGIFOREST, we propose to create such an ecosystem by developing a team of heterogeneous robots to collect and update this raw 3D spatial representations, building large scale forest maps and feeding them to machine learning and spatial AI to semantically segment and label the trees and also the terrain. Our robot team will be diverse: we will use both rugged field robots as well as more experimental vehicles. Most ambitious of all is the intention to (semi-)automate a lightweight harvester for sustainable selective logging.
Progress in this project will be demonstrated with an ambitious series of field trials. With the clear engagement of forestry and industrial companies, commercial pathways are readily available.
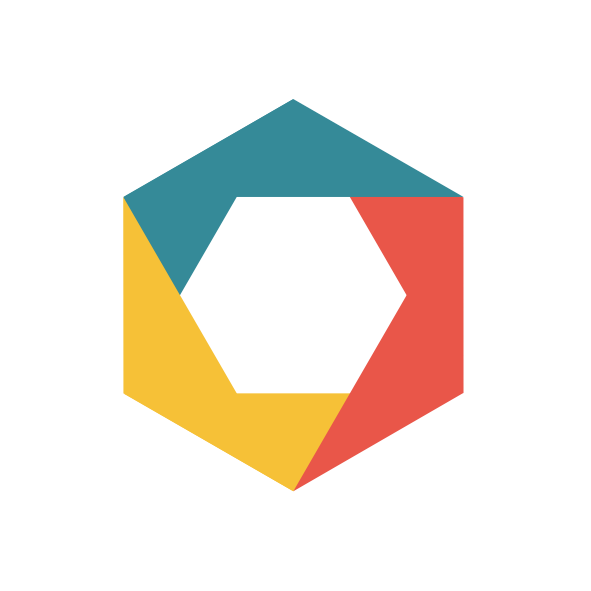
Harmony – Enhancing healthcare with assistive robotic mobile manipulation (EC, H2020)
Harmony will enable robust, flexible and safe autonomous mobile manipulation robots for use in human-centred environments by making fundamental contributions in cognitive mechatronic technologies. Our targeted application area is assistive healthcare robotics, which is motivated by the mounting pressure on our healthcare system due to factors such as Europe’s ageing population. While this presents an immense challenge for the healthcare industry, it is also an opportunity to proactively meet these challenges. Our current robotic automation solutions only offer “islands of automation” where either mobility or manipulation is dealt with in isolation. Harmony aims to fill this gap in knowledge on combining both robotic mobility and manipulation modalities in complex, human-centred environments. Harmony considers two use cases identified by our end user partners: 1) the automation of just-in-time delivery tasks; and 2) the automation of hospital bioassay sample flow. These use cases highlight existing processes that require fast, reliable and flexible automation to undertake the dull and repetitive tasks that are currently conducted by over-qualified staff. Critically, these tasks require robots that can interact with the world across a wide operational spectrum, from the (sub)millimetre precision required for fine manipulation to navigating across building- and campus-scale spaces. This motivates a holistic representation of the environment that facilitates the tight integration of socially aware planning, perception and control, and allows cognitive elements such as learning, reasoning and adaptation of actions for natural interaction. Harmony gathers the required expertise to tackle these core scientific and engineering challenges. Through demonstrators and open software modules, Harmony will show that robotic mobile manipulation systems can integrate seamlessly into our existing processes and spaces to meet growing needs in the healthcare industry and beyond.
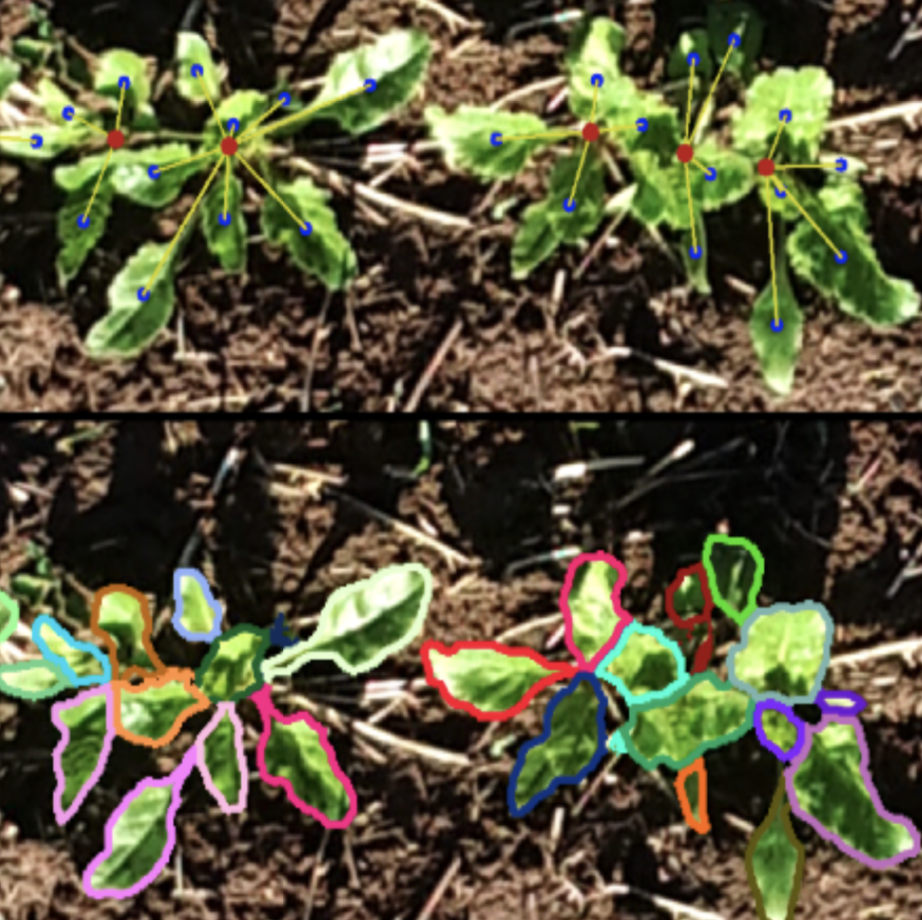
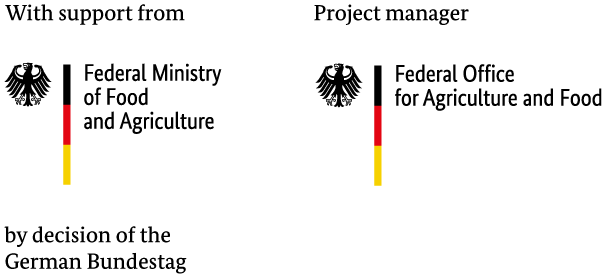
RegisTer – AI for Variety Description of Sugar Beets for the Federal Variety Approval (BLE)
RegisTer targets the use of artificial intelligence and optical sensors in the variety description of the Federal Plant Variety Office in the examination for distinctiveness, uniformity and stability and the value for cultivation within the scope of the variety approval for sugar beets.
Sugar beet is a common crop in Germany and represents an important economic factor for rural areas. Modern varieties must have diverse characteristics such as disease and stress tolerance and high yield potential. These characteristics must be recognized quickly and reliably in the breeding and approval process. Furthermore, each variety must be clearly described and be distinguishable. The goal of the interdisciplinary collaborative project RegisTer is to develop automated routines for the characterization and evaluation of sugar beet varieties based on the geometric and optical/reflective properties of the plants, which are measured using ultra-light flying drones. Drones, equipped with high- resolution RGB and multi-spectral cameras and 3D sensors are used to measure test fields. A millimeter- precise data set is the basis to automatically analyze the plant parameters with state-of-the-art image processing based on machine learning down to the individual plant level. The aim is to automatically extract (new) plant characteristics and their valuable properties for variety description and variety evaluation. The aim of this project is to achieve automation, standardization, and improvement of the assessment process for the register and value tests at the Federal Plant Variety Office and performance tests in the plant breeding industry, which is characterized by small and medium-sized enterprises.
The RegisTer project is technologically based on recording experimental plots and individual plants using drones and terrestrial laser scanning. The drone, equipped with high-resolution RGB and multi-spectral cameras, systematically records test plots at various locations within Germany and observes the plants with the required resolution in the millimeter range. These data form the basis for the investigation of the phenotype. In addition to the 3D data from drone images’ processing, we also collect high-precision 3D data utilizing terrestrial laser scanning, which we use to extract the leaf apparatus’s geometric parameters and for quality control of the 3D data from the drone images.
For the extraction of the register and value characteristics, we develop the most modern image processing with machine learning methods, which aim to recognize and analyze single plants, but at the same time also at the evaluation on the level of the plots. In addition to the automatic recognition of already existing traits, we also examine the data of new, previously unused factors for register inspection. For this purpose, we pursue the working hypothesis that the data contain variety-specific patterns suitable for distinctness and identification of sugar beet varieties. We link the data over different points in time and derive dynamic characteristics. We investigate these parameters to develop new traits for variety description and use the temporal correlation in the data to improve the self-learning algorithms further.
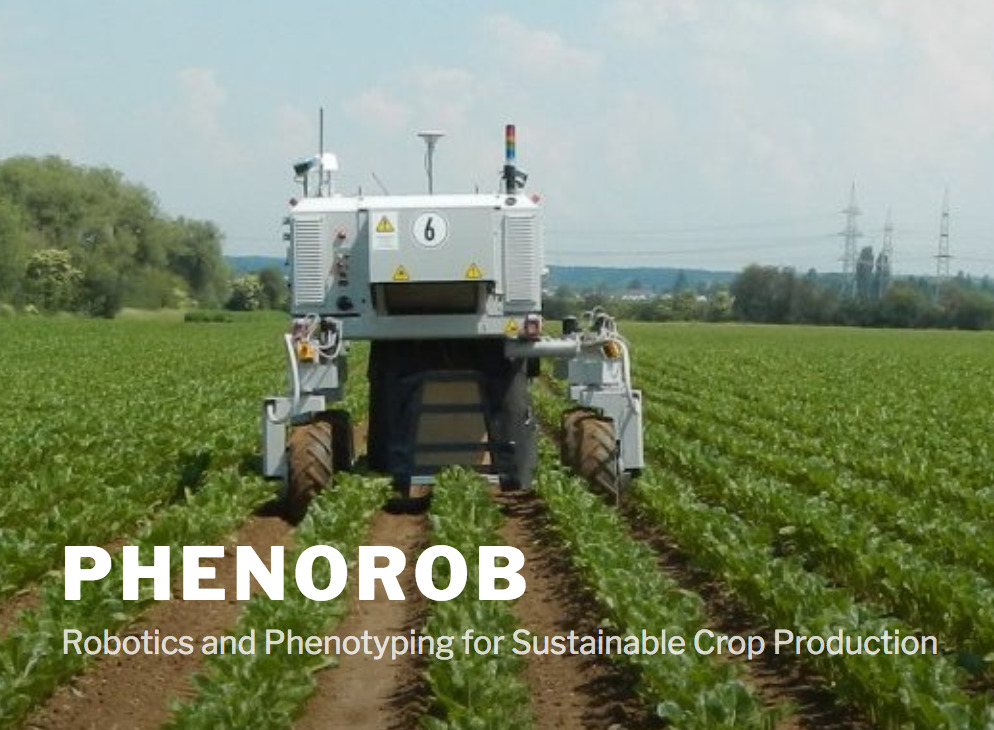
PhenoRob – Robotics and Phenotyping for Sustainable Crop Production (DFG Cluster of Excellence EXC-2070)
One of the greatest challenges for humanity is to produce sufficient food, feed, fiber, and fuel for an ever-growing world population while simultaneously reducing the environmental footprint of agricultural production. x arable land is limited, and the input of agro-chemicals needs to be reduced to curb environmental pollution and halt the decline in biodiversity. Climate change poses additional constraints on crop farming. Achieving sustainable crop production with limited resources is, thus, a task of immense proportions.
Our main hypothesis is that a major shift toward sustainable crop production can be achieved via two approaches: (1) multi-scale monitoring of plants and their environment using autonomous robots with automated and individualized intervention and big data analytics combined with machine learning to improve our understanding of the relation between input and output parameters of crop production, and (2) assessing, modeling, and optimizing the implications of the developed technical innovations in a systemic manner.
To realize our vision, we will take a technology-driven approach to address the challenging scientific objectives. We foresee novel ways of growing crops and managing fields, and aim at reducing the environmental footprint of crop production, maintaining the quality of soil and arable land, and analyzing the best routes to improve the adoption of technology.
The novel approach of PhenoRob is characterized by the integration of robotics, digitalization, and machine learning on one hand, and modern phenotyping, modeling, and crop production on the other. First, we will systematically monitor all essential aspects of crop production using sensor networks as well as ground and aerial robots. This is expected to provide detailed spatially and temporally aligned information at the level of individual plants, nutrient and disease status, soil information as well as ecosystem parameters, such as vegetation diversity. This will enable a more targeted management of inputs (genetic resources, crop protection, fertilization) for optimizing outputs (yield, growth, environmental impact). Second, we will develop novel technologies to enable real-time control of weeds and selective spraying and fertilization of individual plants in field stands. This will help reduce the environmental footprint by reducing chemical input. Third, machine learning applied to crop data will improve our understanding and modeling of plant growth and resource efficiencies and will further assist in the identification of correlations. Furthermore, we will develop integrated multi-scale models for the soil-crop-atmosphere system. These technologies and the gained knowledge will change crop production on all levels. Fourth, in addition to the impact on management decisions at the farm level, we will investigate the requirements for technology adoption as well as socioeconomic and environmental impact of the innovations resulting from upscaling.
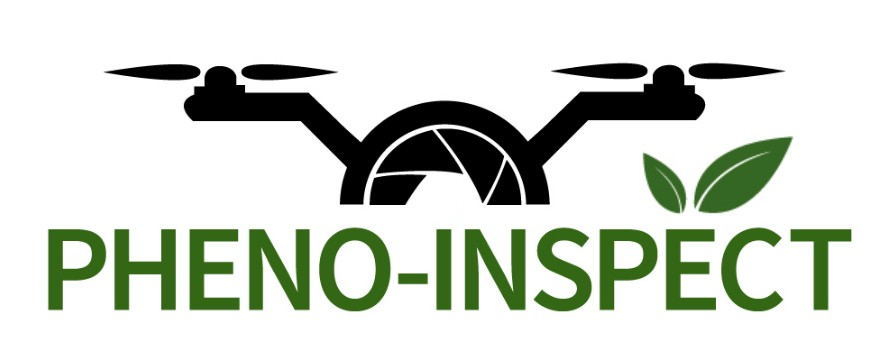
Pheno-Inspect (EFRE-funded Start-Up, TRANSFER.NRW)
Accelerating and improving breeding towards more efficient crops and varieties is key for increasing yield and improving resilience of plants. For plant breeders, it is important to observe and document phenotypic traits describing the appearance of plants in the field to evaluate the quality and success of the breeding process. With Pheno-Inspect, we aim at offering growers and farmers novel software solutions for automated high-throughput phenotyping in the fields. For sensing, we reply on small and lightweight aerial platforms, which enable a flexible, large-area and time-efficient survey of fields and plot experiments. With our software toolchain, we provide breeders and farmers with a tool to gain precise knowledge of the crop and individual plants. We automatically detect phenotypic traits of crop plants, the species of plants and weeds in the field and derive site- or plot-specific statistics. Our approach relies on state-of-the-art machine learning methods optimized for the agricultural domain to semantically interpret the captured image data and to extract the desired parameters about the plants. The learning procedures developed by us use expert knowledge inserted by the user to adapt efficiently and thus deliver the desired results quickly and effectively with regard to individual problems and local characteristics of the environment.
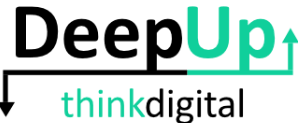
DeepUp (EXIST-funded Start-Up, EXIST Host: Dr. Lasse Klingbeil)
DeepUp – See the Unseen.
Invisible and indispensable. Fiber optics, electricity, gas and water networks are the lifelines of every industrial nation, without which modern life would not be possible. Despite this, they are still treated in the same way today as they were 50 years ago. This leads to massive damage that directly affects not only our national economy, but also each and every one of us. DeepUp is revolutionizing the way we see and understand underground infrastructure – to enable us all to live safely and sustainably in a booming infrastructure world. DeepUp started as an EXIST-funded tech startup at Bonn University.
Escarda Technologies GmbH Spinoff (BMBF, EXISTS)
Applying herbicides is the main means of weed control. The extensive use of chemical substances in agriculture can generate negative effects on our environment and biodiversity. Additionally, several weed varieties have been developing a natural resistance to the applied chemicals, hence new and more potent herbicides have to be developed, which have a higher toxicity with potentially dire consequences for the environment and organisms that come into contact with it. Escarda Technologies is a BMBF-funded EXIST project that aims at combining modern computer vision technology with lasers to develop a chemical-free weeding alternative for our fields. Escarda received funding in 2018 and has turned into a spin-off from the University of Bonn in 2019 with investment from the Berlin Industrial Group. See the Escarda Technologies GmbH website for more information.
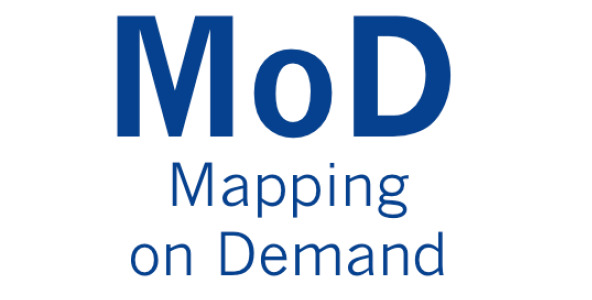
MoD – Mapping on Demand (DFG Research Unit)
Sub-project P4 ‘Incremental Mapping from Image Sequences’
Sub-project P8 ‘Exploration for UAVs’
The goal of the project is the development and testing of procedures and algorithms for the fast three-dimensional identification and mensuration of inaccessible objects on the basis of a semantically specified user inquiry. The sensor platform is a lightweight autonomously flying drone. It uses the visual information from cameras for navigation, obstacle detection, exploration and object acquisition. The methods to be develop focus on the autonomous acquisition of probabilistic models to capture spatial-temporal patterns including semantics. The ability to cope with noisy sensor data and to explicitly represent the uncertainty is a central design element. The targeted approaches can be summarized with the term Mapping on Demand. This includes all processes, techniques, and tools to acquire the sensor data, to process and to interpret it with the goal of building a semantically annotated 3D model for the user. The user obtains the model on time and it supports his decision making process. Within the second phase, the research unit focuses on techniques for onboard execution and that are of incremental nature.
Flourish (EC, H2020)
To feed a growing world population with the given amount of available farm land, we must develop new methods of sustainable farming that increase yield while reducing reliance on herbicides and pesticides. Precision agricultural techniques seek to address this challenge by monitoring key indicators of crop health and targeting treatment only to plants that need it. This is a time consuming and expensive activity and while there has been great progress on autonomous farm robots, most systems have been developed to solve only specialized tasks. This lack of flexibility poses a high risk of no return on investment for farmers. The goal of the Flourish project is to bridge the gap between the current and desired capabilities of agricultural robots by developing an adaptable robotic solution for precision farming. By combining the aerial survey capabilities of a small autonomous multi-copter Unmanned Aerial Vehicle (UAV) with a multi-purpose agricultural Unmanned Ground Vehicle, the system will be able to survey a field from the air, perform targeted intervention on the ground, and provide detailed information for decision support, all with minimal user intervention. The system can be adapted to a wide range of crops by choosing different sensors and ground treatment packages. This development requires improvements in technological abilities for safe accurate navigation within farms, coordinated multi-robot mission planning that enables large field survey even with short UAV flight times, multispectral three-dimensional mapping with high temporal and spatial resolution, ground intervention tools and techniques, data analysis tools for crop monitoring and weed detection, and user interface design to support agricultural decision making. As these aspects are addressed in Flourish, the project will unlock new prospects for commercial agricultural robotics in the near future.
RobDREAM (EC, H2020)
Sleep! For hominids and most other mammals sleep means more than regeneration. Sleep positively affects working memory, which in turn improves higher-level cognitive functions such as decision making and reasoning. This is the inspiration of RobDREAM! What if robots could also improve their capabilities in their inactive phases – by processing experiences made during the working day and by exploring – or “dreaming” of – possible future situations and how to solve them best? In RobDREAM we will improve industrial mobile manipulators’ perception, navigation and manipulation and grasping capabilities by automatic optimization of parameters, strategies and selection of tools within a portfolio of key algorithms for perception, navigation and manipulation and grasping, by means of learning and simulation, and through use case driven evaluation. As a result, mobile manipulation systems will adapt more quickly to new tasks, jobs, parts, areas of operation and various other constraints. From a scientific perspective the RobDREAM robots will feature increased adaptability, dependability, flexibility, configurability, decisional autonomy, as well as improved abilities in perception, interaction manipulation and motion. The technology readiness level (TRL) of the related key technologies will be increased by means of frequent and iterative real-world testing, validation and improvement phases from the very beginning of the project.From an economic perspective the Quality of Service and the Overall Equipment Efficiency will increase, while at the same time the Total Cost of Ownership for setup, programming and parameter tuning will decrease. These advantages will support the competitiveness of Europe’s manufacturing sector, in particular in SME-like settings with higher product variety and smaller lot-sizes. They also support the head start of technology providers adopting RobDREAM’s technologies to conquer market shares in industrial and professional service robotics.
Rovina – Robots for Exploration, Digital Preservation and Visualization of Archeological Sites (EC, FP7, 2013-2016)
Mapping and digitizing archeological sites is an important task to preserve cultural heritage and to make it accessible to the public. Current systems for digitizing sites typically build upon static 3D laser scanning technology that is brought into archeological sites by humans. This is acceptable in general, but prevents the digitization of sites that are inaccessible by humans. In the field of robotics, however, there has recently been a tremendous progress in the development of autonomous robots that can access hazardous areas. ROVINA aims at extending this line of research with respect to reliability, accuracy and autonomy to enable the novel application scenario of autonomously mapping of areas of high archeological value that are hardly accessible. ROVINA will develop methods for building accurate, textured 3D models of large sites including annotations and semantic information. To construct the detailed model, it will combine innovative techniques to interpret vision and depth data. ROVINA will furthermore develop advanced techniques for the safe navigation in the cultural heritage site. To actively control the robot, ROVINA will provide interfaces with different levels of robot autonomy. Already during the exploration mission, we will visualize relevant environmental aspects to the end-users so that they can appropriately interact and provide direct feedback.
EUROPA2 (EC, FP7, 2013-2016)
The goal of the EUROPA2 project, which builds on top of the results of the successfully completed FP7 project EUROPA (see below), is to bridge this gap and to develop the foundations for robots designed to autonomously navigate in urban environments outdoors as well as in shopping malls and shops, for example, to provide various services to humans. Based on the combination of publicly available maps and the data gathered with the robot’s sensors, it will acquire, maintain, and revise a detailed model of the environment including semantic information, detect and track moving objects in the environment, adapt its navigation behavior according to the current situation and anticipate interactions with users during navigation. A central aspect in the project is life-long operation and reduced deployment efforts by avoiding to build maps with the robot before it can operate. EUROPA2 is targeted at developing novel technologies that will open new perspectives for commercial applications of service robots in the future.
Previous Projects by C. Stachniss as a PI conducted at the University of Freiburg
- STAMINA – Sustainable and Reliable Robotics for Part Handling in Manufacturing Automation
- AdvancedEDC – Advanced Intracortical Neural Probes with Electronic Depth Control (within EXC-BrainLinks-BrainTools)
- SFB/TR-8 – Spatial Congnition
- TAPAS – Robotics-enabled Logistics and Assistive Services for the Transformable Factory of the Future
- First-MM – Flexible Skill Acquisition and Intuitive Robot Tasking for Mobile Manipulation in the Real World
- EUROPA – European Robotic Pedestrian Assistant
Previous Projects by W. Förster and B. Waske
- Modelling the spatio-temporal variability of crop and cropping system processes under heterogenous field conditions
- Structural-ecolgical mapping of river courses, using TerraSAR-X and RaipdEye data
- Remote sensing based retrieval of biomethane potential (BMP) of crops, with regard to the EnMAP mission
- Monitoring Farmland Abandonment by multitemporal and multisensor remote sensing imagery (MOFA) (transfered to FU Berlin 01.01.2014)
- Semi-automatic Generation of Highly Detailed Textured Building Models
- eTRIMS- E-Training for Interpreting Images of Man-Made Scenes (2006-2009)
- Ontological Scales
- A Control Point Model Database for Automatic Exterior Orientation (together with Survey Department NRW) (1994-2000)
- Semantic Modeling and Extraction of Spatial Objects from Images and Maps (SM) (1993-1999)
- Image Processing for Automatic Carthographic Tools (IMPACT)
- Photogrammetric investigations with MOMS-02 imagery
- Calibration of a fringe projection (structured light) sensor system (in german)
- Automatic Geometric and Semantic Reconstruction of Buildings from Images by Extraction of 3D-Corners and their 3D-Aggregation
- Photogrammetric Eye
- A Generic Adjustment Module
- Photogrammetric Observation and Reconstruction of the Development of a Fluvial Sediment Surface (in german)
- Semi-automatic Building Acquisition
-
Photogrammetric Acquisition and Representation of 3D Objects for Digital Landscape Models (1997-1999)
- A Photogrammetric Scanner for the Analytical Plotter P3